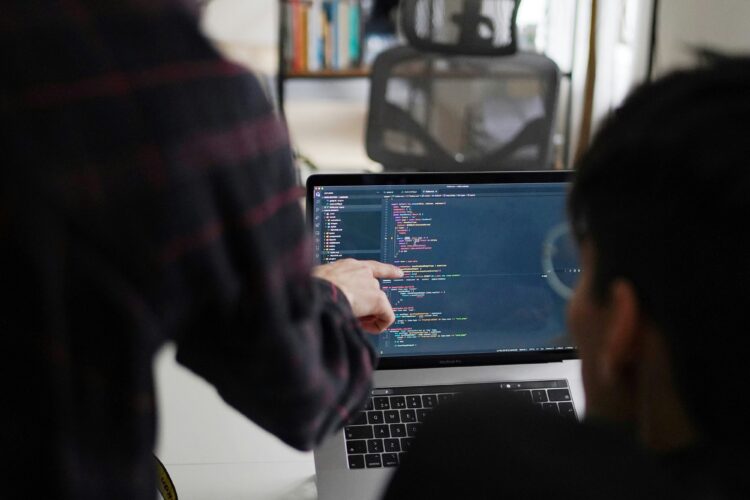
Personalized marketing is no longer a trend—it’s a necessity. Today’s consumers expect brands to recognize their preferences and deliver tailored content across every touchpoint. Marketers are turning to data analytics as a strategic tool to guide personalization efforts to meet this demand. Businesses can craft marketing messages that feel relevant, timely, and individualized by analyzing customer behavior, purchase history, and engagement patterns.
This blog shows how data analytics supports every stage of personalized marketing—from collecting consumer insights to predicting future actions—so your campaigns connect more effectively and drive measurable results.
Key Takeaways
- Data analytics is the foundation of personalized marketing, enabling brands to understand and anticipate individual customer needs and preferences.
- Integrating data from multiple sources—including CRM systems, web behavior, and mobile apps—creates a unified customer view for more accurate targeting.
- Predictive techniques and machine learning help forecast consumer behavior, automate personalization, and improve campaign performance.
- Real-time analytics empowers agile decision-making, allowing marketers to optimize campaigns based on current user interactions.
- Ethical data practices and regulatory compliance (such as GDPR and CCPA) are critical for maintaining consumer trust and protecting personal information.
- Emerging technologies like AI and visualization tools enhance personalized marketing efforts’ precision and scalability.
The Role of Data Analytics in Personalized Marketing
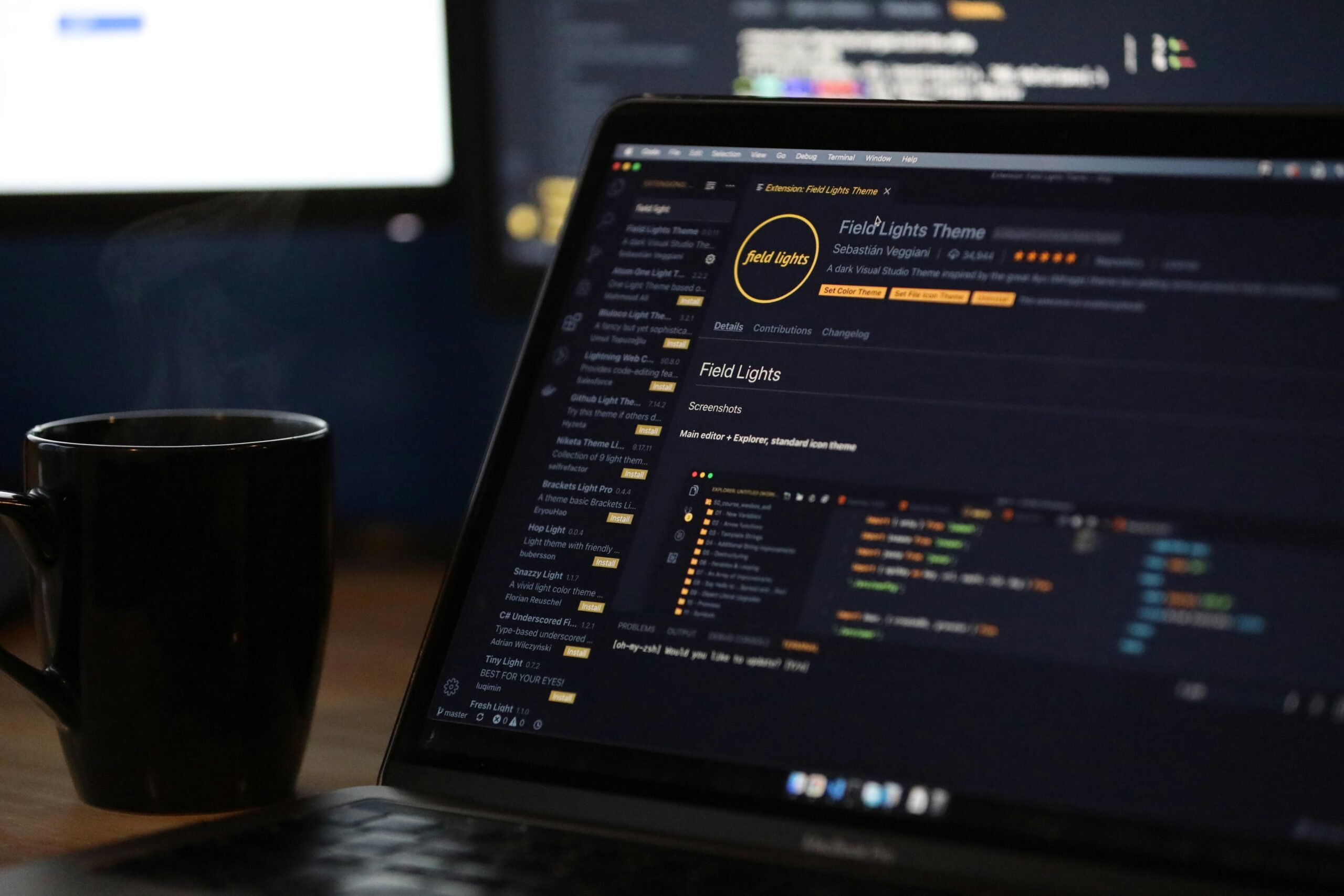
To deliver truly personalized marketing, businesses need more than just intuition—they need data. Data analytics provides the foundation for understanding customer behavior and turning that knowledge into targeted, effective marketing strategies. It enables marketers to analyze how people interact with their brand, identify trends, and align content to individual preferences. This section explores how data analytics powers the shift from generic outreach to dynamic, data-informed personalization.
Building Personalization Through Consumer Data
Personalization starts with data collection. Marketers gather information from multiple sources, such as website activity, mobile app usage, purchase history, and email engagement, to form detailed customer profiles. This data reveals what a customer is interested in and when and how they’re most likely to engage. By mapping out these patterns, marketers can segment their audience and deliver messaging tailored to specific interests, behaviors, and timing.
Extracting Trends and Insights from Behavioral Data
Analyzing consumer behavior over time helps identify emerging trends and recurring patterns. For example, if customers frequently abandon carts at a certain point in checkout, analytics can reveal that friction point. Likewise, identifying high-performing product categories or seasonal behaviors enables proactive marketing. By interpreting these insights, marketers can fine-tune messaging and offers that resonate at the right moment.
Improving Decision-Making with Data-Driven Strategy
Instead of guessing what customers want, marketers use analytics to support strategic decision-making. Data helps determine which channels are performing best, what content is driving engagement, and which segments respond to specific types of campaigns. This clarity reduces wasted spend, increases ROI, and ensures that marketing decisions are backed by evidence—not assumptions.
Integrating and Interpreting Multi-Source Data
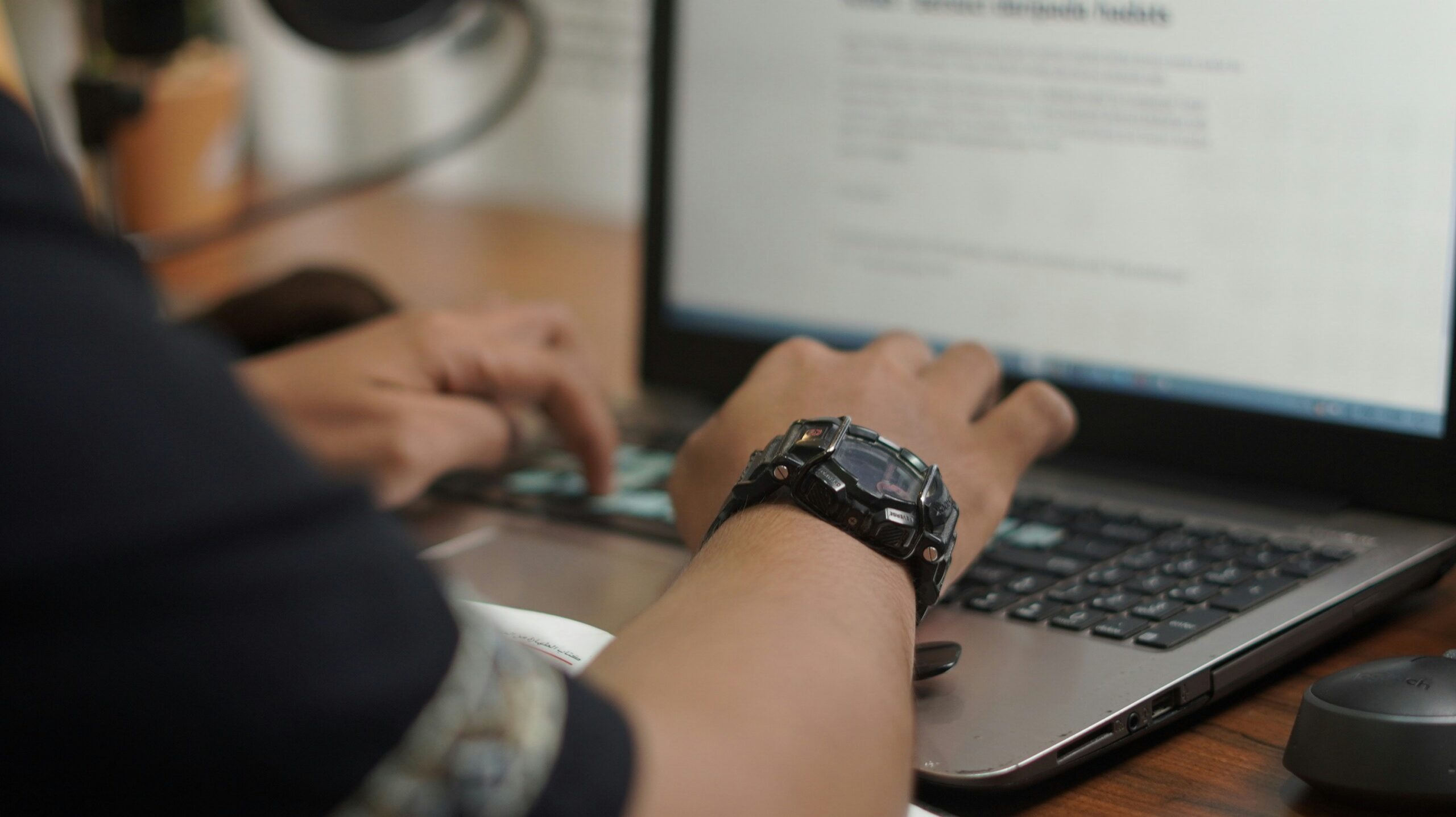
A personalized marketing strategy is only as strong as the data behind it. But collecting data isn’t enough—it must be integrated, interpreted, and activated across all platforms. Siloed information from separate tools can lead to fragmented campaigns and missed opportunities. When marketers unify data from various sources into a single customer view, they can create messaging that’s not just personalized but consistent, timely, and scalable.
Combining CRM, Email, and App Data for a Unified View
Customers interact with brands across multiple touchpoints—emails, websites, apps, loyalty programs, etc. Marketers gain a holistic view of the customer journey by integrating data from these sources. For instance, if a customer frequently opens email promotions but purchases through an app, combining that data helps tailor offers to their preferred channel and behavior. This cross-platform integration ensures messaging is aligned with how each user prefers to engage.
Eliminating Data Silos with Cloud-Based Solutions
Marketers struggle to connect the dots when data lives in isolated systems—like separate CRM tools, analytics platforms, or sales databases. Cloud-based marketing platforms allow for seamless data consolidation, enabling real-time access to customer insights. These tools also support data hygiene practices, reducing redundancy and ensuring accuracy. With a centralized system, marketers can automate recommendations, sync updates across teams, and respond to consumer behavior faster.
Transforming Raw Data into Strategic Insights
Raw data in its original form—clicks, log files, feedback forms—isn’t immediately useful. It must be cleaned, structured, and analyzed to reveal meaning. This transformation process often involves techniques like natural language processing (NLP) to analyze reviews or social comments, and data modeling to highlight patterns and predict outcomes. Once refined, these insights drive smarter segmentation, sharper targeting, and more relevant content creation.
Predictive Techniques That Power Personalization
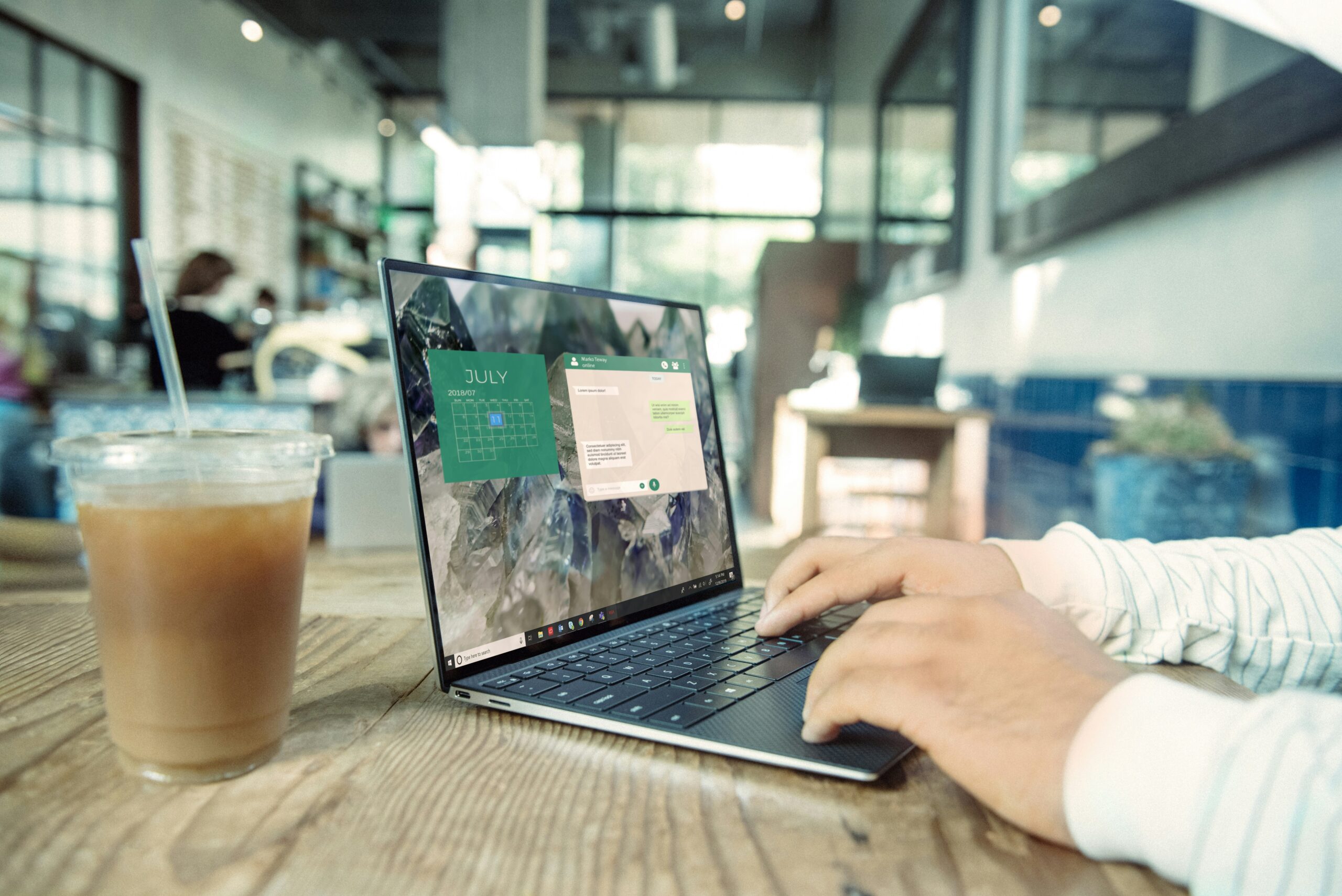
While descriptive analytics tell marketers what has already happened, predictive techniques go a step further—they anticipate what customers are likely to do next. This forward-looking approach is essential for delivering the right message at the right time. Using statistical models and machine learning, businesses can forecast customer behavior, automate decision-making, and scale personalized experiences that align with individual interests and intent.
Using Statistical Models to Forecast Customer Behavior
Statistical techniques like regression analysis, decision trees, and clustering help marketers identify patterns and relationships in historical data. For example, regression can predict how price changes affect sales, while clustering groups customers based on shared behaviors or demographics. These models guide campaign timing, product recommendations, and channel selection, allowing marketers to tailor their efforts based on predicted outcomes rather than past trends alone.
Applying Machine Learning for Dynamic Personalization
Machine learning algorithms continuously improve as they process more data, making them ideal for personalization at scale. They can analyze large, complex datasets, such as browsing behavior, transaction history, and content interactions, to predict what a customer might want next. Whether it’s recommending a product, selecting a subject line, or personalizing a landing page, machine learning ensures marketing adapts in real time to customer needs and behaviors.
Refining Targeting with A/B Testing and Algorithmic Learning
Algorithms don’t just automate personalization—they refine it. Marketers can use A/B testing and multivariate testing to experiment with different messaging elements (like images, CTAs, or offers) and see what resonates. These test results feed back into the algorithm, sharpening targeting precision and improving performance over time. The result is a self-improving system that drives higher engagement, lower acquisition costs, and stronger ROI.
Real-Time Analytics and Continuous Optimization
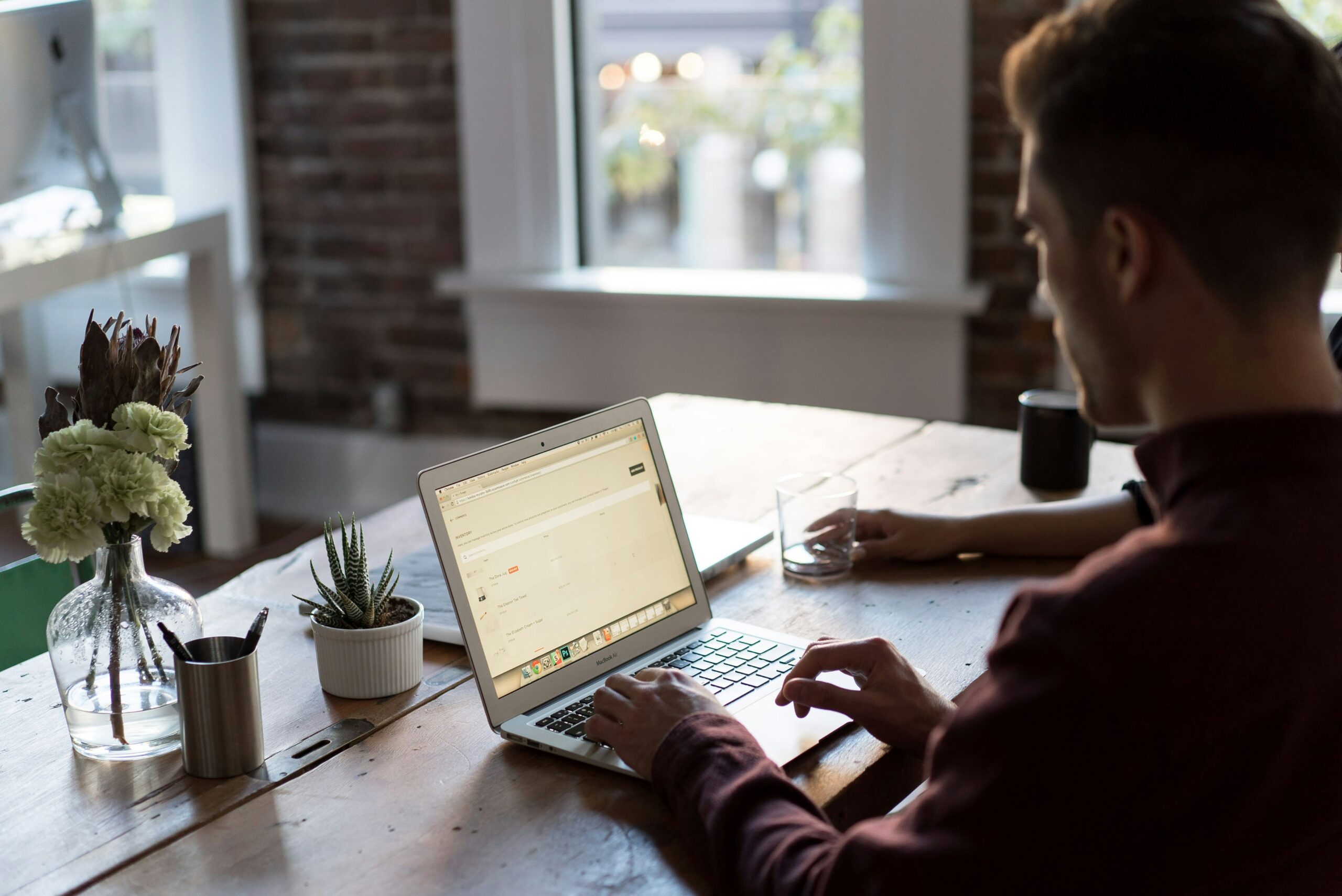
Personalization isn’t a one-and-done effort—it requires constant adjustment. Real-time analytics give marketers the power to monitor campaign performance as it happens, allowing them to refine messaging, offers, and targeting on the fly. By leveraging live data from web activity, emails, social platforms, and mobile apps, businesses can react instantly to consumer behavior, optimizing campaigns for maximum impact and efficiency.
Monitoring Key Performance Indicators (KPIs)
Real-time dashboards let marketers track KPIs like click-through rates, conversions, time on site, and engagement levels. These metrics provide immediate feedback on what’s working and what needs to change. For example, a sudden drop in open rates could prompt a subject line test, while high bounce rates might lead to revisiting the landing page design. With live visibility into campaign performance, marketers can avoid costly delays in making adjustments.
Adjusting Campaign Elements in the Moment
Analytics tools now make it possible to pivot mid-campaign based on user behavior. Marketers can reallocate ad spend or update creatives in real time if data shows a certain product category is trending. Automated personalization systems can even trigger dynamic content based on current user interactions, like offering a time-sensitive discount if a customer hesitates at checkout. These responsive actions help keep marketing relevant and timely.
Experimenting and Iterating for Long-Term Improvement
Continuous optimization isn’t just about reacting—it’s about testing and learning. Through A/B testing, marketers can evaluate different subject lines, visuals, or ad placements. Multivariate testing allows for deeper experimentation with multiple elements at once. The insights from these tests inform future strategies, turning campaign performance into a feedback loop that fuels more innovative marketing with every iteration.
Ethical Considerations and Data Privacy
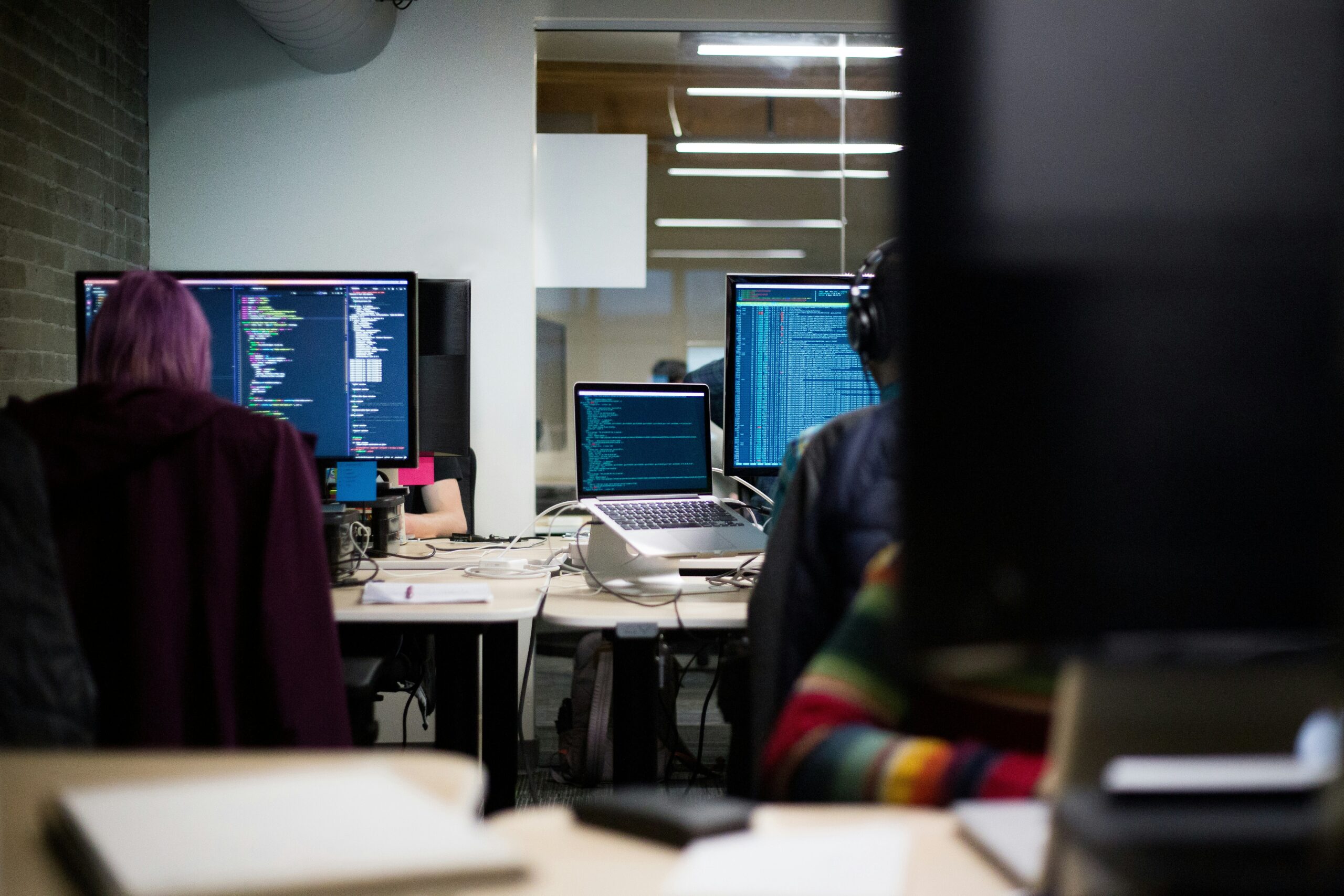
As businesses collect more customer data to power personalization, they also take on greater responsibility. Trust is now a currency in digital marketing, and mishandling consumer data can lead to reputational damage, legal penalties, and lost customers. Ethical data practices and compliance with privacy regulations are not optional; they are essential to personalized marketing strategies’ long-term success and sustainability.
Complying With Data Privacy Regulations (GDPR, CCPA, etc.)
Laws like the General Data Protection Regulation (GDPR) in the EU and the California Consumer Privacy Act (CCPA) in the U.S. establish clear rules for how companies collect, store, and use personal data. These regulations require transparency, user consent, and options for consumers to access or delete their data. For marketers, compliance means implementing consent management tools, limiting data retention, and clearly communicating how data will be used.
Implementing Privacy-First Personalization Practices
Balancing personalization with privacy requires strategic thinking. Privacy-first practices—such as data minimization, pseudonymization, and anonymized data modeling—allow marketers to extract insights while protecting individual identities. Additionally, brands can offer personalization based on behavioral segments instead of directly identifying users, ensuring relevance without overstepping privacy boundaries.
Building Consumer Trust Through Transparency and Security
Beyond compliance, marketers must cultivate trust through transparency. Clear privacy policies, accessible preference centers, and secure data handling protocols show consumers that their data is respected and protected. Techniques like encryption, regular audits, and limited internal data access reduce the risk of breaches. When customers feel safe sharing information, they are more likely to engage, convert, and remain loyal.
Emerging Trends in Personalized Marketing Analytics
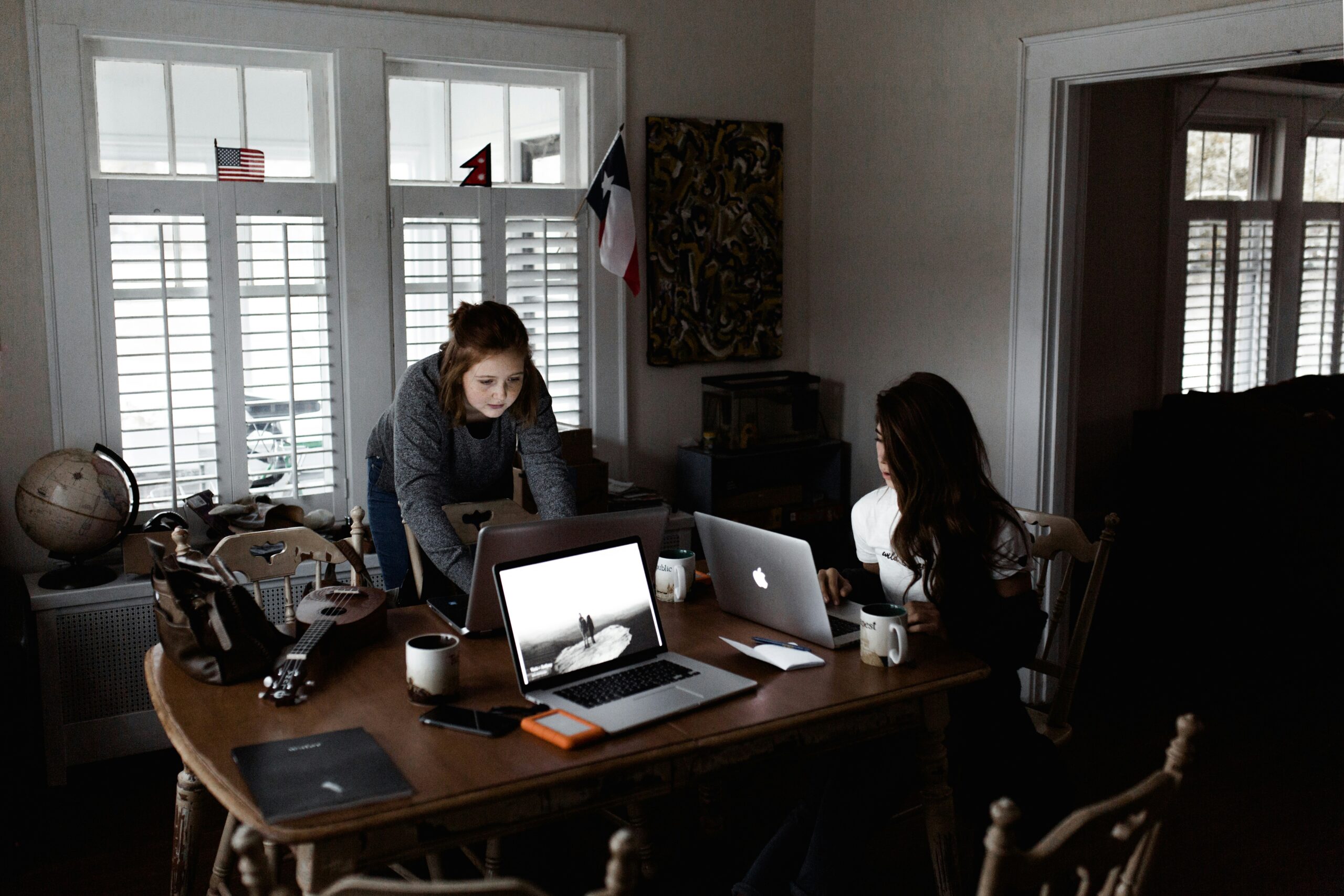
As marketing technology evolves, so do the tools and techniques used to deliver personalized experiences. New developments in AI, analytics platforms, and data governance are shaping the future of personalization, enabling marketers to move faster, reach deeper, and operate more responsibly. Staying ahead of these trends allows businesses to refine their strategies and maintain a competitive edge.
Leveraging AI for Intelligent Personalization
Artificial intelligence (AI) is transforming how marketers analyze and apply customer data. Through machine learning, natural language processing, and real-time behavior tracking, AI can uncover patterns that would be impossible to detect manually. Marketers can use AI to recommend products, automate personalized content, and predict optimal timing for outreach—often in milliseconds. This leads to greater relevance, higher engagement, and more efficient campaign execution.
Adopting Advanced Visualization and Reporting Tools
Today’s analytics platforms offer more than static reports—they provide interactive dashboards, real-time visualizations, and automated insight generation. These tools allow marketing teams to intuitively explore large datasets, identify performance gaps quickly, and confidently make data-informed decisions. Augmented analytics, in particular, empowers non-technical users to uncover insights without relying heavily on data science teams.
Preparing for Future Shifts in Data Regulation and Consumer Expectations
With growing awareness of digital privacy, consumers are becoming more selective about the brands they trust. At the same time, regulatory landscapes are tightening, and marketers must adapt proactively. Businesses should invest in flexible data infrastructures, strengthen consent mechanisms, and stay informed about emerging privacy laws. This future-proofing approach ensures that personalization strategies remain effective and compliant in a changing environment.
Conclusion
Personalized marketing is no longer driven by assumptions—data power it. Businesses can move beyond generic messaging to deliver content that truly resonates with each customer through the strategic use of data analytics. From integrating cross-channel data to applying predictive models and machine learning, analytics provides the insights needed to optimize every stage of the customer journey.
But effective personalization isn’t just about technology—it’s also about trust. Ethical data practices, transparent communication, and compliance with privacy regulations are critical to sustaining customer relationships and long-term success. As emerging tools and trends continue to shape the marketing landscape, organizations that invest in innovative, secure, and scalable data strategies will be best positioned to lead.
Frequently Asked Questions
How does data analytics enhance personalized marketing?
Data analytics helps businesses understand individual consumer behaviors, preferences, and patterns. This enables marketers to craft targeted messages, deliver content quickly, and improve customer experiences across multiple channels.
What types of data are most useful for marketing personalization?
The most valuable data types include browsing history, purchase behavior, email engagement, mobile app activity, social media interactions, and CRM records. Combining these data sources creates a comprehensive view of the customer.
How can real-time analytics improve campaign performance?
Real-time analytics allows marketers to monitor campaign performance as it happens, enabling immediate adjustments to content, targeting, and timing. This leads to more responsive and effective campaigns that adapt to customer behavior on the fly.
What are the key ethical concerns in using customer data?
Major concerns include consumer privacy, data security, and informed consent. Marketers must follow privacy regulations (like GDPR and CCPA), be transparent about data usage, and implement strong security protocols to protect personal information.
How does AI contribute to personalization efforts?
AI enables scalable personalization by analyzing large datasets, predicting customer behavior, and automating recommendations in real time. It helps marketers deliver more relevant content with greater speed, accuracy, and efficiency.
Comments