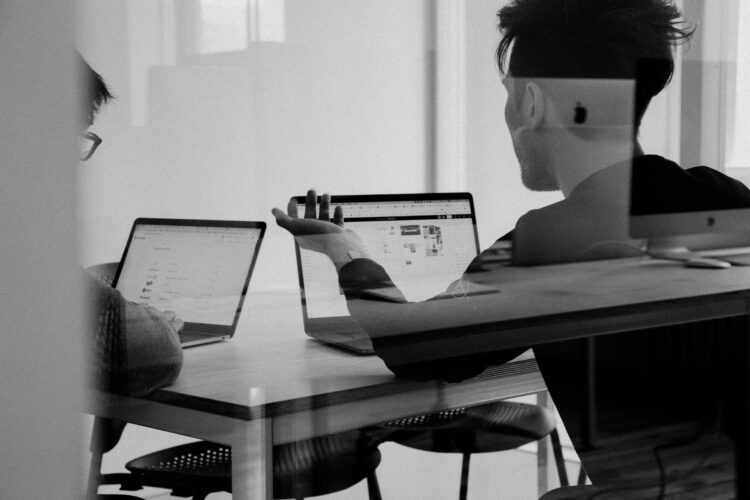
As today’s consumers demand more personalized experiences, traditional segmentation methods based on broad demographics are no longer enough. To stay competitive, marketers are turning to AI-driven segmentation. This data-powered approach uses machine learning and predictive analytics to identify customer patterns, behaviors, and preferences at a granular level. This advanced strategy enables brands to deliver the right message to the right audience at the right time, driving higher engagement, better retention, and improved ROI. In this blog, we’ll explore how AI transforms segmentation strategies and empowers marketers to create smarter, more adaptive campaigns.
Key Takeaways
- AI-driven segmentation uses machine learning and predictive analytics to create more accurate and actionable customer groups.
- Behavioral, demographic, and psychographic data are integrated to build deeper, real-time customer profiles.
- Real-time analytics enable marketers to adjust campaigns instantly based on shifting customer behavior.
- Predictive modeling improves personalization, increases retention, and optimizes marketing spend.
- Data quality, privacy compliance, and ethical AI practices are essential for reliable segmentation outcomes.
- Emerging technologies like NLP and neural networks are expanding the precision and flexibility of customer targeting.
What Is AI-Driven Segmentation in Marketing?
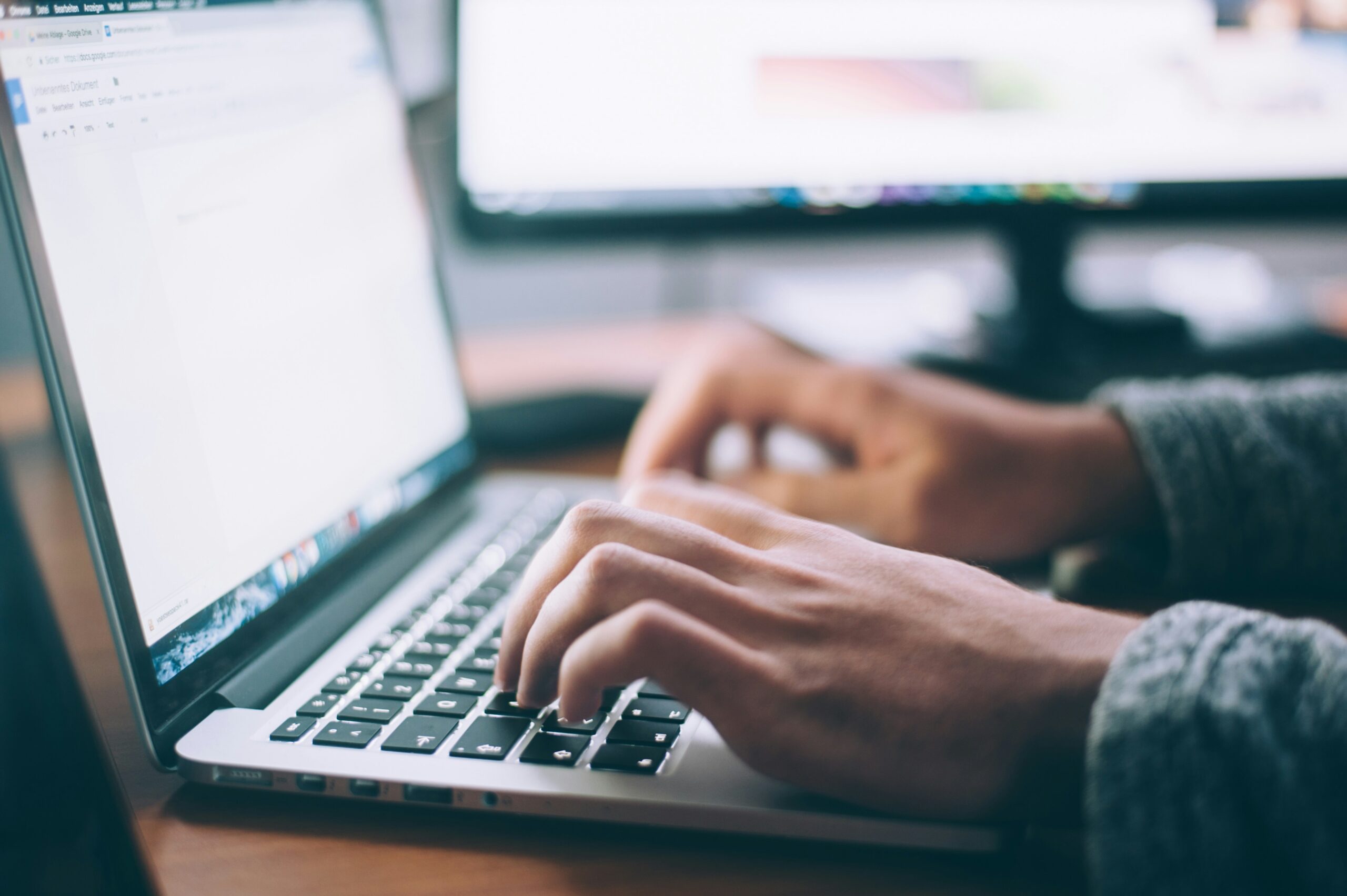
AI-driven segmentation is the process of using artificial intelligence to analyze customer data and divide audiences into highly specific, actionable segments. Unlike traditional methods that group customers based on broad attributes like age or income, AI uses behavioral signals, engagement patterns, and predictive insights to uncover deeper relationships in customer data. The result is smarter targeting and more personalized marketing across every channel.
How AI Goes Beyond Traditional Segmentation
Conventional segmentation relies on fixed criteria such as demographics or geographic location—helpful, but limited in capturing real-time behavior or intent. AI enhances this process by applying machine learning algorithms that adapt to new data, uncover hidden patterns, and predict customer preferences. This shift enables marketers to move from static customer groups to dynamic, evolving profiles that reflect current behaviors and future potential.
Core Technologies Powering AI Segmentation
Several advanced AI technologies work together to make segmentation more accurate and scalable:
- Machine Learning Algorithms – Tools like decision trees, clustering, and neural networks help detect patterns in large datasets and automate segmentation.
- Natural Language Processing (NLP) – NLP analyzes unstructured data, such as customer reviews or social media comments, to gauge sentiment and intent.
- Predictive Analytics – These models forecast customer behavior, such as likelihood to purchase or churn, allowing proactive campaign planning.
By combining these technologies, marketers gain a real-time, 360-degree view of their customers, making it possible to deliver the right message to the right person at the right moment.
Advanced Techniques for Data-Driven Segmentation
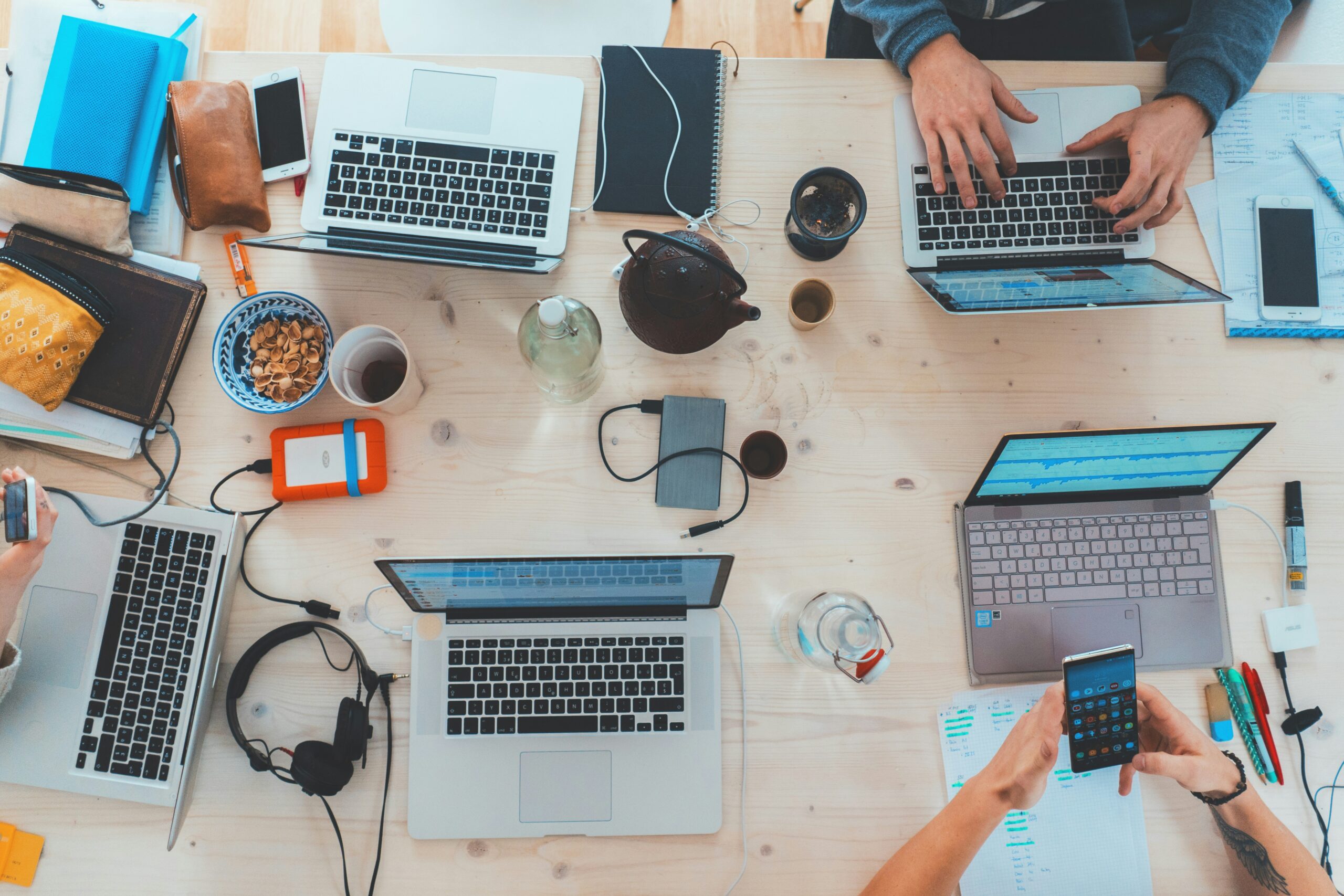
As customer data becomes more diverse and abundant, advanced AI techniques offer new ways to segment audiences more precisely. By combining structured and unstructured data—like transactions, web activity, social sentiment, and mobile usage—marketers can build dynamic, multi-dimensional customer profiles that go far beyond basic demographics.
Blending Behavioral and Demographic Data
Traditional segmentation typically focuses on static factors like age, income, or location. AI allows you to layer these variables with real-time behavioral data—such as browsing habits, content interactions, and purchase frequency—to create richer, more insightful customer segments.
Examples include:
- Grouping users by how often they engage with content, combined with average order value
- Identifying patterns like abandoned carts among a specific age group or device type
This combination creates segments that are not only descriptive but also predictive of future actions.
Leveraging Social Listening and Sentiment Analysis
Using Natural Language Processing (NLP), AI tools can scan and interpret unstructured data from customer reviews, social media, and open-ended survey responses. This helps marketers:
- Detect emotional drivers behind purchase decisions
- Understand brand perception in real time
- Identify micro-segments based on customer sentiment
For instance, you might segment customers who frequently mention “value for money” versus those who prioritize “premium quality,” allowing you to tailor messaging accordingly.
Real-Time Segmentation With Continuous Data Feeds
Modern AI systems process data as it arrives, enabling real-time segmentation that evolves alongside customer behavior. This agility is crucial for staying relevant in fast-moving markets.
Benefits of real-time segmentation include:
- Triggering instant offers or messages based on recent interactions
- Automatically moving users between segments as behavior shifts
- Ensuring personalization remains up-to-date and effective
These advanced techniques turn raw data into responsive, customer-centric marketing strategies that drive higher engagement and loyalty.
Predictive Analytics and Customer Insight
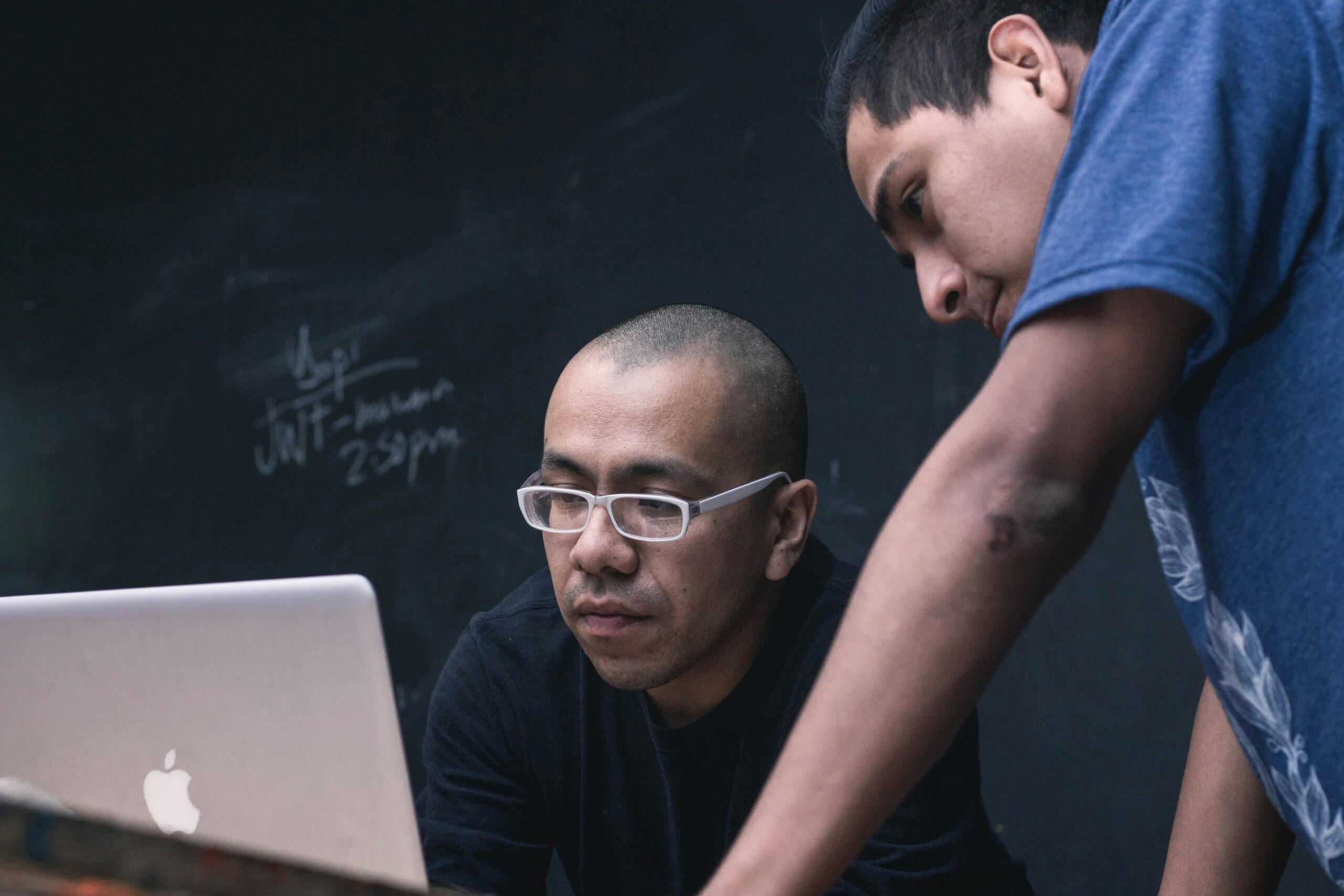
Predictive analytics takes segmentation a step further by forecasting future customer behavior using historical data, trends, and AI-powered modeling. Rather than reacting to customer actions after they happen, marketers can proactively engage customers based on likely outcomes—whether it’s a purchase, churn, or product interest.
Forecasting Customer Behavior With Predictive Models
By analyzing patterns in past behavior, AI models can estimate what a customer is likely to do next. This enables marketers to:
- Identify high-value customers early
- Predict churn and intervene with retention tactics
- Time communications based on purchase intent
For example, predictive models might flag customers who haven’t purchased in 30 days and recommend sending a re-engagement offer, boosting retention before churn occurs.
Enhancing Campaign Effectiveness Through Forecasting
AI-driven forecasting helps refine marketing strategies in real time. Instead of static campaigns, predictive analytics enables:
- Smarter budget allocation based on segment potential
- Dynamic personalization based on predicted needs
- Scenario planning for different market conditions
Dashboards powered by business intelligence tools often visualize these insights, allowing teams to make quick, data-backed decisions.
Turning Insights Into Actionable Marketing Strategies
The real power of predictive analytics lies in execution. When insights feed directly into your marketing stack—email platforms, ad campaigns, mobile notifications—you can:
- Deliver personalized offers just before a customer is likely to convert
- Adjust ad spend for segments showing the highest intent
- Design content strategies tailored to forecasted interests
Predictive analytics transforms segmentation from a snapshot into a forward-looking engine—helping you anticipate needs, improve timing, and maximize marketing ROI.
Personalization and Campaign Optimization
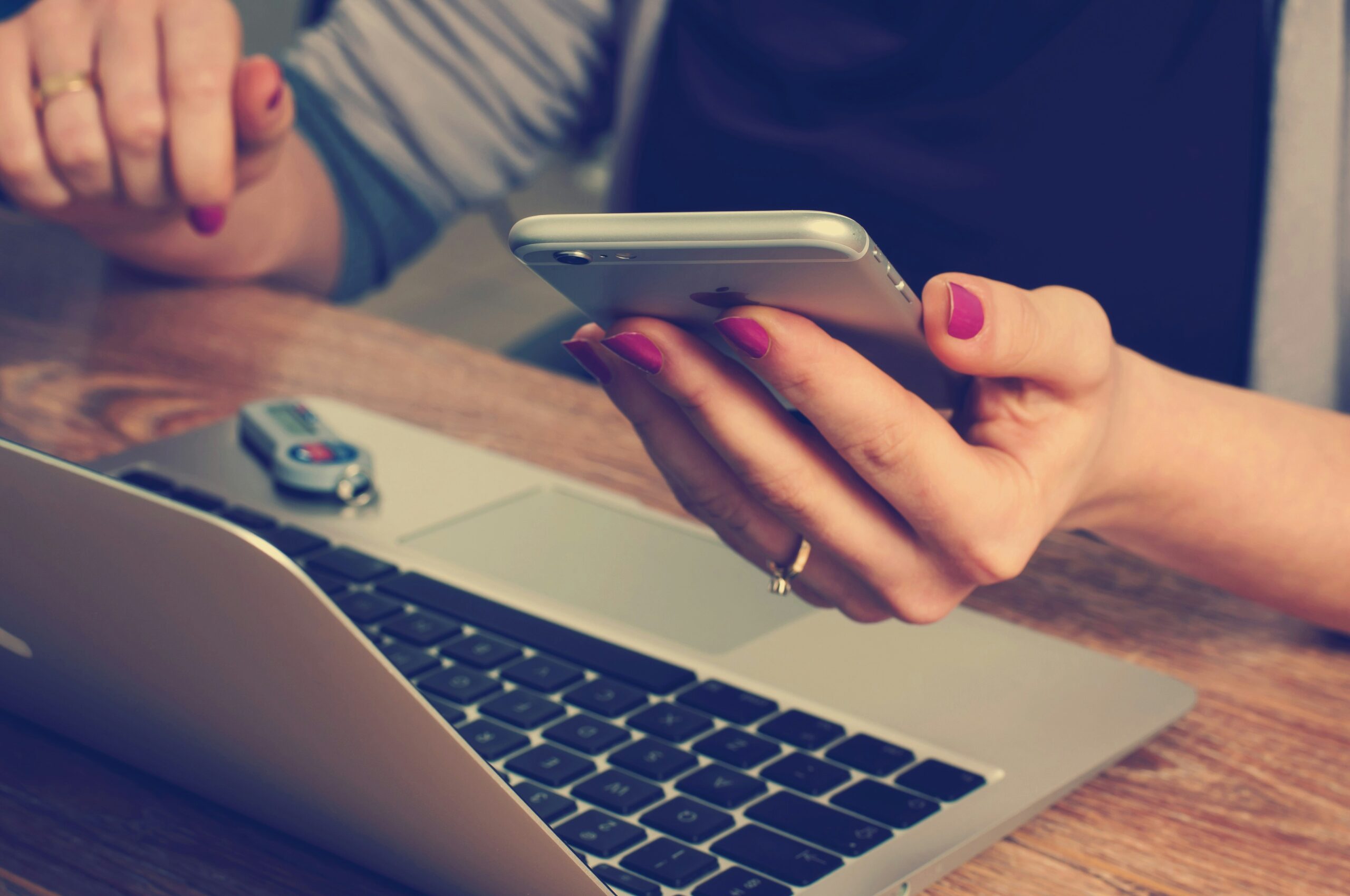
AI-driven segmentation empowers marketers to go beyond basic targeting by creating deeply personalized campaigns. Businesses can significantly increase engagement, conversion rates, and customer loyalty by aligning content, timing, and offers with specific customer behaviors and preferences.
Delivering Hyper-Personalized Content at Scale
AI allows personalizing campaigns across multiple touchpoints—email, website, ads—without manual effort. Key techniques include:
- Dynamic Content Customization – Website banners, email subject lines, and product recommendations can change automatically based on segment data.
- Predictive Product Suggestions – Based on purchase history and browsing behavior, AI models serve up items customers are most likely to buy.
- Cross-Channel Personalization – Messages stay consistent and relevant across email, social, and mobile, improving the customer journey.
This level of personalization builds stronger connections with each audience segment and encourages repeat engagement.
Real-Time Optimization of Marketing Campaigns
AI doesn’t just set and forget—it continuously learns and adjusts. With real-time insights, marketers can:
- Monitor engagement metrics as they happen
- Modify messaging, creative, or offers instantly
- Reallocate budget toward high-performing segments on the fly
For example, if a segment’s email open rate drops, the AI can automatically test a new subject line or delivery time to boost performance.
Continuous Testing and Automated Decision-Making
AI also powers automated A/B testing and multi-variant testing, helping marketers identify what works best for each segment without manual trial-and-error.
- Test different creatives across audience segments
- Use AI to determine winners faster
- Apply results to future campaigns for ongoing improvement
This data-driven loop ensures every campaign becomes smarter and more effective over time.
With AI handling personalization and optimization in tandem, marketers can focus less on guesswork and more on strategy—knowing that every customer is receiving the most relevant experience possible.
Data Management and Ethical Considerations
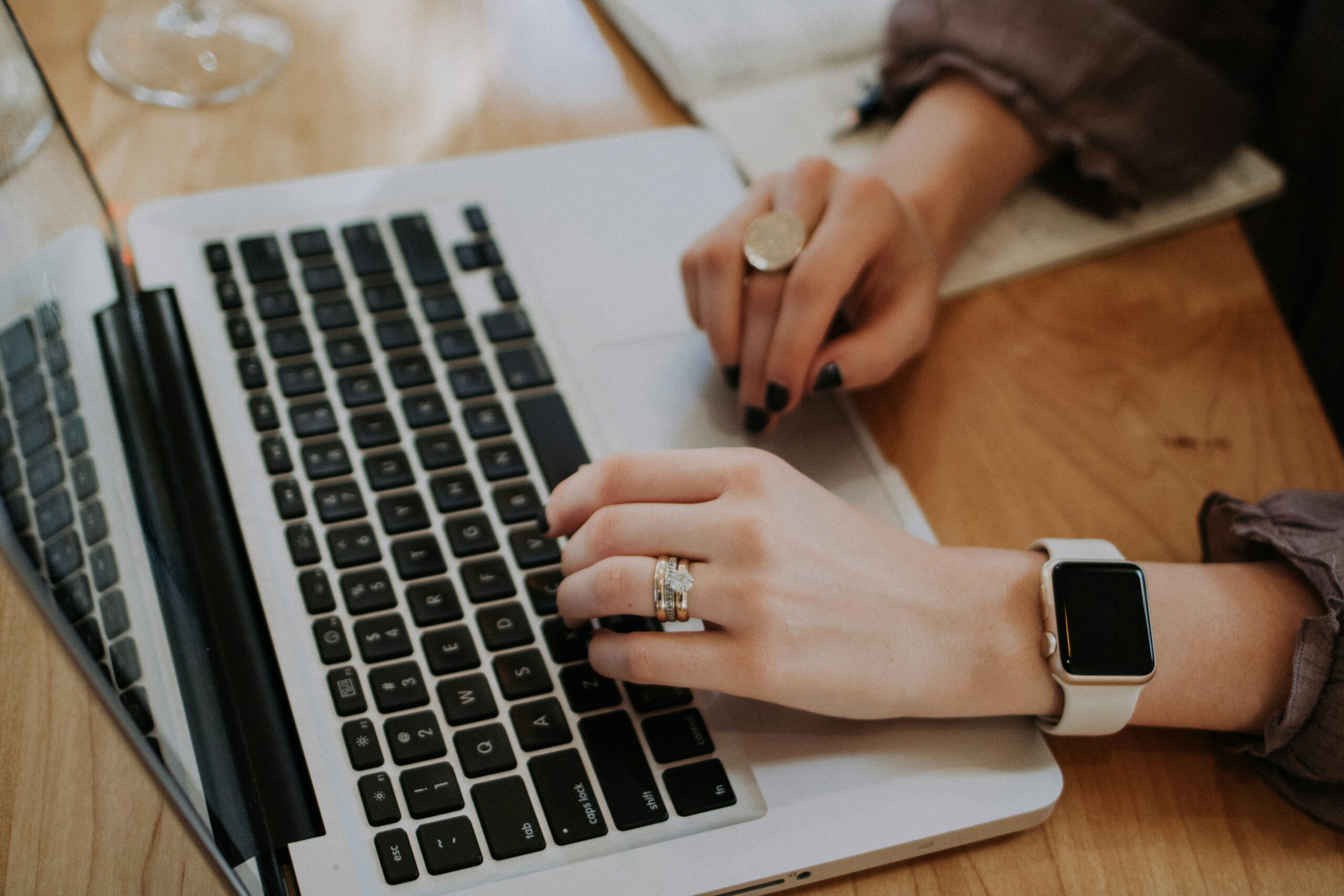
Effective AI-driven segmentation depends on the quality, integrity, and ethical use of data. Without strong data management practices, even the most sophisticated AI models can deliver inaccurate results—or worse, introduce bias and violate customer trust. To succeed, marketers must prioritize clean, compliant, and securely managed data.
Maintaining High Data Quality for Accurate Segmentation
AI models are only as good as the data they’re trained on. Inconsistent, outdated, or duplicate data can distort customer insights and lead to poor targeting decisions.
Key best practices include:
- Data Cleansing – Regularly remove duplicates, fix errors, and fill in missing values using automated tools.
- Standardization – Use uniform formats and naming conventions across all data sources.
- Real-Time Validation – Ensure incoming data is validated at the collection point to prevent errors from entering the system.
High-quality data leads to more precise segmentation, predictions, and trustworthy personalization.
Ensuring Data Security and Privacy Compliance
With rising consumer concerns and strict regulations like GDPR and CCPA, data privacy is non-negotiable. AI segmentation must be built on secure and transparent data practices.
Essential safeguards include:
- Encryption – Secure data at rest and in transit using industry-standard protocols.
- Role-Based Access Controls (RBAC) – Limit data access to authorized users only.
- Regular Security Audits – Conduct audits and penetration testing to detect vulnerabilities.
- Anonymization Techniques – Use data masking or pseudonymization where appropriate to protect personal identifiers.
Demonstrating responsible data practices protects your brand and fosters trust with your audience.
Avoiding Bias in AI Models
Bias can creep into segmentation models if historical data reflects unequal treatment or if algorithms aren’t tested for fairness. Marketers must take steps to minimize these risks.
Recommendations include:
- Audit AI Models Regularly – Check for disparities in how different groups are treated or targeted.
- Train on Diverse Datasets – Ensure data reflects various customer experiences and demographics.
- Apply Ethical Frameworks – Adopt guidelines that govern how AI decisions are made and evaluated.
Ethical AI use is critical to building sustainable, inclusive marketing practices that align with evolving consumer expectations and societal standards.
Future Directions for AI in Segmentation
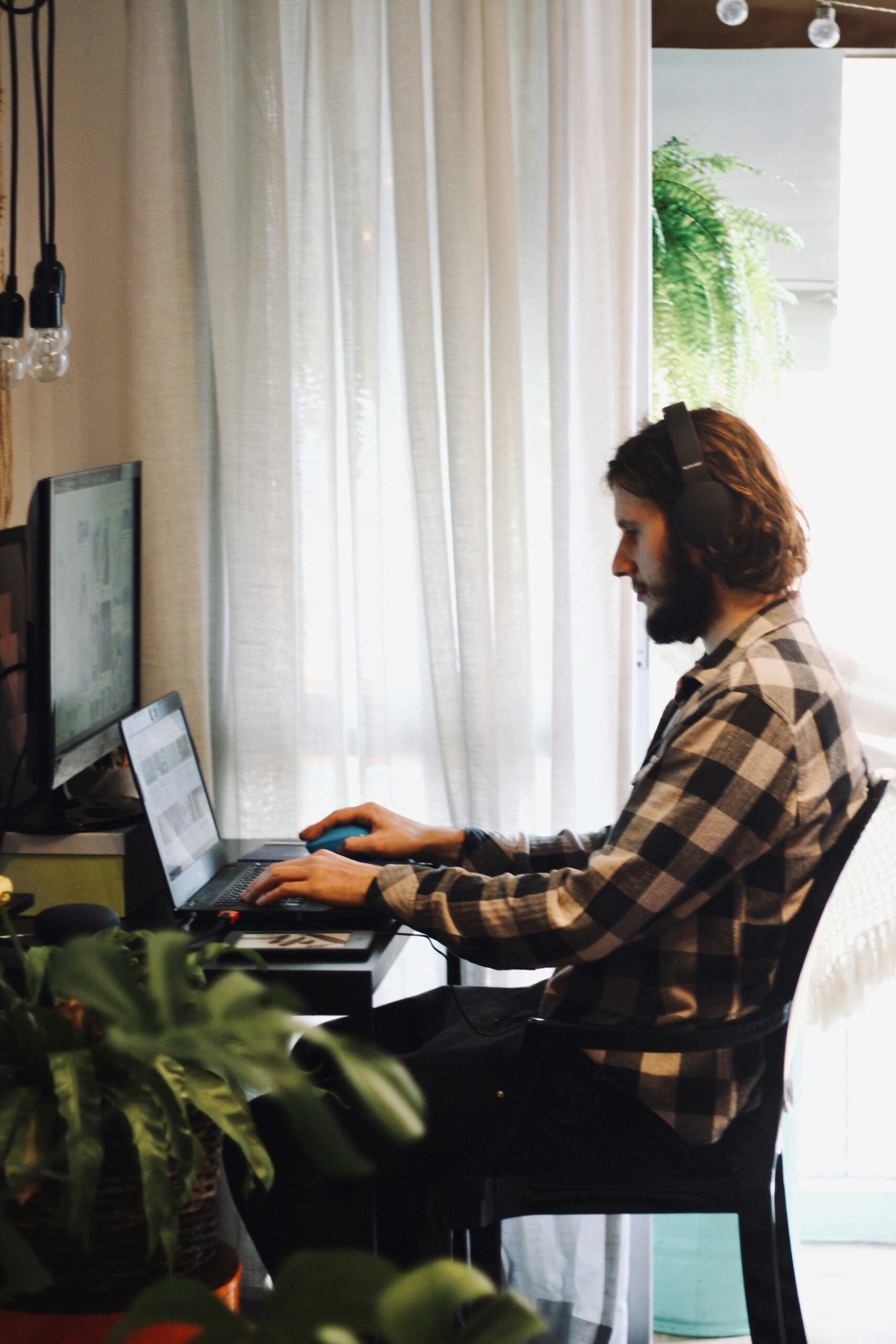
AI-driven segmentation is constantly evolving. As technology advances, new capabilities are emerging that will redefine how marketers understand and engage with their audiences. From deeper contextual analysis to faster decision-making, these innovations promise even greater precision, personalization, and agility.
Emerging Technologies Shaping Customer Segmentation
Several breakthrough technologies are extending the power of AI segmentation and setting the stage for next-generation marketing:
- Advanced Neural Networks – Deep learning models like CNNs and RNNs are improving how AI identifies subtle behavioral patterns across complex data sets.
- Enhanced Natural Language Processing (NLP) – Modern NLP tools can extract sentiment, intent, and emotion from large volumes of text, unlocking deeper insights from customer feedback and social media.
- Edge Computing – Real-time processing at the data source allows marketers to react instantly to user behaviors—especially valuable in mobile and IoT environments.
- IoT Data Integration – Wearables, smart devices, and sensors offer rich behavioral data that can inform context-aware segmentation strategies.
- (Foresight) Quantum Computing – While still emerging, quantum computing could eventually process segmentation models at speeds and scales unreachable with today’s technology.
These technologies not only increase accuracy and speed but also expand the range of inputs marketers can use to build context-rich customer profiles.
Adapting Segmentation to Shifting Consumer Behavior
AI’s greatest strength lies in its adaptability. As consumer preferences evolve due to economic shifts, cultural trends, or digital habits, AI models can update segments in real time—ensuring relevance at every touchpoint.
Key tactics include:
- Continuous Learning Systems – Machine learning models that self-update with new data to keep segmentation accurate and timely.
- Feedback Loops – Real-time feedback from customer interactions (e.g., clicks, conversions, reviews) helps refine models quickly.
- Scenario Simulations – Predictive analytics can simulate market changes and guide contingency planning for future shifts.
Agile segmentation allows marketers to stay ahead of trends, anticipate customer needs, and avoid missed opportunities.
Strategic Recommendations for Marketers
To prepare for the future and scale AI segmentation effectively, marketers should:
- Invest in Flexible, Scalable Data Infrastructure – Cloud-native platforms and automated pipelines ensure smooth data flow and adaptability.
- Encourage Cross-Department Collaboration – Integrate marketing, data science, and IT teams to align goals and optimize segmentation workflows.
- Prioritize Ethics and Governance – Embed data ethics and compliance into every stage of the segmentation process.
- Continuously Optimize AI Models – Regularly retrain models to reflect new business goals and consumer patterns.
- Measure Impact with Precision – Use performance dashboards to monitor segmentation effectiveness and ROI in real time.
By adopting these strategies, marketers can future-proof their segmentation efforts and build a foundation for sustained customer engagement and competitive growth.
Frequently Asked Questions
What is AI-driven segmentation in marketing?
AI-driven segmentation uses machine learning and predictive analytics to group customers based on behavioral patterns, preferences, and real-time interactions. Compared to traditional segmentation based on static demographics, this enables more personalized and effective marketing strategies.
How does AI improve traditional segmentation methods?
AI enhances traditional segmentation by continuously learning from customer data and adapting to changes. Instead of relying solely on age or location, AI factors in browsing history, purchase behavior, sentiment, and engagement trends to create dynamic, accurate customer segments.
Can small and mid-sized businesses use AI segmentation?
Yes. With the rise of cloud-based tools and user-friendly AI platforms, small and mid-sized businesses can now access powerful segmentation capabilities without the need for in-house data science teams.
What types of data are required for effective AI segmentation?
Effective segmentation requires both structured data (like purchase history, demographics) and unstructured data (like customer reviews, social media sentiment). The more diverse and clean your data, the more accurate your AI models will be.
How do I ensure data privacy and compliance in AI segmentation?
Ensure compliance by encrypting sensitive data, restricting access via role-based controls, and following regulations like GDPR and CCPA. Incorporate anonymization techniques and ethical data governance to protect consumer trust and reduce legal risk.
Conclusion
AI-driven segmentation is no longer a luxury—it’s a marketing necessity. Brands that rely solely on traditional segmentation methods will fall behind as consumer expectations for personalization continue to rise. AI enables marketers to go beyond surface-level traits, harnessing behavioral insights, predictive models, and real-time data to deliver highly relevant, individualized experiences at scale.
AI segmentation becomes a strategic advantage when integrated with your existing systems and powered by quality data. It sharpens targeting, improves campaign performance, boosts retention, and ensures that marketing stays aligned with evolving customer behavior. By embracing this approach and preparing for future innovations, brands can build deeper connections with their audiences and turn segmentation into a driver of long-term growth.
Comments