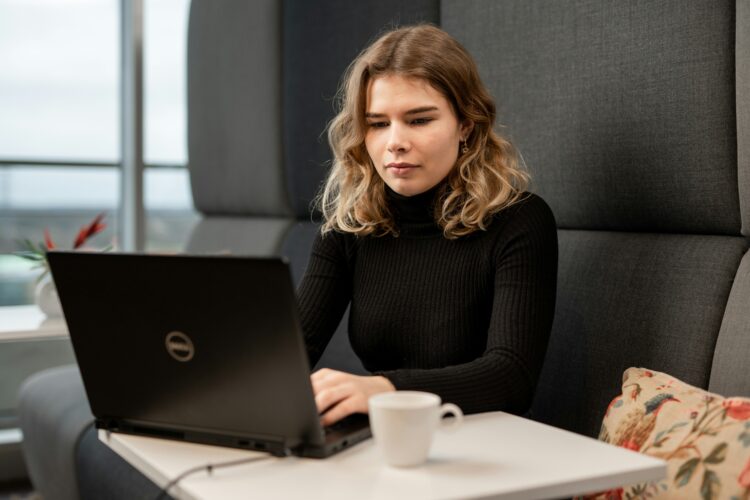
In today’s hyper-competitive digital landscape, success in marketing depends on more than just creativity—it requires precision. Data-driven marketing has become the cornerstone of strategic decision-making, allowing brands to deliver targeted messages, optimize campaigns, and boost investment returns. But as the volume and complexity of consumer data continue to grow, traditional analysis methods fall short.
This is where artificial intelligence (AI) transforms the game. By automating the interpretation of vast data sets and generating predictive insights, AI-powered tools enable marketers to stay ahead of trends, personalize experiences at scale, and refine real-time strategies. From forecasting customer behavior to automating ad spend optimization, AI isn’t just a supporting tool—it’s the engine driving modern marketing forward.
Key Takeaways
- AI predictive tools analyze historical and real-time data to forecast campaign performance and consumer behavior with high accuracy.
- Machine learning algorithms enhance targeting by identifying behavioral patterns and segmenting audiences for more personalized outreach.
- Real-time optimization allows marketers to dynamically adjust campaigns based on performance metrics, improving efficiency and ROI.
- Integrating AI with CRM and analytics platforms streamlines insight generation and reduces manual effort.
- Privacy-compliant AI systems ensure marketing strategies align with data protection regulations like GDPR and CCPA.
- Continuous model training and data integration enable businesses to adapt quickly to changing market dynamics and customer expectations.
The Role of AI in Modern Data-Driven Marketing

As marketing becomes more data-centric, artificial intelligence transforms how marketers plan, execute, and optimize campaigns. Traditional methods of analyzing customer data are no longer sufficient in a landscape where speed, accuracy, and scale are critical. AI bridges this gap by automating complex analysis, offering real-time insights, and continuously learning from new data. This enables marketers to move from reactive decision-making to predictive, forward-looking strategies.
By integrating AI into the marketing stack, organizations can better understand their customers, personalize content delivery, and drive measurable improvements across every stage of the buyer journey.
How AI Enhances Data Interpretation
AI systems process large volumes of structured and unstructured data—from website behavior and CRM entries to social media interactions. Unlike traditional analytics, AI can detect nuanced patterns and correlations across diverse data points. This allows marketers to uncover insights that might otherwise remain hidden.
For example, machine learning algorithms can analyze browsing behavior alongside purchase history to reveal when a customer is most likely to convert, or which content formats drive the highest engagement. These insights guide more strategic content creation, lead nurturing, and timing decisions.
Predictive Analytics for Smarter Campaign Decisions
Predictive analytics enables marketers to make informed decisions before campaigns even launch. AI models generate forecasts that inform content direction, budget allocation, and distribution timing by analyzing historical data, customer touchpoints, and market trends.
For instance, predictive tools can project which customer segments will most likely respond to a specific message or offer. This empowers marketing teams to prioritize high-value opportunities, reduce customer acquisition costs, and improve overall conversion rates.
Real-Time Optimization Through AI
AI tools don’t just analyze past data—they also operate in real time. By continuously monitoring key performance indicators (KPIs), AI systems can suggest or automatically apply optimizations to active campaigns. This includes reallocating budget, tweaking messaging, or adjusting bidding strategies based on performance signals.
Such real-time agility means marketers can respond to underperformance quickly, rather than waiting for post-campaign reports. As a result, campaigns stay aligned with audience behavior and deliver better ROI throughout their lifecycle.
Forecasting and Trend Prediction with AI Tools
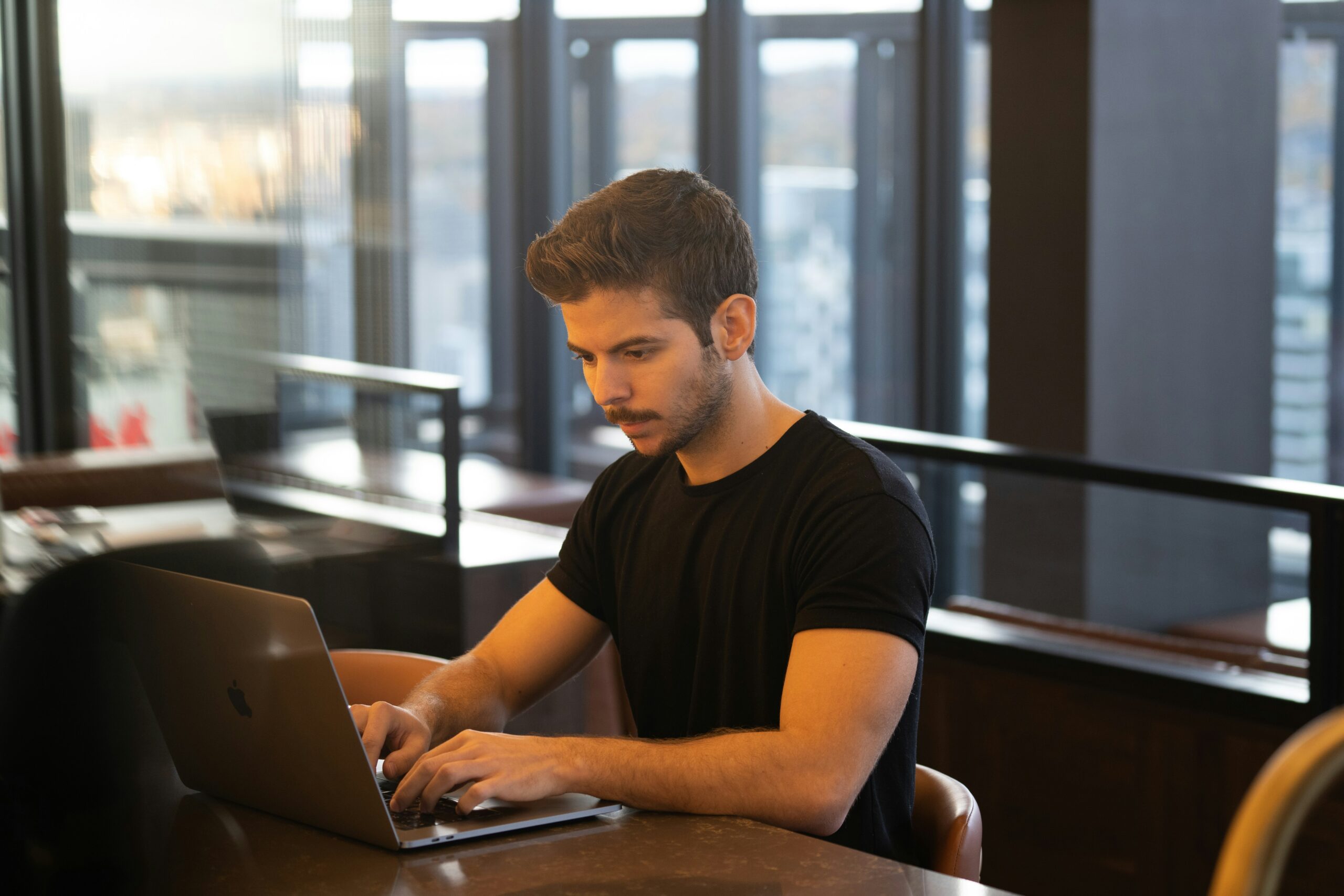
In an environment where consumer behavior and market conditions evolve rapidly, the ability to predict trends gives marketers a decisive edge. AI forecasting tools analyze historical data alongside real-time inputs to generate forward-looking insights, allowing marketing teams to plan more effectively, reduce waste, and capitalize on emerging opportunities.
AI-powered trend prediction goes beyond reactive reporting—it anticipates what customers will want and when, enabling smarter campaign planning, product promotion, and audience engagement.
Techniques for AI-Based Forecasting
AI forecasting relies on various statistical and machine learning methods, such as time-series analysis, regression models, and deep learning. To predict future performance, these techniques evaluate seasonality, purchasing patterns, demographic shifts, and external signals (like search trends or economic indicators).
For example, time-series analysis can help forecast traffic spikes during seasonal events, while regression models estimate how different variables—like email frequency or ad budget—impact conversion rates. These models grow more accurate as they ingest more data over time.
Applications in Seasonal and Behavioral Trend Planning
Marketers can use AI forecasts to precisely plan campaign timing, inventory levels, and promotional schedules. For instance, an e-commerce retailer might use AI tools to predict when shoppers begin holiday purchases, allowing them to roll out content and offers ahead of competitors.
AI also identifies behavioral patterns that signal emerging interests or pain points, helping marketers adjust messaging before trends peak. This proactive approach reduces lag time in campaign execution and aligns content with consumer expectations.
Forecasting Consumer Demand and Content Needs
Beyond campaign-level insights, AI forecasting helps brands predict consumer preferences and demand shifts. By analyzing product interest, sentiment data, and engagement trends, AI can indicate which offerings are gaining momentum and which topics are likely to resonate with audiences shortly.
For example, AI tools might flag a rising interest in sustainability among a brand’s audience, prompting the team to highlight eco-friendly product features in upcoming campaigns.
Enhancing Customer Segmentation and Targeting with AI
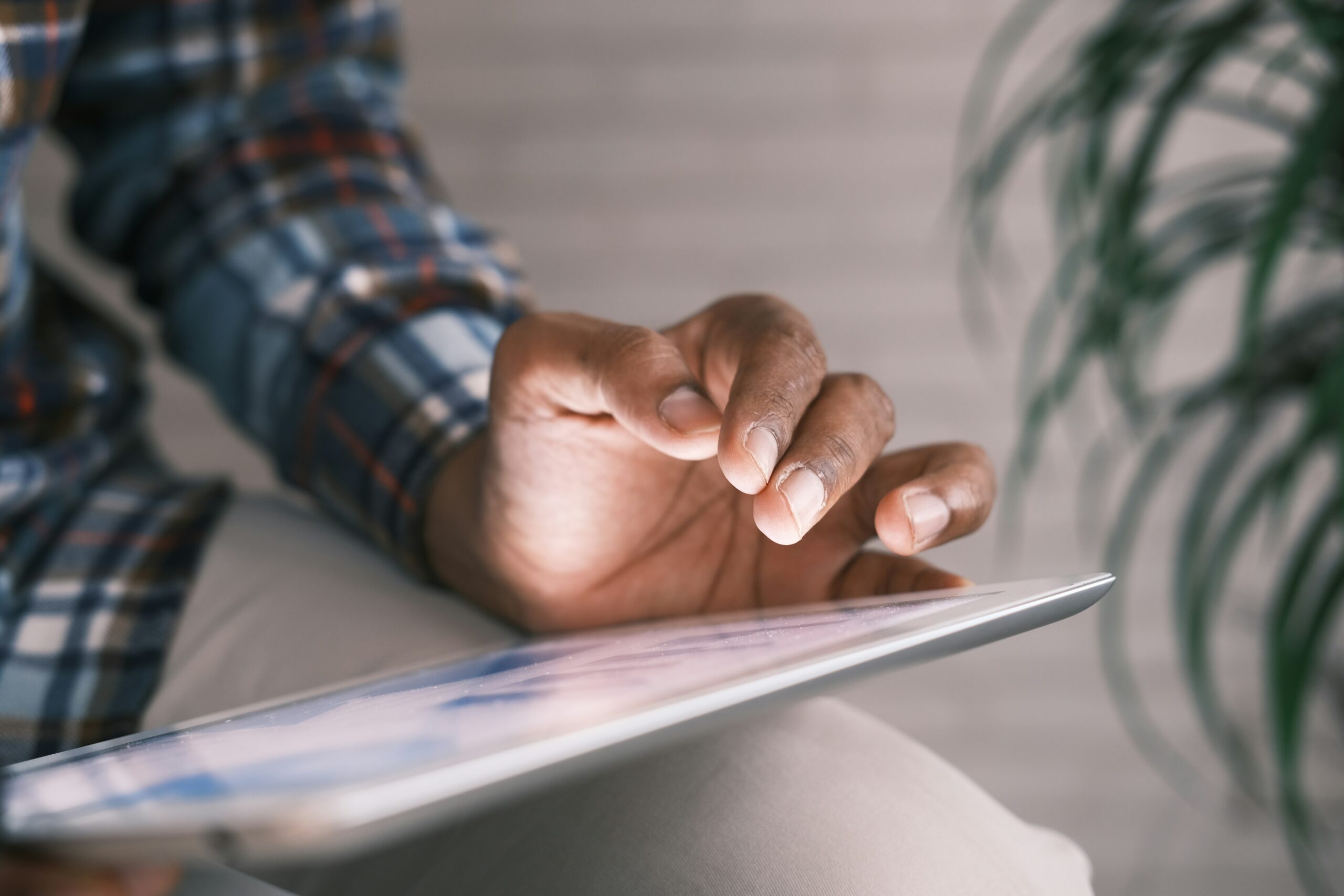
Effective customer segmentation is the foundation of personalized marketing—and AI takes this process far beyond traditional demographic filters. By analyzing behavior, preferences, and interactions at scale, AI-driven tools create detailed customer profiles that help marketers deliver highly targeted and relevant messages. This advanced segmentation not only improves campaign performance but also builds stronger relationships with consumers by ensuring content speaks directly to their needs, interests, and timing.
Behavior-Based Audience Profiling
AI platforms analyze a wide range of behavioral data, including browsing patterns, email engagement, social media activity, and transaction history. These insights go beyond static audience definitions and allow marketers to build dynamic profiles that evolve with each interaction.
For example, a customer who frequently browses a particular product category but hasn’t made a purchase might be segmented into a “high-intent, low-conversion” group—prompting a targeted follow-up campaign designed to overcome hesitation or objections.
Clustering Algorithms for Micro-Segmentation
Using unsupervised learning techniques like clustering, AI can uncover micro-segments that marketers might not have identified manually. These algorithms group users based on shared behaviors or preferences—even when they don’t fit into conventional demographic buckets.
This kind of micro-segmentation allows for extremely precise targeting. A brand might discover, for example, a group of users who frequently buy products late at night and respond best to SMS promotions—information that would be hard to identify without AI analysis.
NLP and Sentiment Analysis for Audience Insights
Natural Language Processing (NLP) enables AI to interpret qualitative data like customer reviews, survey responses, and social media comments. Sentiment analysis tools assess the tone and emotion behind this content to reveal how audiences feel about products, services, or messaging.
These insights help marketers refine their tone of voice, identify emerging issues, and tailor campaigns to match the emotional landscape of their audience. For instance, detecting a rise in frustration around delivery times could prompt an email campaign that reassures customers about shipping improvements.
Improving Campaign ROI and Operational Efficiency
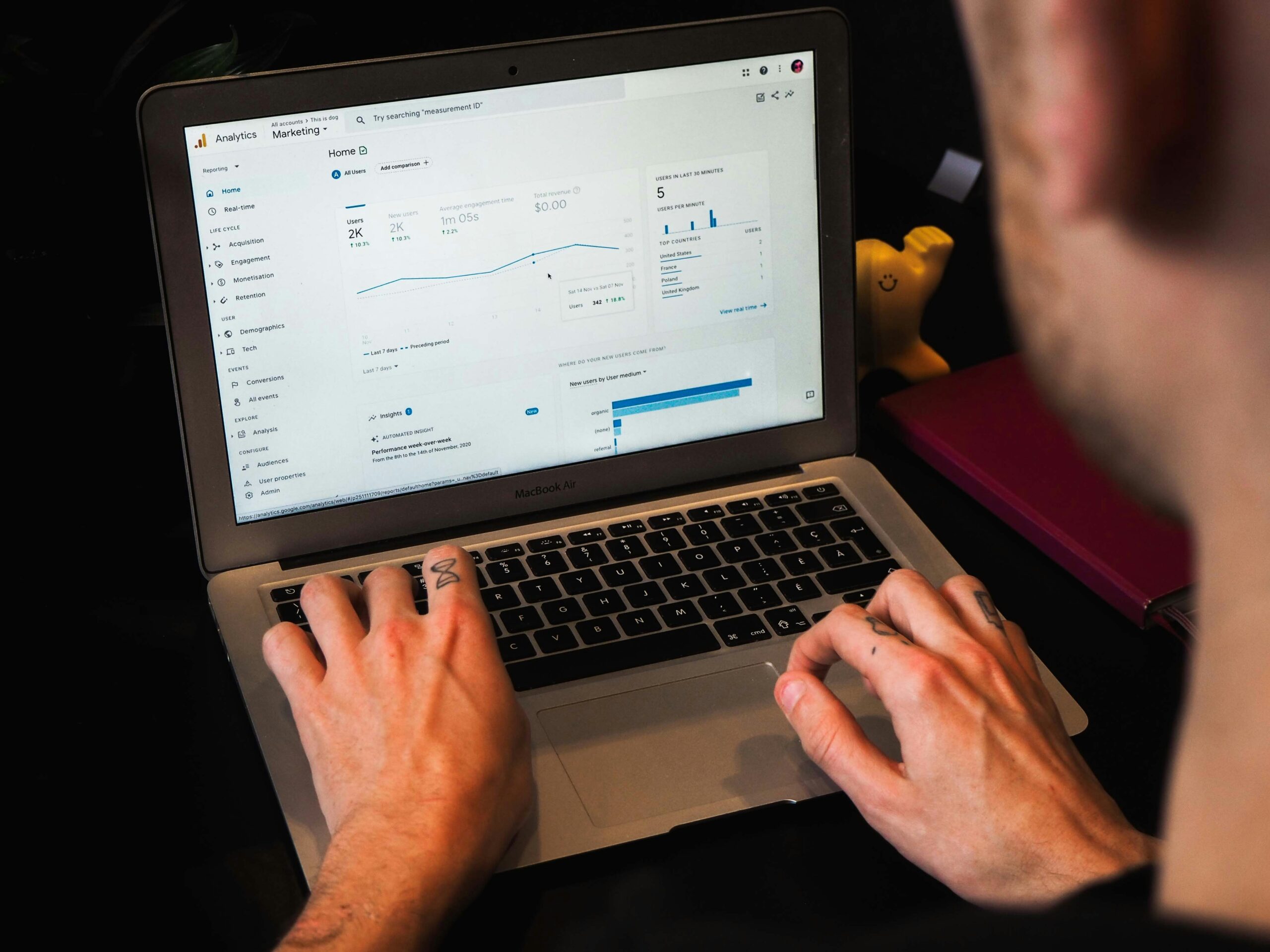
Maximizing return on investment (ROI) and reducing waste are top priorities in any marketing strategy. AI tools can support these goals by streamlining decision-making, optimizing resources in real time, and automating labor-intensive processes. From budget reallocation to performance tracking, AI not only speeds up execution but also enhances the precision of every campaign element. The result is a more agile marketing operation with higher impact and lower costs.
Dynamic Budget Allocation and Ad Spend Optimization
AI tools analyze performance data across channels to reallocate budget where it yields the highest returns continuously. Instead of relying on static budget plans, marketers can shift spending mid-campaign, favoring top-performing platforms, ads, or audiences. For example, if an AI system detects that Instagram ads outperform Google Display Network ads significantly, it can automatically divert more of the budget to Instagram. This dynamic optimization ensures that every dollar works harder.
Automated A/B Testing for Creative Efficiency
Traditional A/B testing often limits marketers to evaluating one or two variables at a time. AI-powered testing expands this capacity by simultaneously testing multiple creatives, headlines, or audience segments, then identifying the highest-performing combinations quickly. This automation accelerates the creative decision-making cycle. Instead of waiting days or weeks for results, marketers get near-instant feedback and can iterate faster, improving engagement rates and cutting down campaign waste.
Reducing Acquisition Costs Through Predictive Targeting
AI helps lower customer acquisition costs (CAC) by identifying which leads are most likely to convert and which channels generate the best results. Predictive scoring models assess engagement frequency, behavior patterns, and historical conversions to prioritize high-value prospects. Marketers avoid overexposure and minimize ad spend on unqualified leads by focusing resources on the most promising segments. This leads to higher conversion rates and a more efficient sales funnel.
Implementing AI in Your Marketing Infrastructure
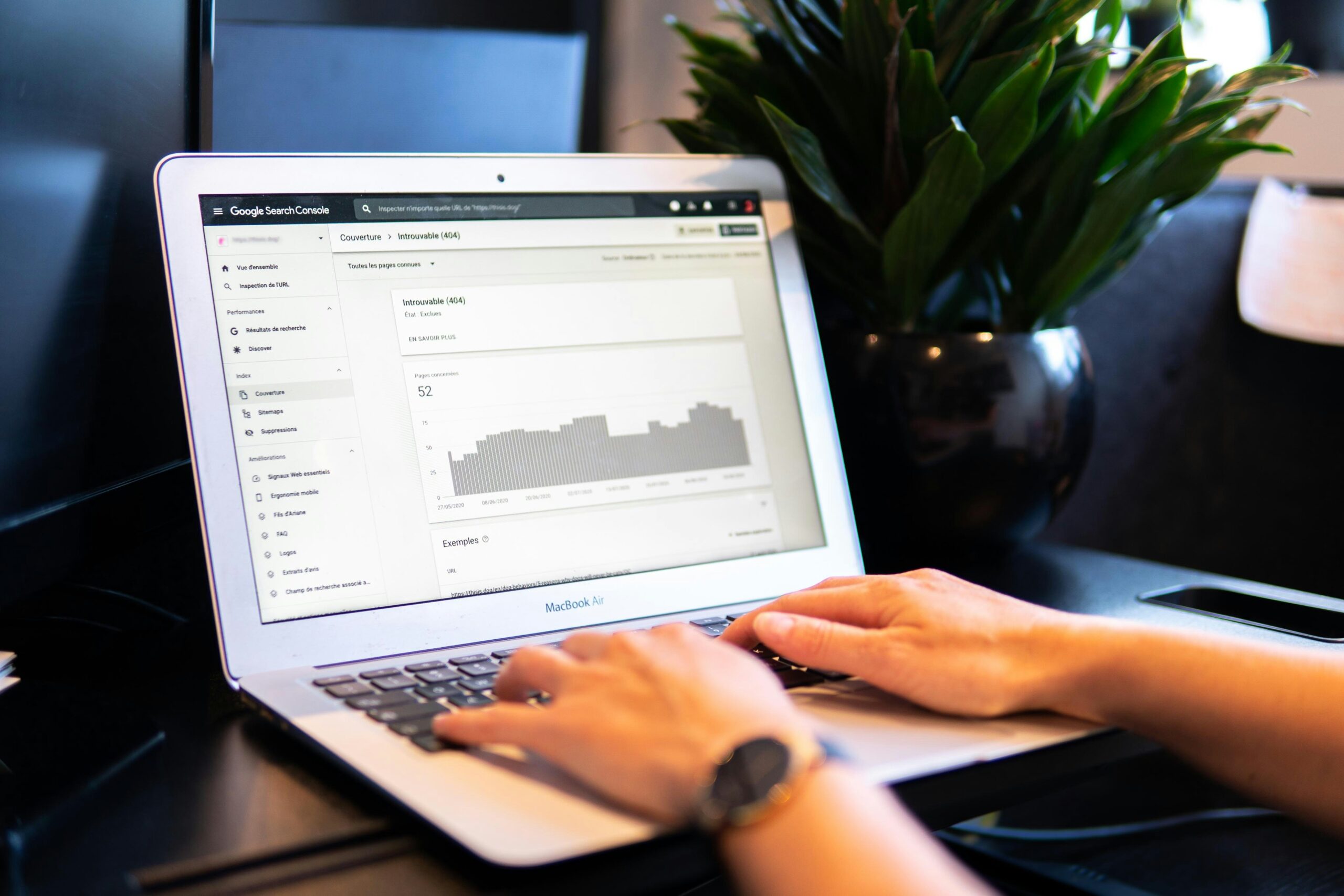
Adopting AI in marketing is more than a plug-and-play process—it requires thoughtful planning, system compatibility, and organizational alignment. To maximize ROI and ensure a smooth transition, businesses should approach AI integration in phases, starting with pilot programs and gradually scaling based on performance outcomes.
A well-executed implementation enhances existing workflows and builds a sustainable framework for long-term innovation and data-driven agility.
Preparing Your Data Ecosystem
AI tools rely on clean, structured, and centralized data to generate accurate insights. Before implementation, marketers must evaluate the quality of their data sources—CRM entries, web analytics, email platforms—and resolve inconsistencies or gaps.
This process often involves conducting a data audit, standardizing data formats, and integrating disparate systems to create a unified data environment. Even the most advanced AI solutions will produce unreliable or biased results without a strong data foundation.
Choosing the Right AI Tools for Marketing Goals
There’s no one-size-fits-all AI platform. The right tools depend on a company’s marketing objectives, available resources, and existing technology stack. Whether it’s predictive analytics, customer segmentation, content personalization, or automated testing—each function may require a specialized solution.
When evaluating AI platforms, marketers should consider factors like ease of integration, scalability, user interface, data security protocols, and the availability of customer support or onboarding resources.
Training and Adoption Across Marketing Teams
For AI to deliver value, marketers need to understand how to use the tools effectively. This means investing in training programs that empower teams to interpret AI-generated insights, adjust strategies based on recommendations, and monitor ongoing performance.
Encouraging collaboration between marketing, data science, and IT teams ensures smoother integration and more actionable outcomes. Over time, as comfort with AI grows, organizations can embed AI workflows into daily campaign management and strategic planning.
Data Privacy and Compliance in AI-Enhanced Marketing
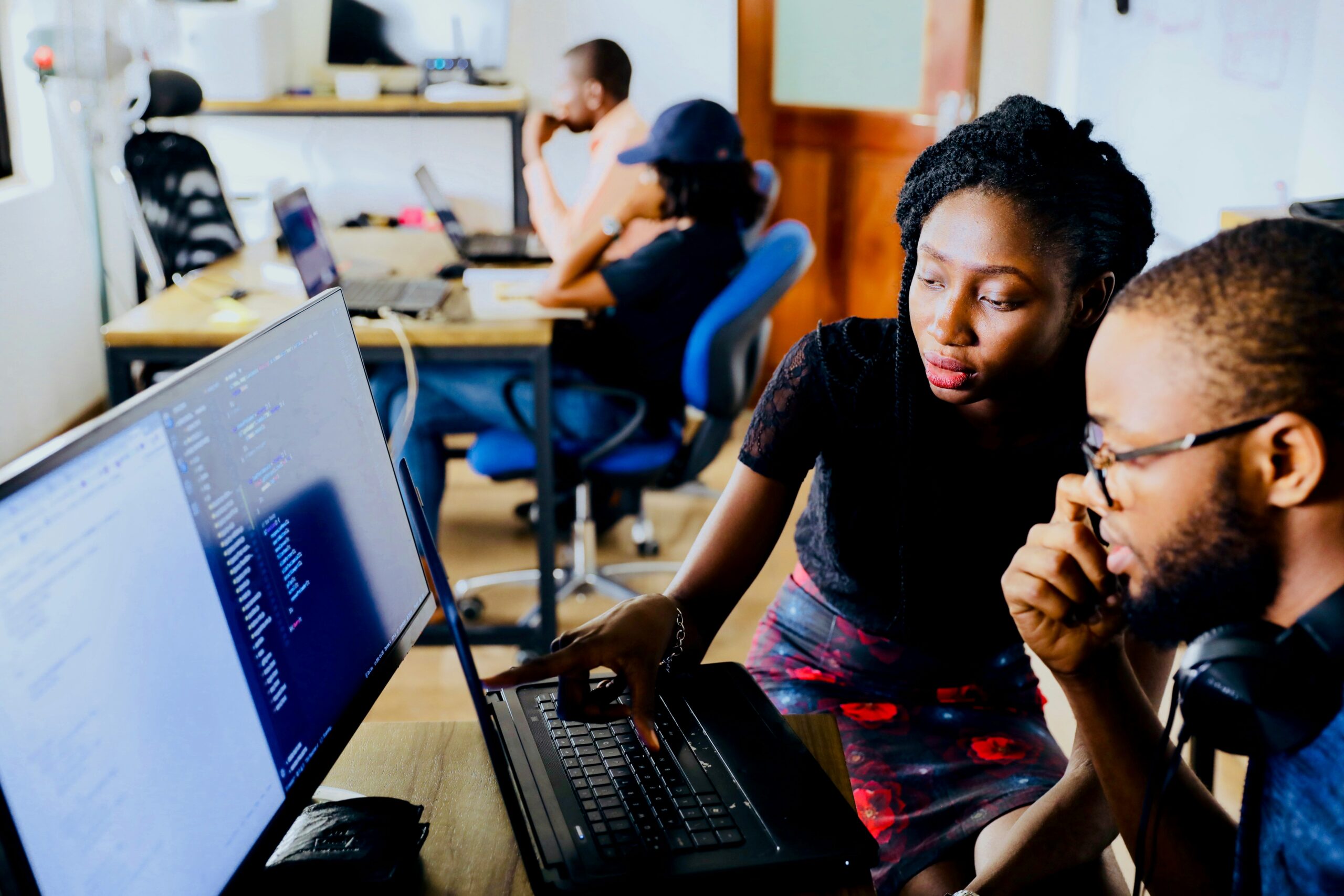
As AI tools become integral to marketing, ensuring data privacy and regulatory compliance is non-negotiable. Consumers are more aware of how their information is used, and global regulations such as the General Data Protection Regulation (GDPR) and the California Consumer Privacy Act (CCPA) place strict requirements on businesses handling personal data. AI platforms must be implemented responsibly—not only to protect customer trust but also to avoid legal and financial risks. Marketers need to work closely with data governance teams to align AI initiatives with compliance standards from the ground up.
Built-In Compliance Features of AI Tools
Many modern AI platforms come with embedded features designed to support regulatory compliance. These include data anonymization, opt-in consent tracking, automated access logs, and data minimization protocols. For example, an AI system might mask personally identifiable information (PII) during analysis to ensure data privacy is maintained. Such features help businesses leverage customer data ethically without compromising security or violating legal frameworks.
Aligning with Regulations Like GDPR and CCPA
To remain compliant, businesses must ensure that AI tools are configured in a way that respects consumer rights. This includes:
- Giving users the ability to opt out of data tracking or profiling.
- Being transparent about how AI is used in personalization or targeting.
- Ensuring that collected data is stored securely and processed only for its intended purpose.
- Responding promptly to consumer data requests (access, correction, deletion).
Regularly reviewing internal data handling policies and updating them in line with evolving regulations is essential. Compliance should be treated as an ongoing process—not a one-time checklist.
Building Consumer Trust Through Ethical AI Use
Beyond legal requirements, ethical data practices are key to building long-term relationships with customers. Transparency about how AI systems make decisions, coupled with a clear privacy policy, fosters confidence in your brand. When customers understand that AI is being used to improve their experience—rather than exploit their information—they’re more likely to engage, convert, and stay loyal.
Conclusion
Artificial intelligence has fundamentally reshaped how marketers approach data-driven strategies. No longer limited to reactive analysis, AI enables proactive planning, personalized engagement, and continuous optimization at scale. From predictive modeling and customer segmentation to real-time budget adjustments and automated testing, AI tools empower marketers to deliver more brilliant campaigns with greater precision and efficiency.
However, successful AI integration goes beyond technology—it requires clean data, cross-team collaboration, and a commitment to privacy and compliance. Businesses that invest in these areas will enhance marketing ROI and future-proof their strategies against shifting consumer behavior and market dynamics. As AI capabilities continue to evolve, marketers who thoughtfully embrace these tools will be best positioned to lead with insight, agility, and impact.
Frequently Asked Questions
How quickly can businesses see results after implementing AI marketing tools?
Many organizations report noticeable improvements in campaign performance within the first few weeks. However, the most impactful results—such as improved ROI, reduced customer acquisition costs, and better segmentation—often emerge over several campaign cycles as the AI models refine their predictions.
Can small businesses benefit from AI in marketing, or is it just for large enterprises?
Absolutely. Many AI-driven marketing tools are now scalable and affordable, making them accessible to small and mid-sized businesses. These tools help automate tasks like customer segmentation, email targeting, and performance reporting—leveling the playing field.
What data do AI tools need to work effectively?
AI tools require a mix of structured and unstructured data, such as website activity, CRM records, email interactions, purchase history, and social media engagement. The quality, completeness, and consistency of this data directly impact the accuracy of AI-generated insights.
Are AI marketing tools compliant with privacy laws like GDPR and CCPA?
Most reputable AI platforms are designed with compliance in mind and include features such as consent tracking, data anonymization, and access controls. However, it’s the company’s responsibility to configure and use these tools in accordance with applicable regulations.
Do marketers need technical skills to use AI tools effectively?
While some foundational knowledge of data analytics is helpful, many AI marketing platforms now offer intuitive interfaces and automated reporting. Marketers primarily need to understand how to interpret the insights and apply them to campaign strategies—technical support or collaboration with data teams can cover more advanced needs.
Comments