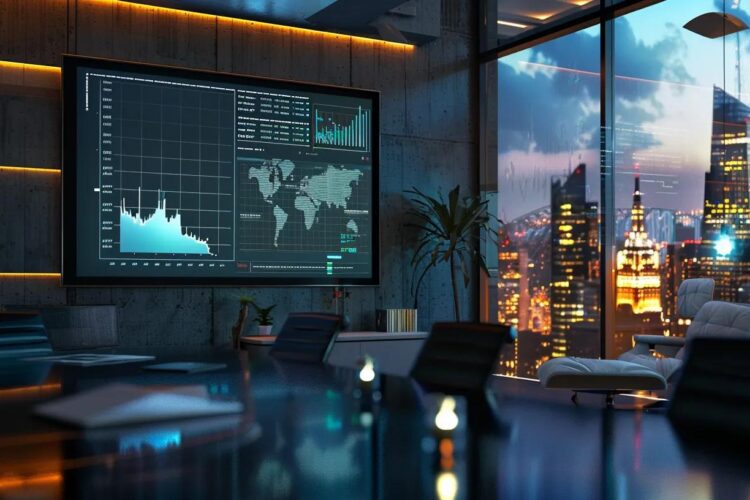
In today’s data-driven economy, accurate sales forecasting is essential for strategic planning, inventory management, and revenue growth. Traditional methods—often reliant on manual inputs and static models—struggle to keep pace with the complexity and speed of modern markets. That’s where artificial intelligence (AI) comes in.
AI-powered forecasting solutions use machine learning algorithms, real-time data processing, and predictive analytics to deliver faster, more reliable sales projections. These technologies reduce human error and uncover hidden patterns in customer behavior, seasonal trends, and market dynamics. As businesses face increasing pressure to make agile, data-informed decisions, AI quickly becomes a foundational tool in the sales leader’s toolkit.
This blog explores how AI is transforming the forecasting landscape—from improving data quality and predictive accuracy to overcoming implementation hurdles and shaping future innovation in sales strategy.
Key Takeaways
- AI enhances sales forecasting accuracy by analyzing large volumes of historical and real-time data using advanced machine learning models.
- Machine learning reduces human bias and error, delivering more consistent and data-driven sales predictions.
- Natural Language Processing (NLP) and real-time validation improve the quality of both structured and unstructured data sources, leading to more reliable forecasts.
- Overcoming adoption challenges requires a clear data strategy, employee training, and the right AI tools tailored to business needs.
- Future advancements in AI forecasting will include deeper integration with IoT devices, real-time automation, and more accessible tools for small and mid-sized businesses.
Understanding How AI Integrates with Sales Forecasting
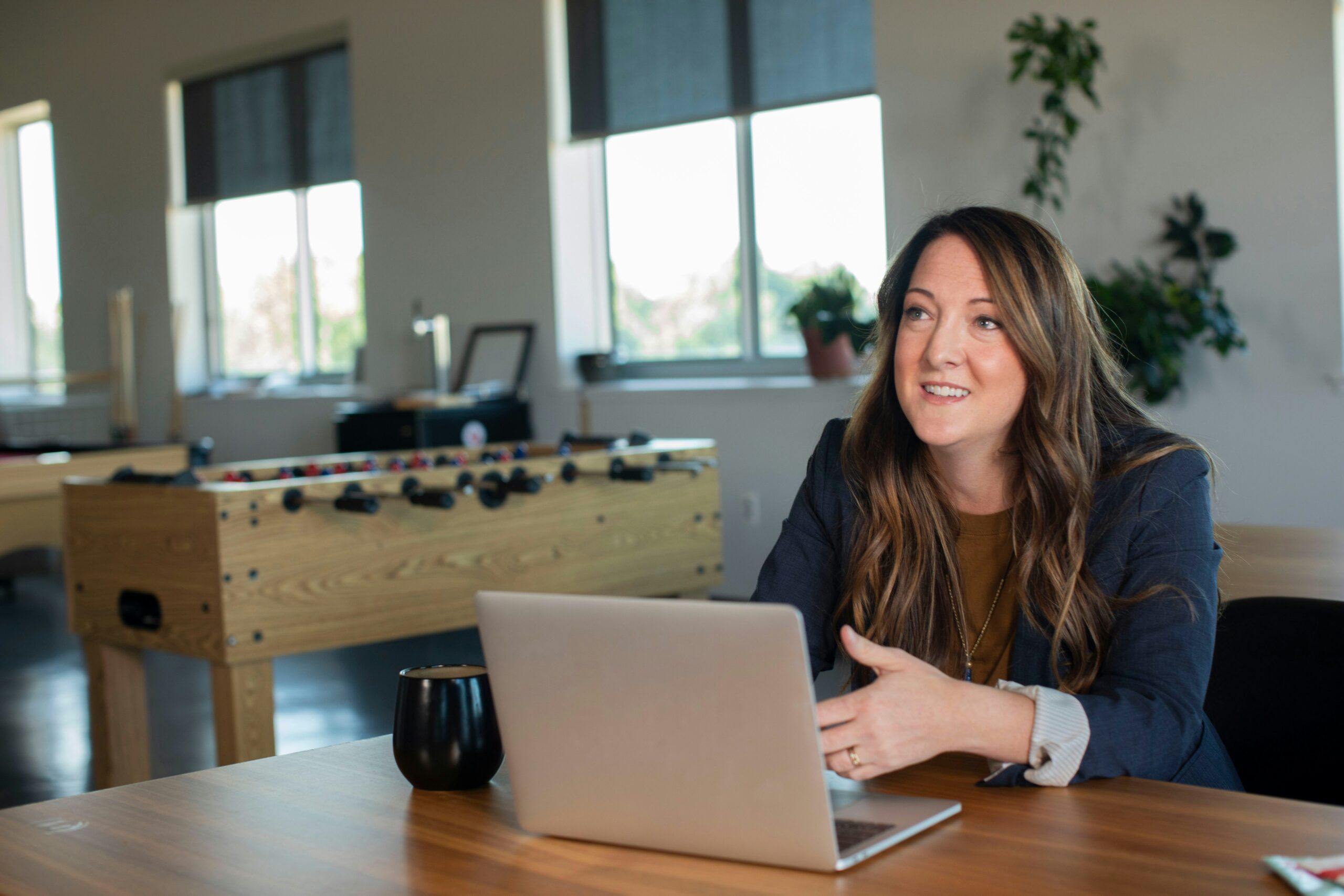
Artificial intelligence transforms how businesses forecast sales by shifting from static, manual processes to dynamic, data-driven models. Traditional forecasting often relies on historical sales averages and human judgment, which can be prone to bias and slow to adapt. AI, by contrast, uses advanced algorithms to process large datasets, identify hidden patterns, and generate more precise and adaptable forecasts. By integrating AI into sales operations, organizations can enhance forecast reliability, respond faster to market changes, and optimize strategic planning.
Machine Learning Models and Historical Data Analysis
At the core of AI-powered sales forecasting are machine learning (ML) models that learn from historical sales data to predict future outcomes. Supervised learning algorithms—such as regression models and decision trees—train on labeled datasets to recognize patterns associated with sales performance over time. Unsupervised learning models cluster and segment data, revealing trends or anomalies that may not be immediately visible to human analysts.
These models can incorporate variables such as seasonality, economic indicators, regional demand differences, and marketing activity, enabling businesses to generate nuanced, context-aware forecasts. By analyzing structured and semi-structured data, AI systems offer deeper insights beyond simple trendlines.
Real-Time Forecasting and Model Adaptation
A significant advantage of AI in sales forecasting is its ability to adapt to new data inputs in real time. As sales transactions, market conditions, or customer behaviors evolve, AI models continuously update predictions based on fresh data. This adaptive learning allows businesses to respond more quickly to shifts in demand, competitive pressure, or supply chain disruptions.
AI-powered real-time forecasting tools integrate seamlessly with CRM platforms, ERP systems, and external data feeds. This connectivity ensures that sales projections remain current and actionable, empowering decision-makers to pivot strategies proactively rather than reactively.
Enhancing Sales Projections with Machine Learning
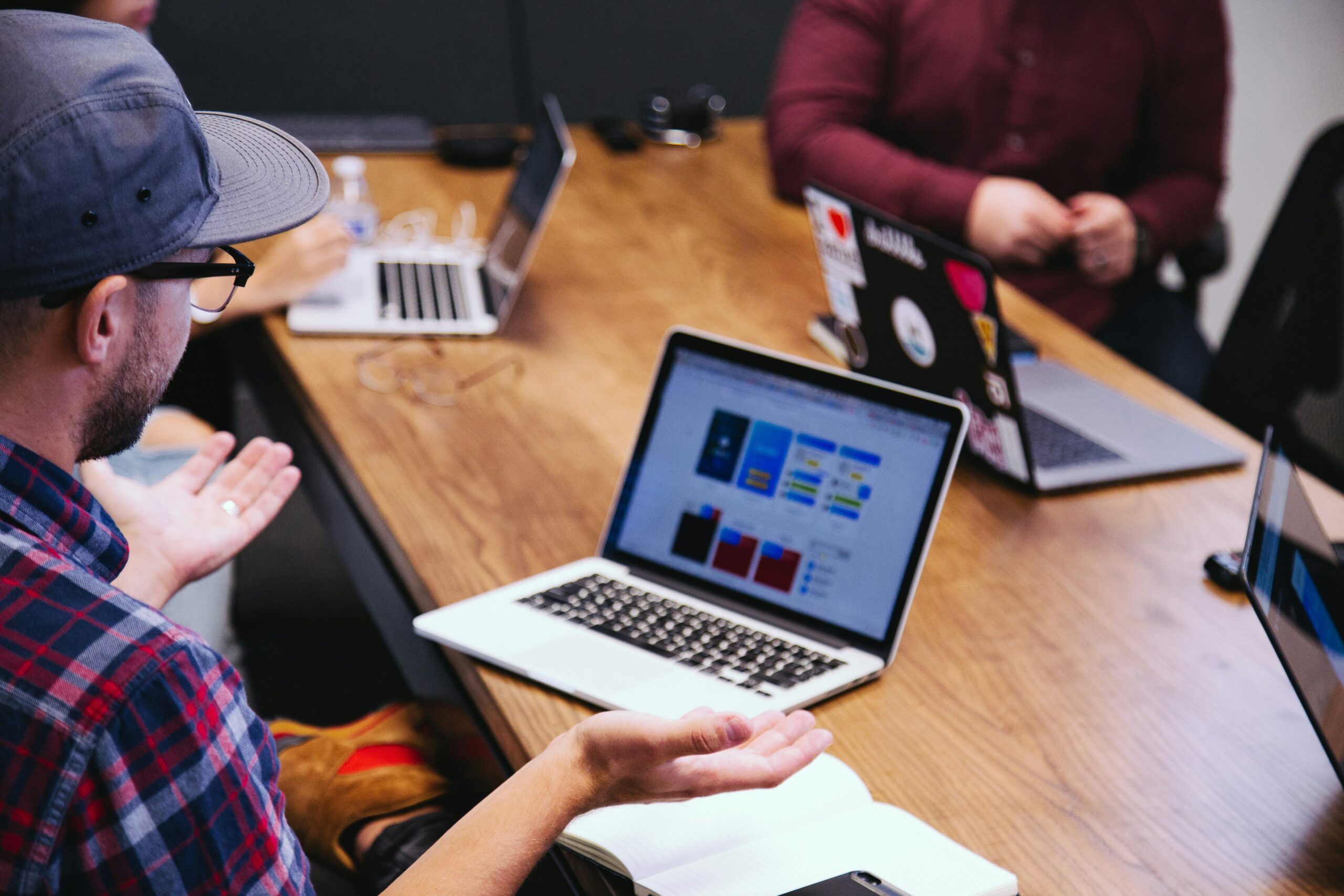
Machine learning (ML) is the engine behind AI’s ability to elevate sales forecasting from a reactive task to a strategic, forward-looking capability. Unlike traditional methods that rely heavily on historical averages and static assumptions, machine learning models can analyze complex variables, detect evolving patterns, and generate more accurate projections. These models support both granular short-term planning and broader long-term strategy by continuously learning and improving from new data inputs.
By leveraging ML, businesses can better align inventory levels, marketing budgets, staffing schedules, and revenue goals with actual market demand—leading to smarter decisions and improved operational efficiency.
Predictive Analytics for Smarter Sales Planning
Machine learning enables predictive analytics by processing massive datasets through algorithms that uncover relationships between sales drivers and outcomes. Tools such as:
- Linear and logistic regression assess how specific variables (e.g., pricing changes, regional promotions) impact sales volume.
- Decision trees segment customers or product categories based on historical performance, making it easier to forecast by segment.
- Neural networks model complex, nonlinear relationships between multiple factors (e.g., seasonality, market sentiment, economic shifts), enhancing long-term projections with greater accuracy.
These models deliver statistically robust, dynamically updated forecasts and can support scenario planning across sales, marketing, and supply chain functions.
Reducing Human Bias and Forecasting Errors
One key advantage of using machine learning in sales forecasting is significantly reducing human bias and manual error. Traditional forecasting methods often depend on subjective judgment or manual data manipulation, which can introduce inconsistencies or skew projections based on overly optimistic or conservative assumptions.
Machine learning automates the data processing and model training stages, ensuring that the output is driven by objective analysis rather than gut feeling. This leads to more consistent and trustworthy forecasts. Additionally, AI-powered systems can flag anomalies, detect outliers, and self-correct over time, minimizing the risk of costly misjudgments.
Ultimately, machine learning empowers businesses to make faster, more confident sales decisions by reducing manual effort and introducing analytical rigor.
Improving Data Quality with AI Technologies
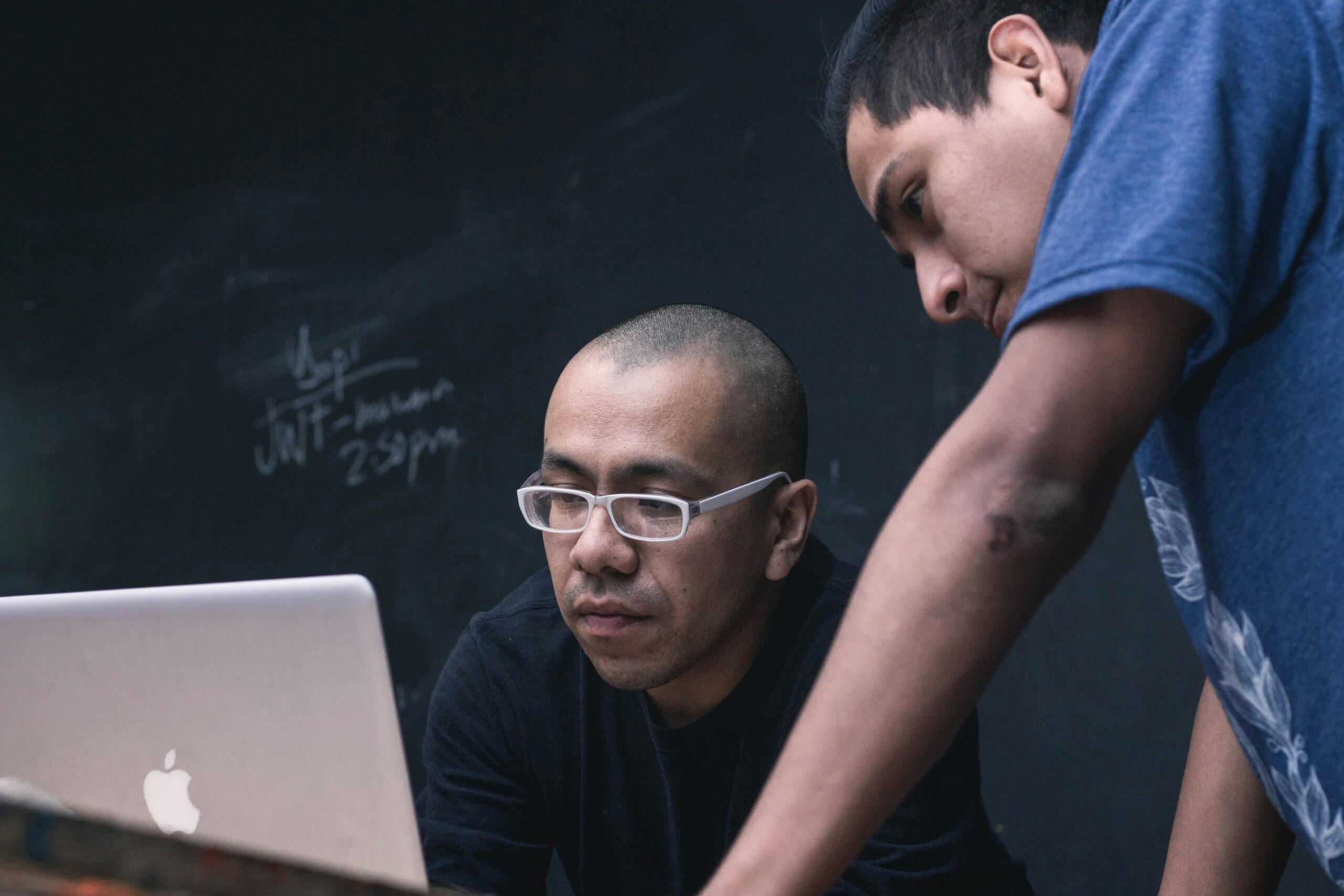
Accurate sales forecasting depends on the quality of the data that feeds into the models. Poor data, whether incomplete, outdated, or inconsistent, can severely compromise forecasting outcomes. AI addresses this challenge by automating data preprocessing, cleansing, and validation at scale. With AI technologies, organizations can ensure their forecasts are built on reliable, high-integrity datasets that reflect real-time market conditions and customer behavior.
From anomaly detection to structured enrichment of unorganized inputs, AI-driven tools elevate the quality and consistency of sales data across every channel.
Data Cleansing, Enrichment, and Anomaly Detection
AI excels at identifying and resolving inconsistencies within datasets through advanced preprocessing techniques. These include:
- Data cleansing involves removing duplicates, correcting formatting errors, and ensuring consistency across data sources.
- Data enrichment, which supplements internal data with external sources—such as demographic insights or market trends—to provide more context for forecasting.
- Anomaly detection, where AI models flag outliers or unexpected patterns (e.g., sudden sales spikes or drops) that could distort forecast accuracy.
These automated processes significantly reduce the manual labor required for data preparation and enhance the predictive power of forecasting models.
Natural Language Processing (NLP) for Unstructured Data
Beyond traditional sales figures and CRM inputs, AI uses Natural Language Processing (NLP) to extract valuable insights from unstructured data sources. This includes:
- Customer reviews
- Support tickets
- Email conversations
- Social media mentions
NLP algorithms convert this qualitative information into structured data points—such as customer sentiment or intent—which can be integrated into forecasting models to predict better buying behavior and market trends. By incorporating the customer’s voice, businesses can build forecasts that are not only quantitative but also behaviorally informed.
Real-Time Validation and Continuous Feedback Loops
AI systems integrated with cloud infrastructure and CRM platforms enable continuous, real-time validation of incoming data. AI tools instantly verify and update records as new transactions, customer interactions, and marketing campaign results flow in. This:
- Ensures consistency across data sources
- Eliminates time lags in data reporting
- Creates a feedback loop where forecasting models become more accurate with each iteration
This level of responsiveness ensures that sales forecasts are always based on the most current and credible data available—boosting executive confidence and supporting agile decision-making.
Overcoming Implementation Challenges in AI Sales Forecasting
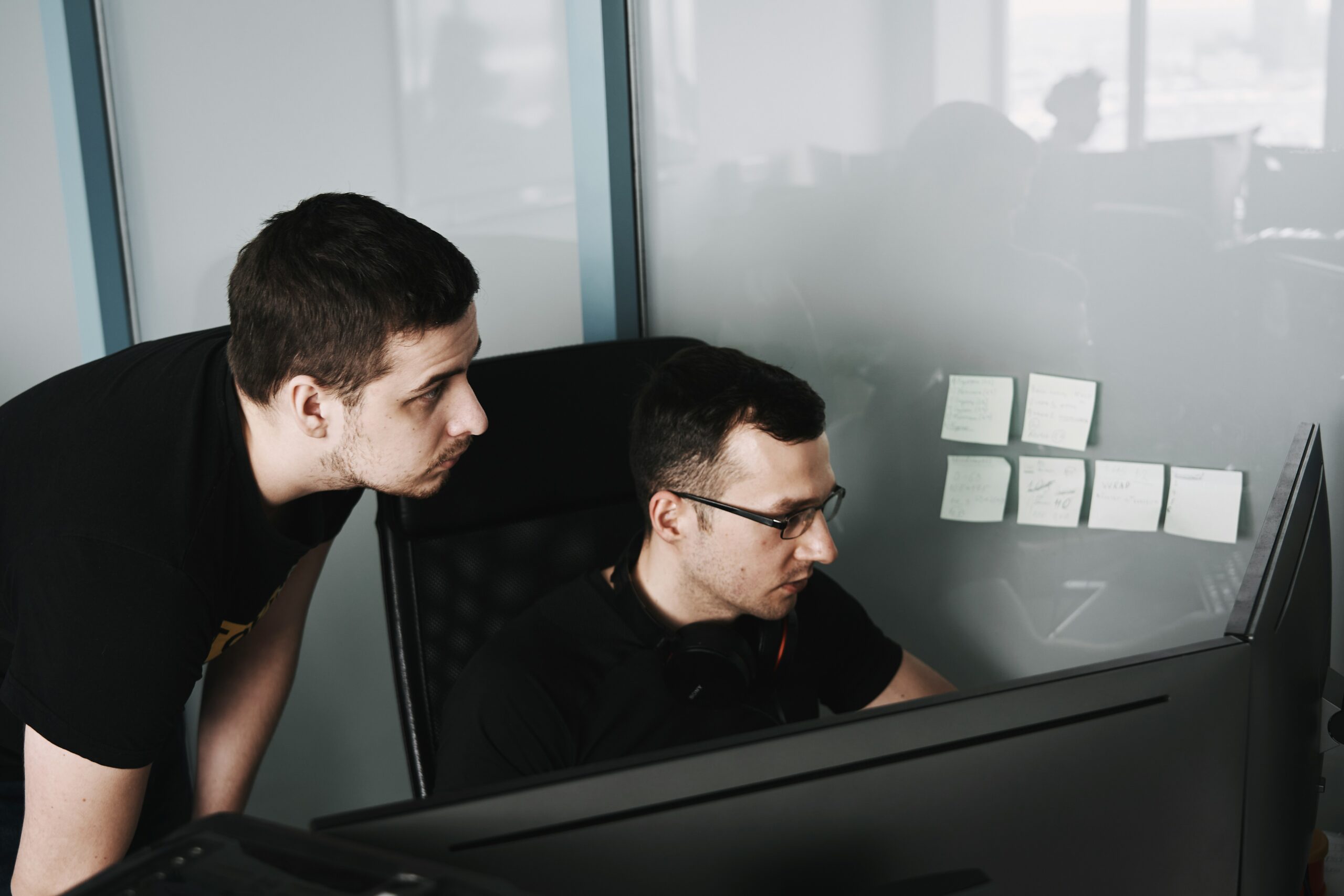
While AI offers significant benefits in sales forecasting, implementing these technologies isn’t without hurdles. Many organizations struggle with fragmented data systems, resistance from internal teams, and uncertainty around selecting the right AI models. These obstacles can stall or undermine the impact of AI initiatives if not addressed strategically.
However, with the right combination of infrastructure, education, and phased adoption, businesses can overcome these challenges and unlock the full potential of AI-driven forecasting.
Building a Robust Data Strategy
A successful AI forecasting initiative begins with clean, centralized, and accessible data. Many organizations operate with siloed systems—CRM platforms, marketing tools, inventory databases—that do not communicate effectively.
To address this, businesses should:
- Implement data integration frameworks that unify data from various departments.
- Use cloud-based platforms to centralize storage and enable real-time access.
- Standardize data collection protocols to ensure consistency across inputs.
Establishing a solid data foundation reduces friction during AI implementation and increases forecast accuracy from the start.
Driving Organizational Buy-In Through Training
Internal resistance is a common barrier, especially when teams are accustomed to traditional forecasting tools or manual spreadsheets. Employees may be hesitant to trust automated models or feel unsure about how AI will impact their roles.
Overcoming this requires:
- Ongoing training programs that explain the value of AI in sales forecasting and how it complements, rather than replaces, human expertise.
- Pilot programs that demonstrate early wins and allow teams to interact with AI tools in a controlled environment.
- Leadership involvement to reinforce the strategic importance of data-driven forecasting across departments.
Education and transparency are key to building trust and driving adoption.
Selecting the Right Forecasting Models
Choosing the wrong AI model can lead to inaccurate predictions and wasted resources. The right model should align with the company’s sales cycles, data complexity, and market volatility.
For example:
- Regression models work well for stable markets with predictable patterns.
- Neural networks and ensemble models are better suited for dynamic environments with multiple influencing variables.
- Time-series forecasting tools are ideal for businesses with seasonality and trend-based fluctuations.
Running test models in parallel and measuring their performance allows companies to identify the best fit before scaling.
Addressing Compliance and Data Privacy Concerns
AI tools process vast amounts of sensitive customer and business data, which makes regulatory compliance a top priority. Ignoring privacy regulations can result in reputational damage and financial penalties.
To stay compliant, organizations should:
- Follow standards like GDPR or CCPA based on their geographic and industry obligations.
- Use data encryption, access controls, and audit trails to secure information.
- Appoint a compliance officer or data governance lead to monitor ongoing adherence.
Prioritizing privacy and transparency protects the business and builds trust with customers and stakeholders.
The Future of AI in Sales Forecasting
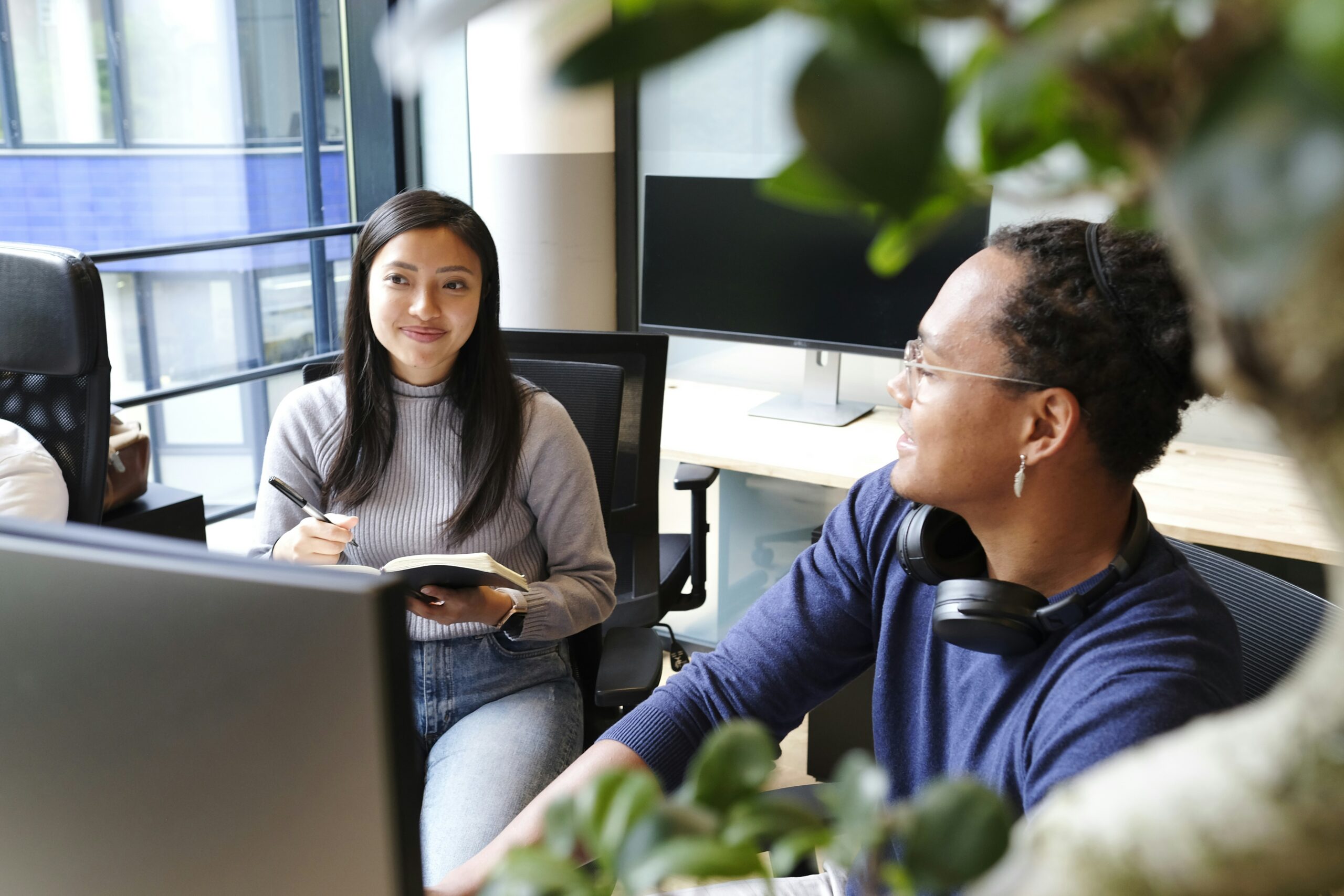
As AI technology evolves, its role in sales forecasting is expected to grow deeper, brighter, and more integrated. Tomorrow’s forecasting tools won’t just predict what might happen—they’ll help businesses understand why it’s happening and what to do next. Innovations in deep learning, automation, and cross-platform integration will make forecasting faster, more personalized, and actionable at every level of an organization.
The future of AI in this space lies in enhancing accuracy, accessibility, and real-time responsiveness, driving a shift from passive reporting to proactive sales intelligence.
Deep Learning and Multi-Variable Forecasting
Traditional models are effective with structured datasets, but they often struggle with complex, nonlinear relationships between variables. That’s where deep learning comes in. These models can analyze diverse data streams—like macroeconomic shifts, promotional calendars, and even customer sentiment—in parallel, uncovering subtle correlations that improve forecast precision.
Future applications will use these models to:
- Account for volatile market behavior
- Incorporate third-party data like competitor pricing or weather patterns
- Predict long-term customer value and lifecycle trends
This leap in complexity will allow businesses to forecast with greater nuance and strategic depth.
IoT Integration for Real-Time Data Streams
The rise of the Internet of Things (IoT) is expanding the data available for forecasting. Sensors, connected devices, and smart retail systems will feed real-time data directly into AI models.
For example:
- POS systems will instantly transmit sales performance
- Smart shelves and scanners will monitor inventory in real time
- Customer traffic sensors in physical stores will inform demand patterns
By integrating these live data feeds, AI forecasting tools can provide up-to-the-minute projections that reflect actual market conditions—not just historical trends.
AI-Powered Dashboards and Automation Features
Future AI forecasting platforms will prioritize usability through intuitive dashboards that display real-time forecasts, anomaly alerts, and trend visualizations. These tools will help non-technical users interpret complex models and take swift action.
More importantly, forecast automation will go beyond suggestions. It will trigger:
- Replenishment orders
- Sales strategy adjustments
- Dynamic resource allocation
This shift from analytics to autonomous action will allow organizations to respond immediately to forecast changes—minimizing risk and maximizing opportunity.
Democratizing Forecasting for Small and Mid-Sized Businesses
As AI solutions become more affordable and user-friendly, small and mid-sized businesses (SMBs) will gain access to tools once reserved for large enterprises. No longer limited by budget or in-house data science teams, these companies can now use AI to:
- Improve cash flow predictability
- Align staffing with seasonal trends
- Respond faster to market shifts
The democratization of AI-driven forecasting will level the competitive playing field and drive innovation across all sectors.
Frequently Asked Questions
How does AI improve sales forecasting accuracy?
AI analyzes large volumes of historical and real-time data to identify patterns and trends. Machine learning models automatically adjust to new inputs, leading to significantly more accurate forecasts than those generated using traditional methods.
What types of AI models are commonly used in forecasting?
Common models include linear regression, logistic regression, decision trees, and neural networks. Each serves different forecasting needs—ranging from stable, repeatable patterns to more dynamic, behavior-driven predictions.
Can AI handle unstructured data like customer reviews or emails?
Yes. Through Natural Language Processing (NLP), AI can extract insights from unstructured data sources such as customer feedback, emails, and social media. These insights are converted into structured variables that enhance forecast quality.
What are the key challenges in implementing AI for sales forecasting?
Significant challenges include poor data integration, lack of internal expertise, employee resistance, and data privacy compliance. A strategic rollout plan that provides for training, infrastructure investment, and regulatory alignment is essential for success.
Is AI forecasting suitable for small businesses?
Absolutely. Many modern AI forecasting tools are cloud-based, scalable, and cost-effective, making them accessible to small and midsize businesses (SMBs). These tools can help SMBs plan more accurately, reduce waste, and respond quickly to market changes.
Conclusion
Artificial intelligence redefines how businesses forecast sales by replacing static spreadsheets and guesswork with adaptive, data-driven precision. By integrating machine learning models, real-time analytics, and high-quality data inputs, AI enables companies to make smarter decisions, faster. Whether identifying sales trends, minimizing stockouts, or optimizing resource allocation, AI-powered forecasting offers a strategic advantage in today’s competitive market.
Although challenges like data integration and team adoption still exist, organizations that invest in the proper infrastructure and training can overcome these barriers. With advancements in deep learning, IoT integration, and automation on the horizon, AI will continue to elevate forecasting from a back-office task to a proactive business intelligence function.
Comments