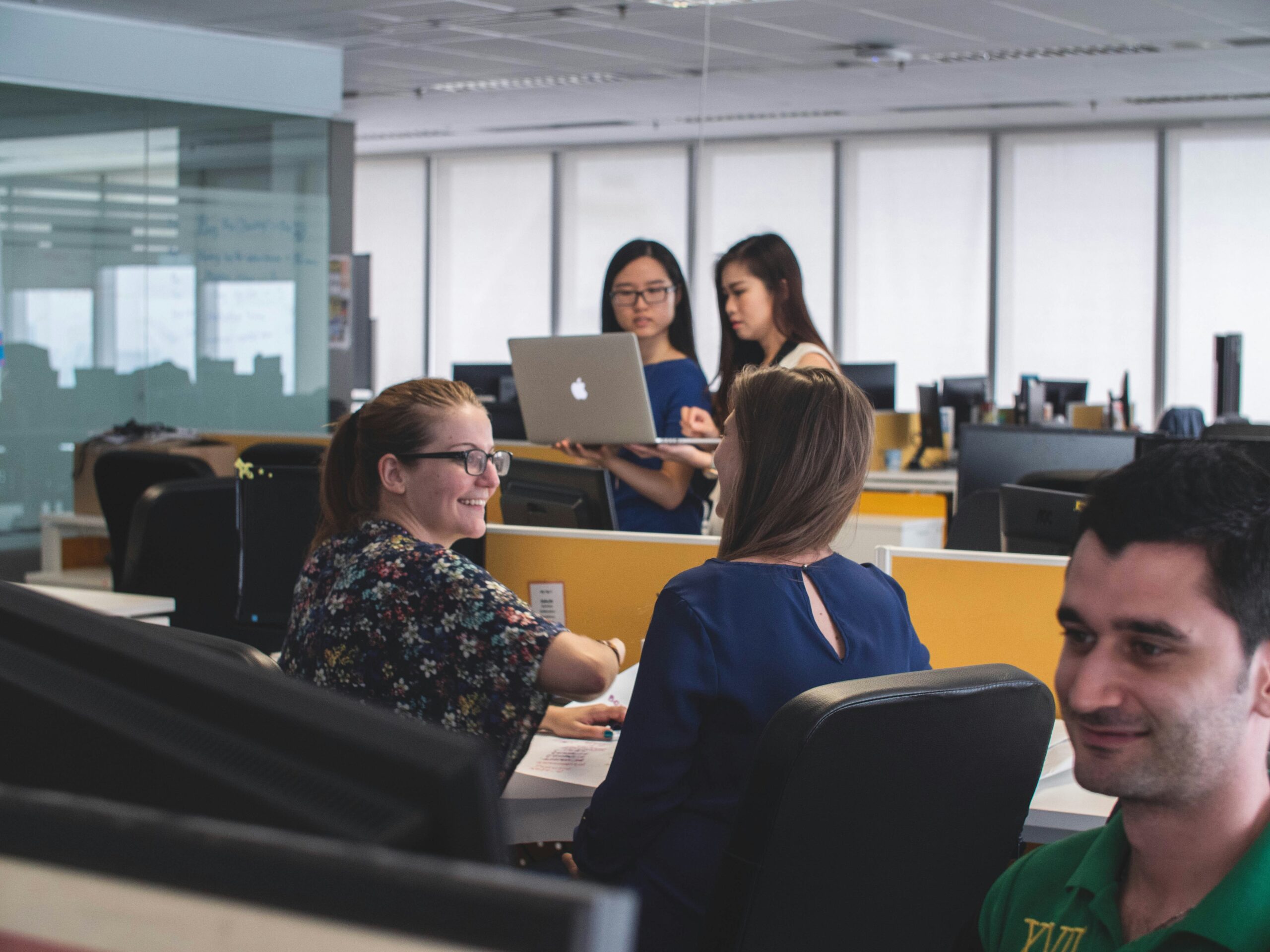
Maximizing marketing success can be challenging in a competitive landscape. With machine learning strategies, businesses can transform their marketing efforts. This article will explore how machine learning enhances personalized campaigns and improves content creation and distribution. Readers will learn to utilize predictive analytics for targeted outreach, ultimately boosting customer engagement. If struggling to keep up with consumer demands and optimize marketing strategies, this content will provide actionable insights to streamline efforts and drive results.
Key Takeaways
- Machine learning enhances marketing by analyzing customer behavior for personalized strategies
- Predictive analytics help identify leads, increasing conversion rates and optimizing marketing efforts
- Automating content distribution boosts visibility and supports search engine optimization strategies
- Continuous learning in machine learning improves marketing effectiveness and user experiences
How Machine Learning Enhances Marketing Opportunities
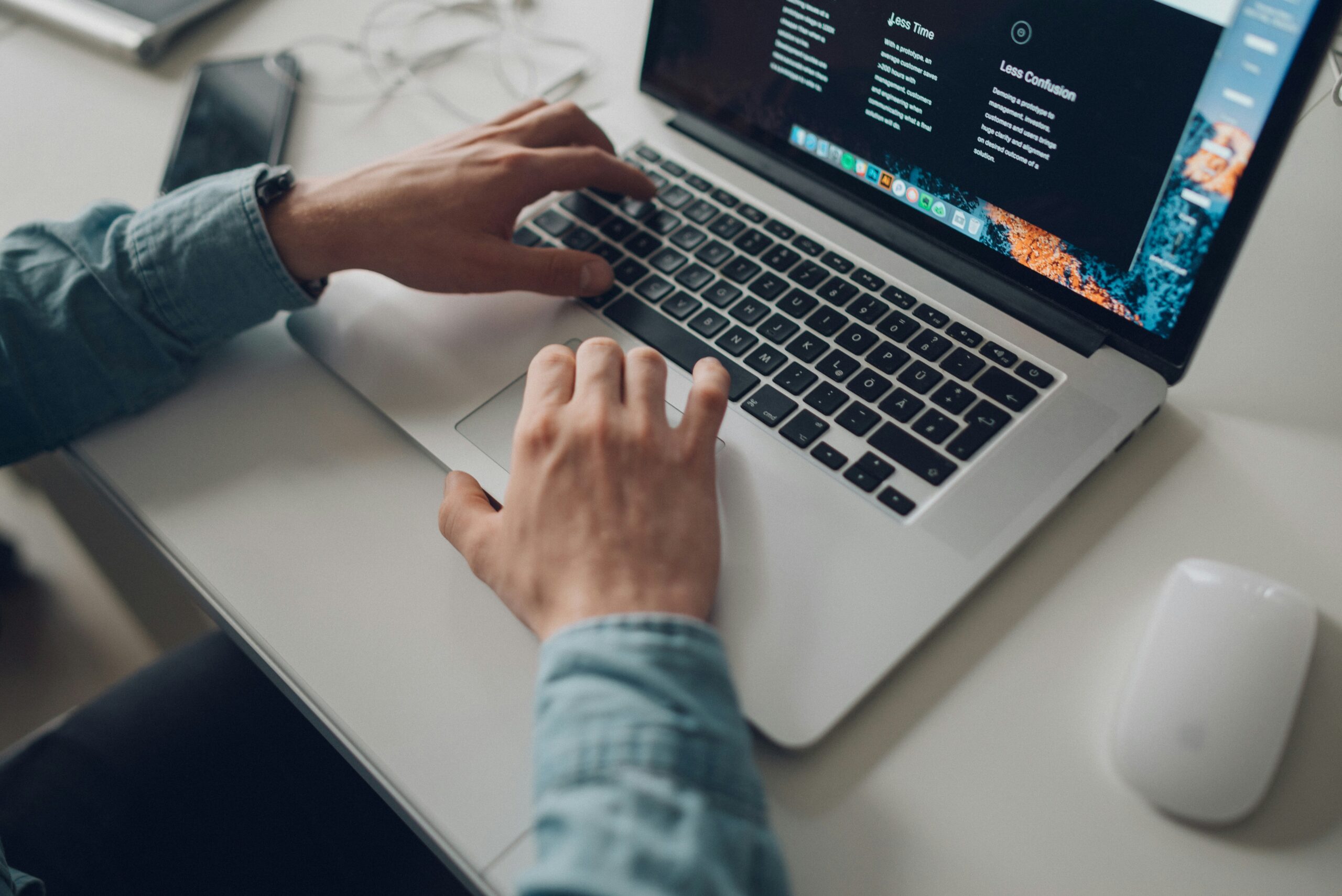
Machine learning is transforming how marketers uncover opportunities, improve customer targeting, and drive campaign effectiveness. By analyzing vast amounts of behavioral data, machine learning algorithms help brands make smarter decisions, faster.
Uncovering Consumer Insights Through Behavioral Analysis
Machine learning enables marketers to perform deep analysis of customer behavior, revealing patterns that traditional analytics might overlook. These insights help identify high-performing channels, optimize campaign timing, and personalize outreach across segments. This data-driven approach supports more accurate forecasting and refined audience targeting.
Enhancing Personalization Beyond Onboarding
Rather than focusing solely on onboarding, machine learning powers end-to-end personalization throughout the customer journey. By tracking how users interact with content, products, or services, algorithms can suggest tailored messages, offers, or experiences that improve user satisfaction and increase conversion rates.
Optimizing Product Offerings and Promotional Strategies
Brands can use machine learning to dynamically adjust product recommendations and promotional strategies based on real-time feedback and historical performance. This ensures campaigns remain relevant and aligned with evolving customer needs. The result is higher engagement, improved campaign ROI, and stronger customer loyalty.
Utilizing Predictive Analytics for Targeted Campaigns
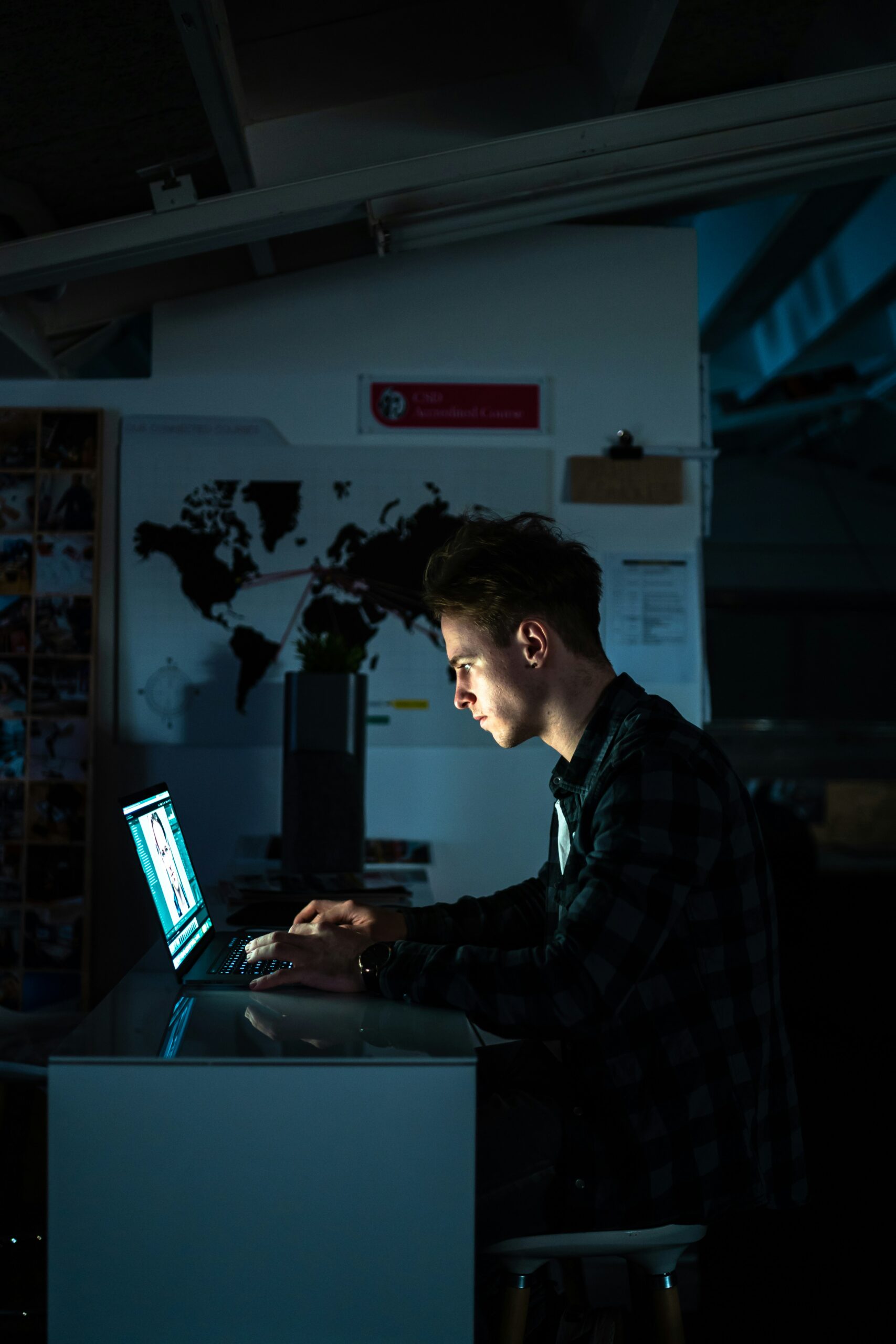
Predictive analytics, powered by machine learning, allows marketers to make informed, data-backed decisions that drive performance. These models can forecast future outcomes and optimize marketing efforts by analyzing historical behavior.
Identifying High-Value Leads Before They Convert
Machine learning algorithms process large sets of past customer data to predict which prospects are most likely to convert. This empowers marketing teams to focus their efforts on high-intent leads, improving resource efficiency and boosting conversion rates. Targeted outreach becomes more strategic, reducing wasted ad spend and increasing ROI.
Enhancing Campaign Precision With Quality Data
Data quality is a key factor in the accuracy of predictive analytics. Clean, enriched, and well-organized datasets allow machine learning models to deliver precise insights into customer behavior and preferences. These insights help marketers refine their messaging, segment audiences more effectively, and adapt campaign strategies in real time.
Driving Engagement Through Smart Social Strategies
With predictive analytics, brands can fine-tune their social media efforts by identifying what type of content resonates with different segments. From optimal posting times to message tone, ML-driven insights help increase social engagement and foster brand loyalty. The result is more meaningful interactions and a stronger digital presence.
Implementing Personalization Strategies With Machine Learning
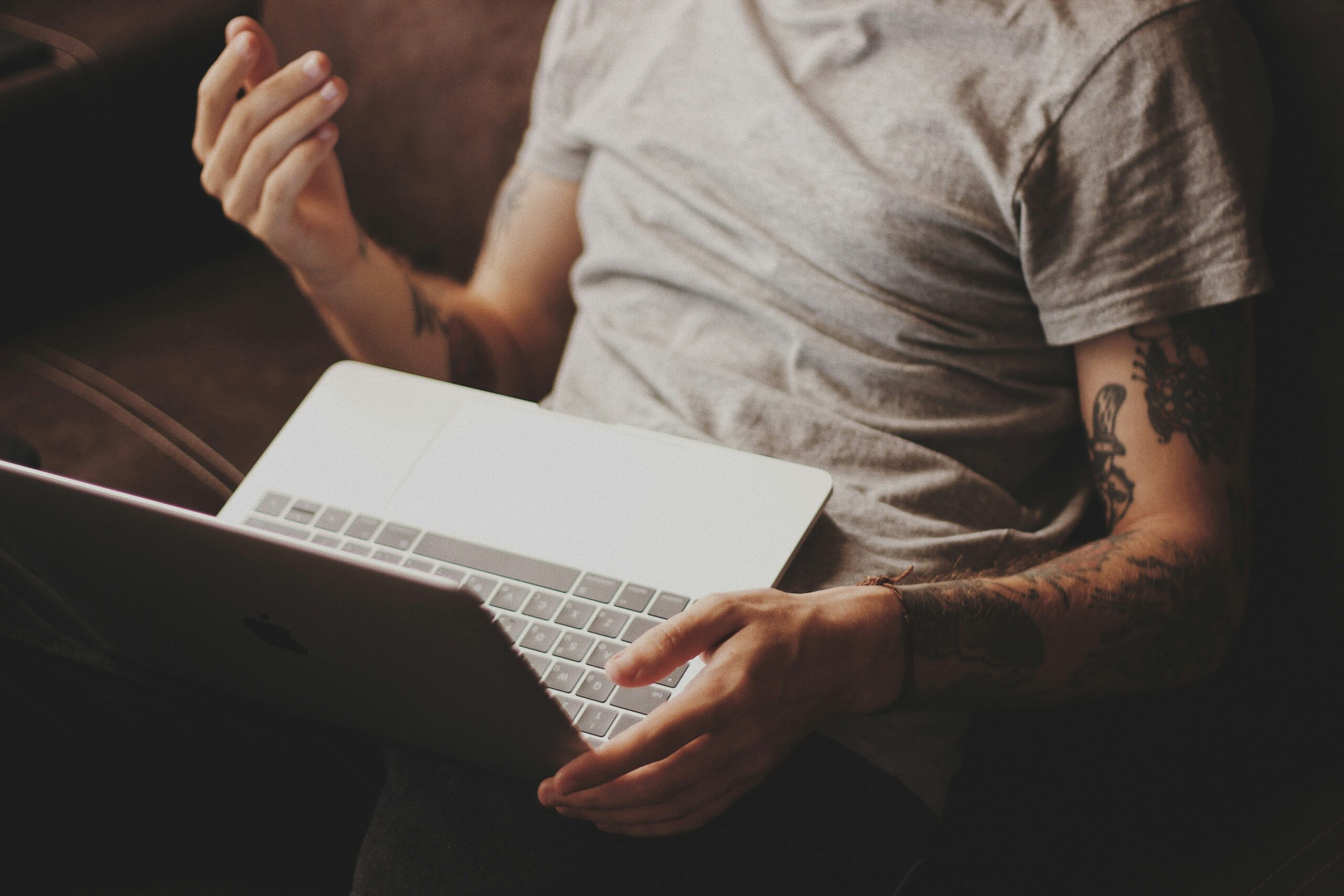
Machine learning enables highly personalized marketing experiences by turning customer data into actionable insights. These strategies help brands move beyond generic messaging and deliver relevant, timely, and personal communications.
Delivering Tailored Content at Every Touchpoint
By analyzing customer interactions across channels—such as website visits, email clicks, and purchase history—machine learning allows marketers to tailor content for each user. This results in hyper-relevant messaging that reflects individual preferences, behaviors, and stage in the customer journey.
Predicting Customer Needs Before They Arise
Machine learning models excel at identifying patterns that indicate future behavior. Marketers can use these predictive insights to anticipate customer needs, recommend products, or trigger timely communications—such as follow-up emails, reminders, or dynamic website content. This proactive approach enhances satisfaction and supports long-term loyalty.
Building Stronger Customer Relationships Through Relevance
When personalization is executed well, it goes beyond transactions and builds emotional connections. Machine learning supports this by continuously learning and adapting based on customer feedback and interaction data. The result is a marketing strategy that feels intuitive, relevant, and trustworthy—leading to deeper engagement and increased customer retention.
Streamlining Content Creation and Distribution Through Automation

Machine learning plays a key role in automating content workflows, allowing marketers to create, optimize, and distribute content more efficiently. This automation reduces manual effort while ensuring that messaging aligns with audience preferences and behavior.
Creating Smarter Content With Behavioral Insights
Machine learning analyzes user data—such as browsing history, engagement metrics, and purchase patterns—to inform content creation. These insights help marketers develop blog posts, email copy, social media content, or product descriptions that speak directly to what their audience wants. The result is more relevant, engaging material tailored to user intent.
Automating Distribution Across Channels
Once content is created, machine learning algorithms determine the best times, platforms, and formats for distribution. Whether it’s identifying peak engagement windows for social posts or segmenting email lists based on interest, ML ensures that content reaches the right people at the right moment—boosting visibility and impact.
Supporting SEO and Content Performance
In addition to automation, machine learning improves SEO strategies by analyzing keyword trends, content gaps, and user search behavior. It helps marketers identify high-performing topics, structure content for discoverability, and refine optimization techniques. Over time, these enhancements lead to better search rankings, increased traffic, and stronger brand authority.
Improving Workflow Efficiency
Machine learning can also automate repetitive content tasks—such as tagging, categorization, and basic formatting—freeing up creative teams to focus on strategy and innovation. By streamlining content management processes, brands can scale their marketing efforts without compromising quality.
Overcoming Challenges in Machine Learning Integration
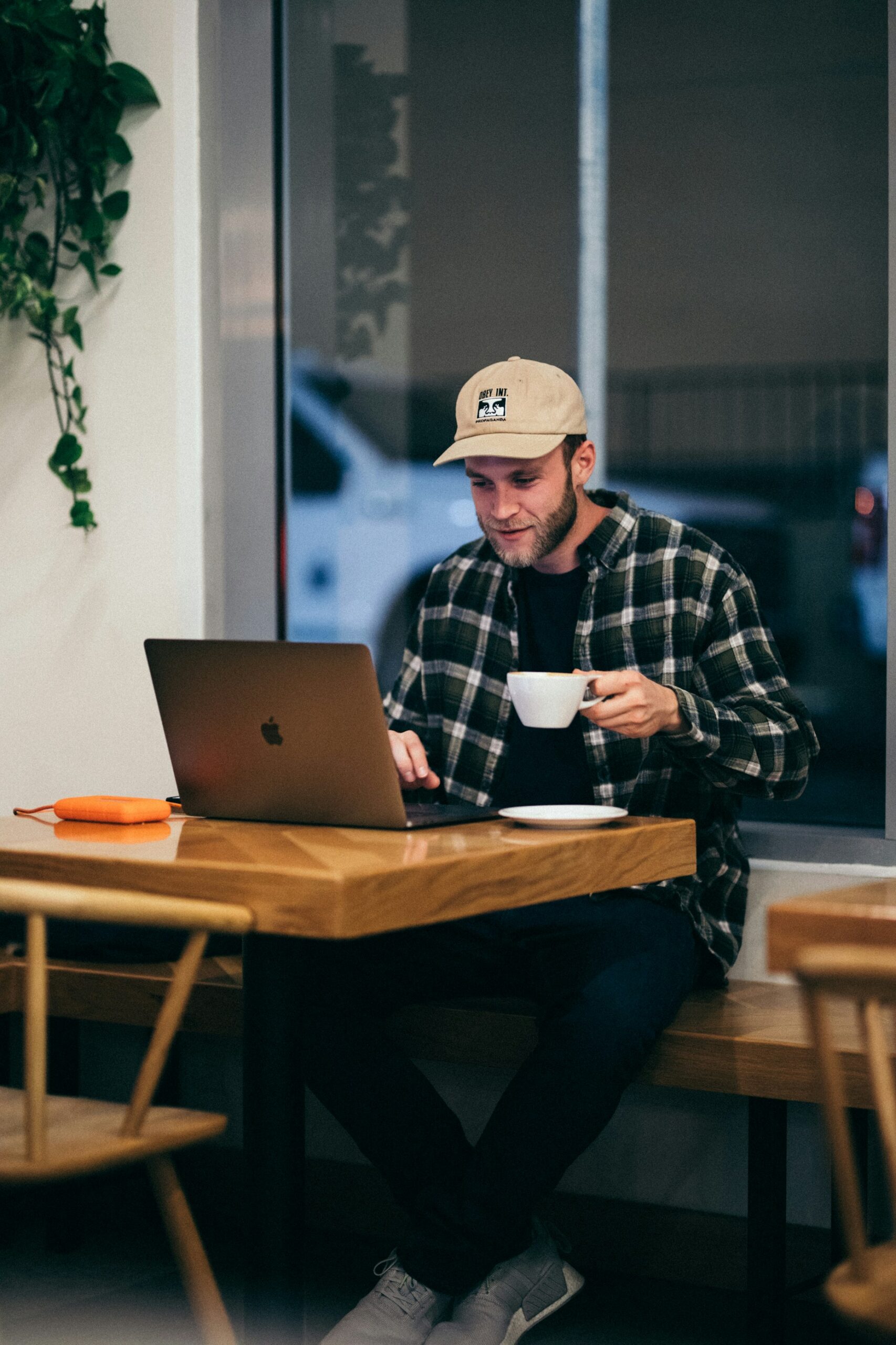
While machine learning offers powerful advantages for marketers, successful integration requires overcoming technical, operational, and strategic hurdles. Addressing these challenges is key to unlocking the full potential of intelligent marketing automation.
Dealing With Data Quality and Compatibility Issues
Machine learning models rely heavily on high-quality, structured data. Inconsistent formats, missing values, or siloed datasets can lead to poor predictions and ineffective personalization. To avoid this, marketers must invest in data cleansing, consolidation, and governance strategies to ensure reliable inputs across systems and campaigns.
Aligning Technology With Existing Workflows
Integrating machine learning into marketing workflows often means adapting legacy systems or retraining teams. Misalignment between tools and team processes can slow adoption and limit effectiveness. A successful implementation requires choosing tools that integrate seamlessly with existing platforms—such as CRMs or content management systems—and support real-time decision-making.
Empowering Teams Through Training and Upskilling
Marketers often lack the technical knowledge to fully leverage machine learning features. Investing in training programs helps teams understand how to interpret data outputs, collaborate with data scientists, and apply insights to campaigns. Upskilling also builds confidence in using automation tools strategically rather than relying on guesswork.
Scaling With Supportive Infrastructure
Although not the main focus, supporting technologies—like cloud platforms—can help process large data volumes and scale ML applications effectively. Leveraging cloud-based solutions enables faster model deployment and real-time analytics, especially for high-volume marketing campaigns.
Future Trends of Machine Learning in Marketing Automation
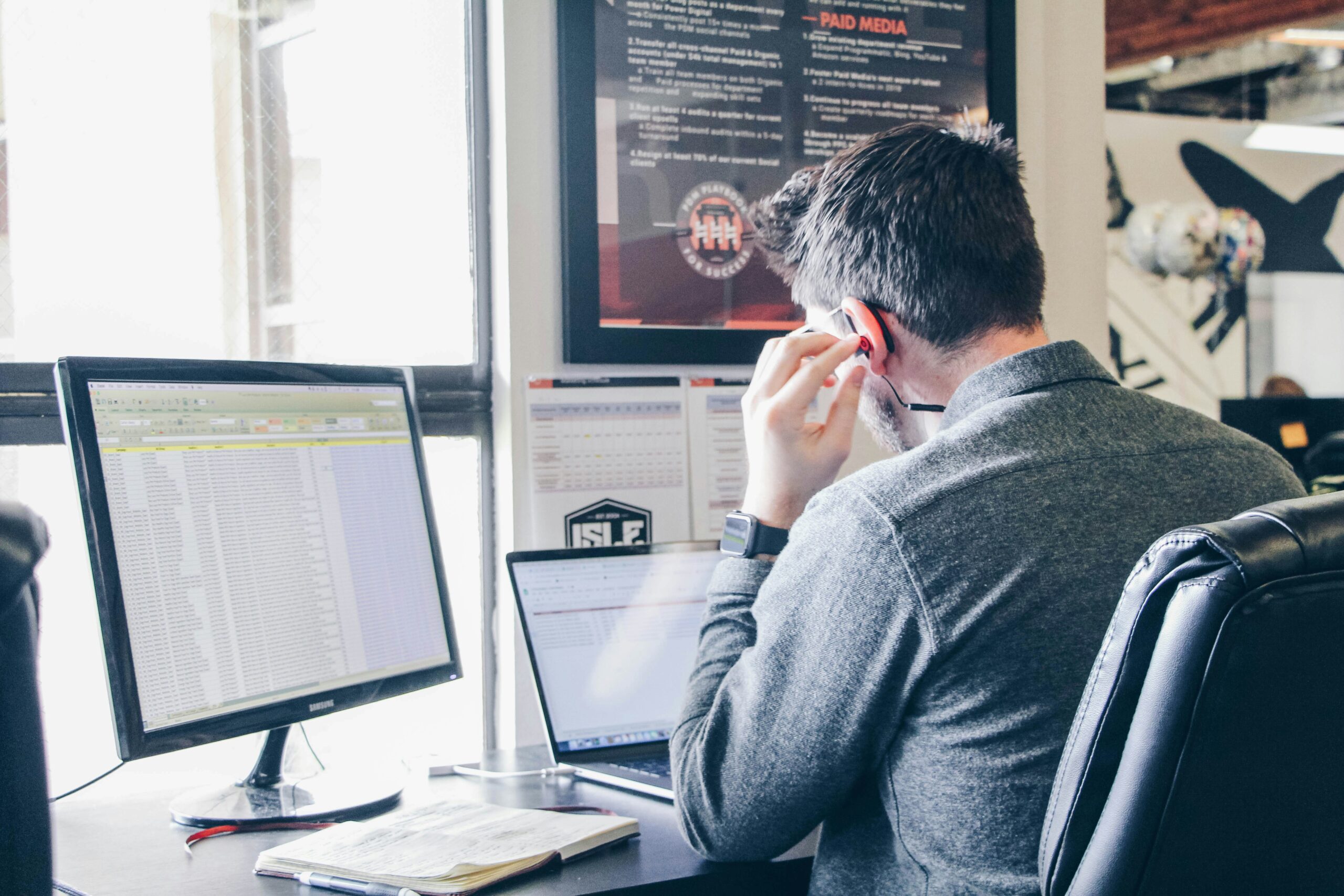
As marketing technologies evolve, machine learning continues to reshape how brands engage customers, optimize strategies, and stay competitive. Understanding emerging trends helps marketers prepare for what’s next and adopt innovations that drive measurable impact.
Evolving Algorithms for Deeper Personalization
Machine learning models are becoming more advanced in analyzing customer behavior and preferences. Future algorithms will deliver even more precise personalization—automatically adjusting messaging, content, and offers in real time based on contextual signals like location, device, and past interactions. This will enable brands to craft ultra-relevant experiences at scale.
Integrated AI and Automation Platforms
The future of marketing automation will center around unified platforms that combine machine learning, predictive analytics, and AI-driven workflows. These all-in-one solutions will simplify campaign execution, allow real-time optimization, and help marketing teams align strategy across channels—reducing silos and improving efficiency.
Expansion of A/B Testing With Adaptive Learning
Traditional A/B testing is evolving into adaptive experimentation, where machine learning models test multiple variants simultaneously and adjust campaigns automatically based on performance. This dynamic approach enables faster insights, continuous improvement, and better results without manual intervention.
Increased Focus on Ethical AI and Data Transparency
As personalization becomes more sophisticated, ethical concerns around data use and algorithmic bias will become more prominent. Brands will need to prioritize transparency, ensure data compliance, and communicate how customer information is used. This builds trust while maintaining responsible AI practices.
Conclusion
Maximizing marketing success with machine learning strategies is vital for brands aiming to enhance engagement and loyalty. By harnessing predictive analytics, businesses can identify leads and optimize campaigns, ensuring relevance in today’s fast-paced market. Personalized data-driven strategiesmustreal-time elevate customer interactions, ultimately improving satisfaction and retention. Embracing these technologies positions organizations to thrive and adapt in an ever-changing marketing landscape.
Comments