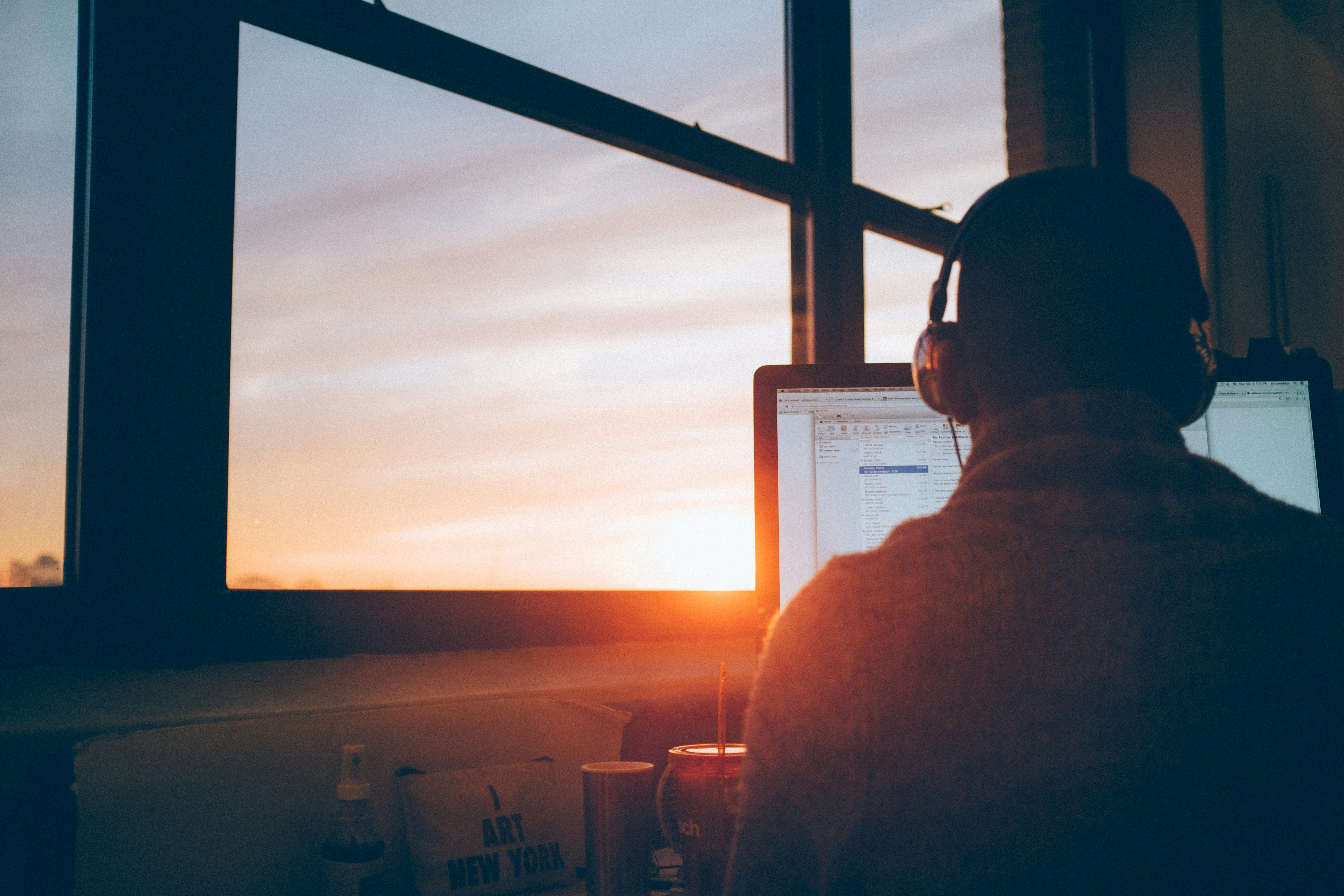
Predictive analytics is transforming the way advertisers approach campaign planning and audience engagement. Predictive models enable marketers to anticipate consumer actions and tailor strategies by analyzing historical data and identifying behavioral patterns. These models leverage machine learning and statistical techniques to forecast outcomes such as click-through rates, conversions, and customer retention, allowing for smarter decisions and more efficient ad spend.
In today’s data-driven advertising landscape, success depends on creative messaging, precise targeting, and budget optimization. Predictive analytics offers the tools to deliver both. This blog explores how predictive models work in advertising, the methods commonly used, the benefits they offer, and what future innovations are on the horizon. Whether you’re optimizing paid media or planning long-term campaigns, understanding predictive analytics is essential for staying competitive and maximizing return on investment.
Key Takeaways
- Predictive analytics uses historical and real-time data to anticipate advertising outcomes such as conversions, churn, and engagement.
- Machine learning models like regression, decision trees, and neural networks help advertisers identify high-performing segments and optimize campaign strategies.
- These models support smarter budget allocation by focusing spend on channels and audiences most likely to convert.
- Predictive methods enable personalized advertising by tailoring content based on user behavior and preferences.
- As the field evolves, advancements in AI, real-time data processing, and privacy-compliant modeling are shaping the future of predictive advertising.
Understanding Predictive Analytics in Advertising
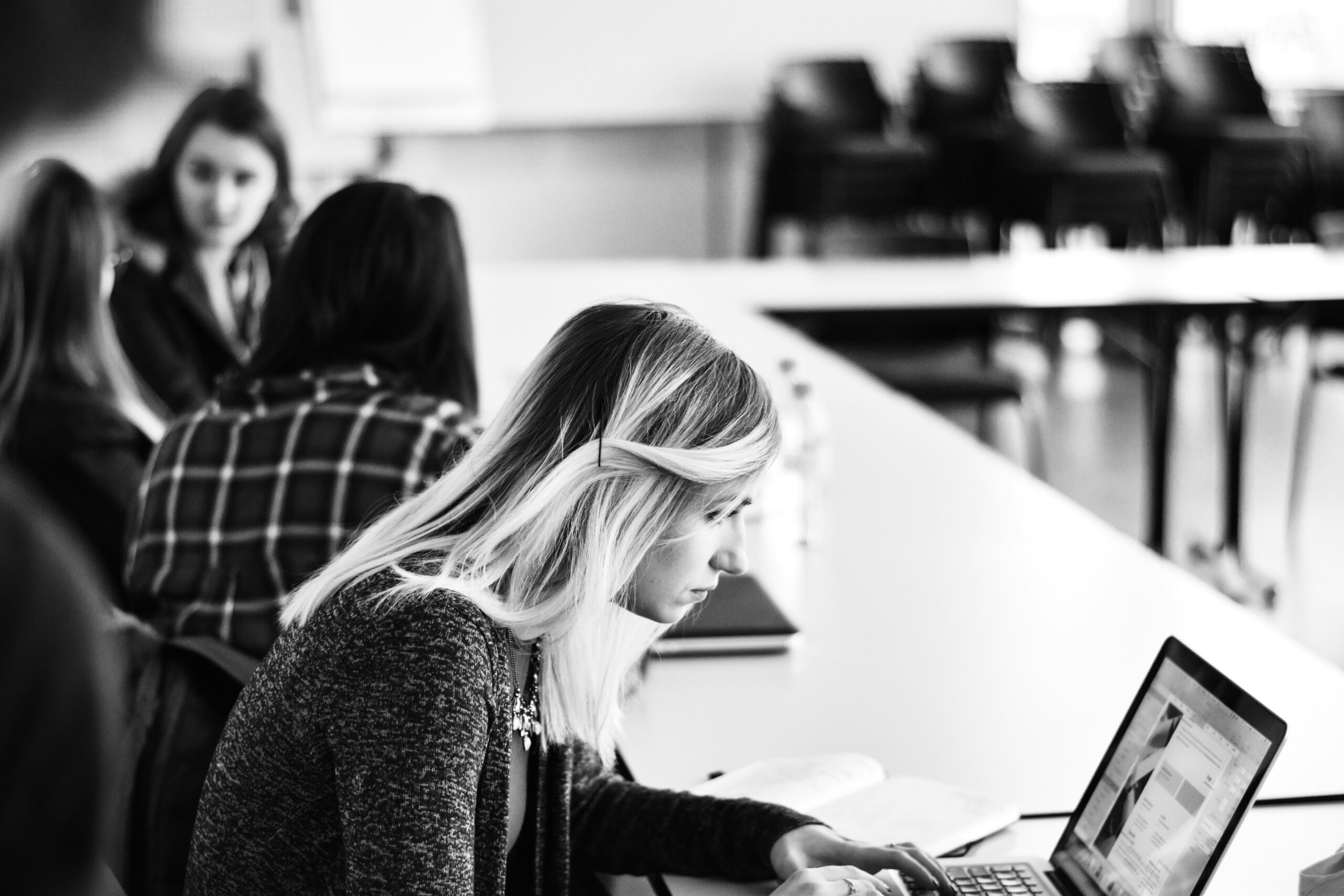
Predictive analytics enables advertisers to make informed decisions by forecasting future outcomes based on historical data. Instead of relying solely on intuition or broad demographic assumptions, advertisers can now analyze large datasets to detect meaningful patterns in user behavior, media interaction, and conversion performance. These insights allow for more accurate targeting, better timing, and more efficient campaign execution.
What Is Predictive Analytics?
Predictive analytics refers to the use of statistical algorithms and machine learning techniques to identify the likelihood of future events. In the advertising space, it draws on past performance data—such as clicks, impressions, customer purchases, and browsing behavior—to predict how users are likely to respond to future campaigns. This proactive approach enables brands to shift from reactive to anticipatory marketing strategies.
How It Applies to Advertising
In advertising, predictive models serve as decision-support tools. They analyze customer journeys, segment audiences, and estimate metrics like click-through rates (CTR), cost per acquisition (CPA), and conversion probability. For instance, a model might determine that users who visit a pricing page twice in one week are 3x more likely to convert—allowing advertisers to retarget them with tailored messaging or special offers. With this level of insight, campaigns become more personalized and impactful.
Key Benefits of Predictive Analytics for Marketers
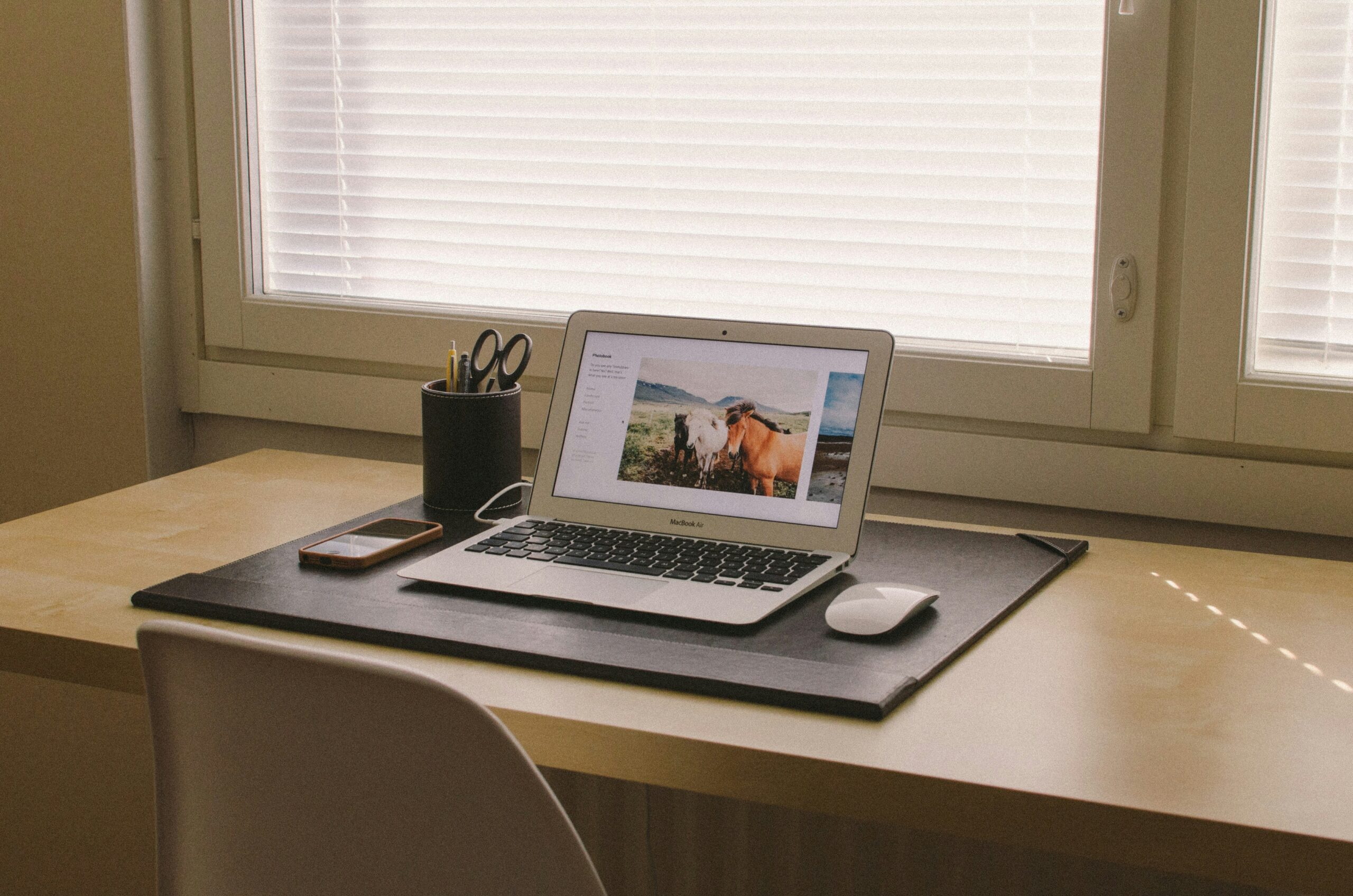
Predictive analytics isn’t just a buzzword—it’s a foundational tool that helps marketers streamline decision-making, reduce wasted spend, and create more relevant customer experiences. By combining historical data with advanced algorithms, marketers can better understand audience behavior, campaign performance, and future outcomes. These insights translate directly into higher return on investment and stronger competitive positioning.
Enhanced Campaign Efficiency
One of the most significant advantages of predictive analytics is improving efficiency across the entire advertising workflow. Marketers can allocate resources more strategically by forecasting which campaigns or audience segments are most likely to perform well. Instead of spreading budget thinly across multiple channels, predictive models allow teams to double down on high-value opportunities, resulting in lower acquisition costs and increased marketing ROI.
Improved Audience Segmentation
Traditional segmentation methods rely heavily on surface-level data like age, gender, or location. Predictive analytics goes further by uncovering behavioral patterns, purchase intent, and user engagement signals. Marketers can build more dynamic and actionable audience segments, enabling them to serve personalized ads that speak directly to each group’s interests and readiness to convert.
Lower Cost Per Acquisition (CPA)
When marketers understand who is most likely to take action, they can minimize spend on unqualified audiences. Predictive models help identify high-converting user profiles and optimize bidding strategies accordingly. As a result, brands often experience a measurable drop in CPA and an overall boost in return on ad spend (ROAS).
Risk Mitigation and Early Problem Detection
Predictive analytics isn’t just about boosting performance—it’s also a powerful tool for identifying risk. Whether it’s spotting early signs of campaign fatigue, customer churn, or budget inefficiency, these models allow marketers to address issues before they escalate proactively. With timely alerts and predictive diagnostics, marketing teams can pivot quickly and stay ahead of performance dips.
Building and Training Predictive Models
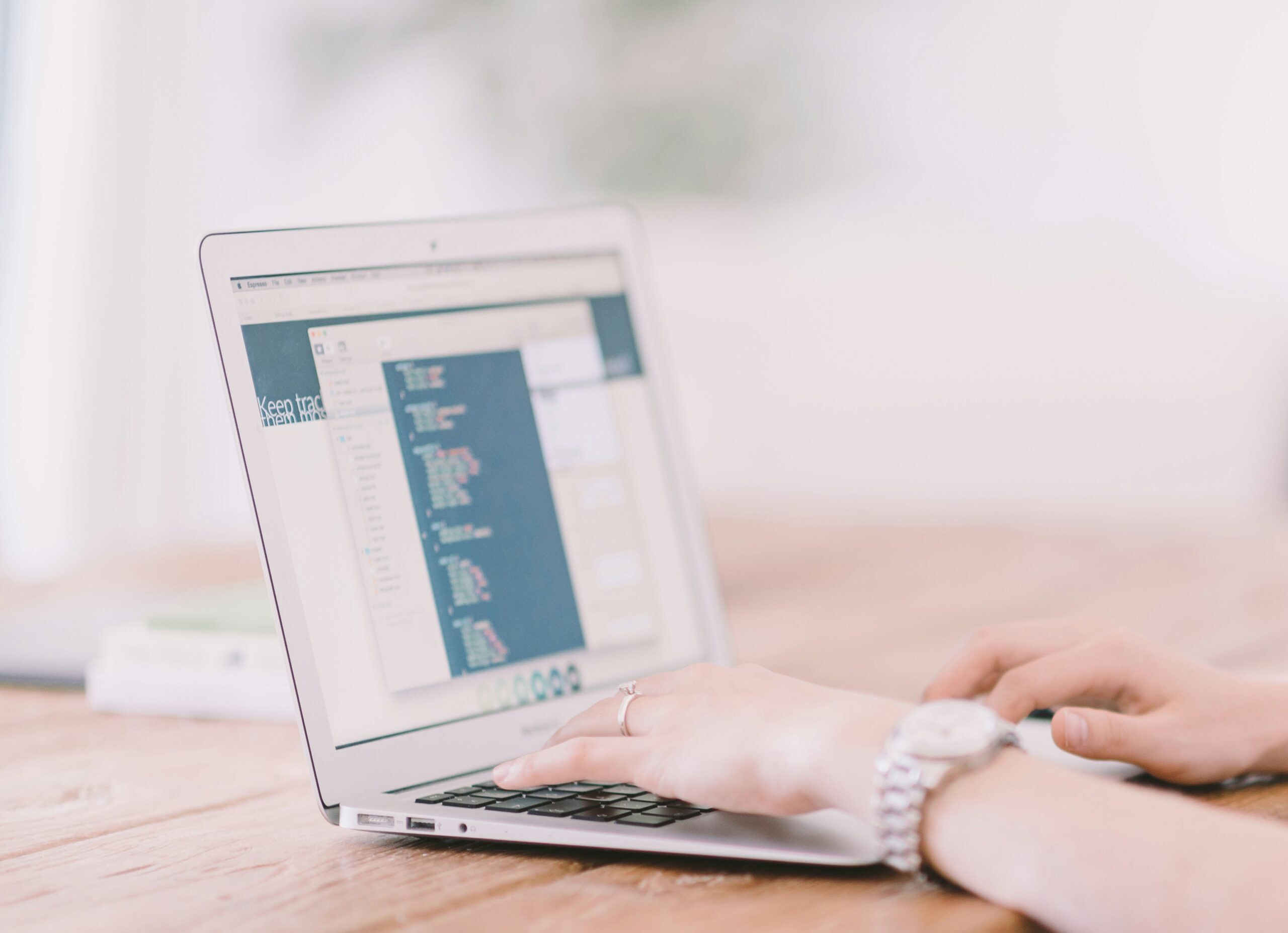
Developing effective predictive analytics models for advertising requires a strategic combination of quality data, the right algorithms, and rigorous testing. Each phase of the model-building process plays a critical role in determining how well the predictions align with real-world outcomes. When executed properly, these models become valuable tools for campaign planning, performance forecasting, and audience targeting.
Data Collection and Feature Engineering
The foundation of any predictive model lies in its data. Marketers must collect both structured and unstructured data from a wide range of sources, including CRM systems, website analytics, ad platforms, and social media channels. Once gathered, feature engineering must transform this raw data into meaningful inputs.
Feature engineering involves identifying variables (features) that influence consumer behavior, such as time on site, frequency of purchases, or engagement with previous ads. Additional steps like data cleaning, normalization, and outlier detection ensure that the dataset is accurate, consistent, and ready for modeling.
Model Selection and Algorithm Training
Choosing the right model depends on the specific advertising objective. For example:
- Linear regression is often used to forecast ad spend impact on conversions.
- Random forest and gradient boosting are great for classification tasks like predicting churn or conversion likelihood.
- ARIMA models are valuable for time-series forecasting, such as projecting seasonal ad demand.
During training, the model analyzes historical data to learn the relationship between features and outcomes. It then adjusts its internal parameters to minimize prediction error—essentially “learning” from past behavior to make future predictions more accurate.
Validation and Overfitting Prevention
After training, it’s essential to test the model on new, unseen data. This validation step determines whether the model performs well outside its training environment. Techniques like cross-validation help ensure that the model generalizes to various campaign scenarios. Marketers must also watch for overfitting—a condition where the model becomes too tailored to the training data and loses effectiveness on new inputs. To mitigate this risk, strategies such as regularization, early stopping, and ensemble methods can be applied to enhance robustness.
Popular Predictive Analytics Methods in Advertising
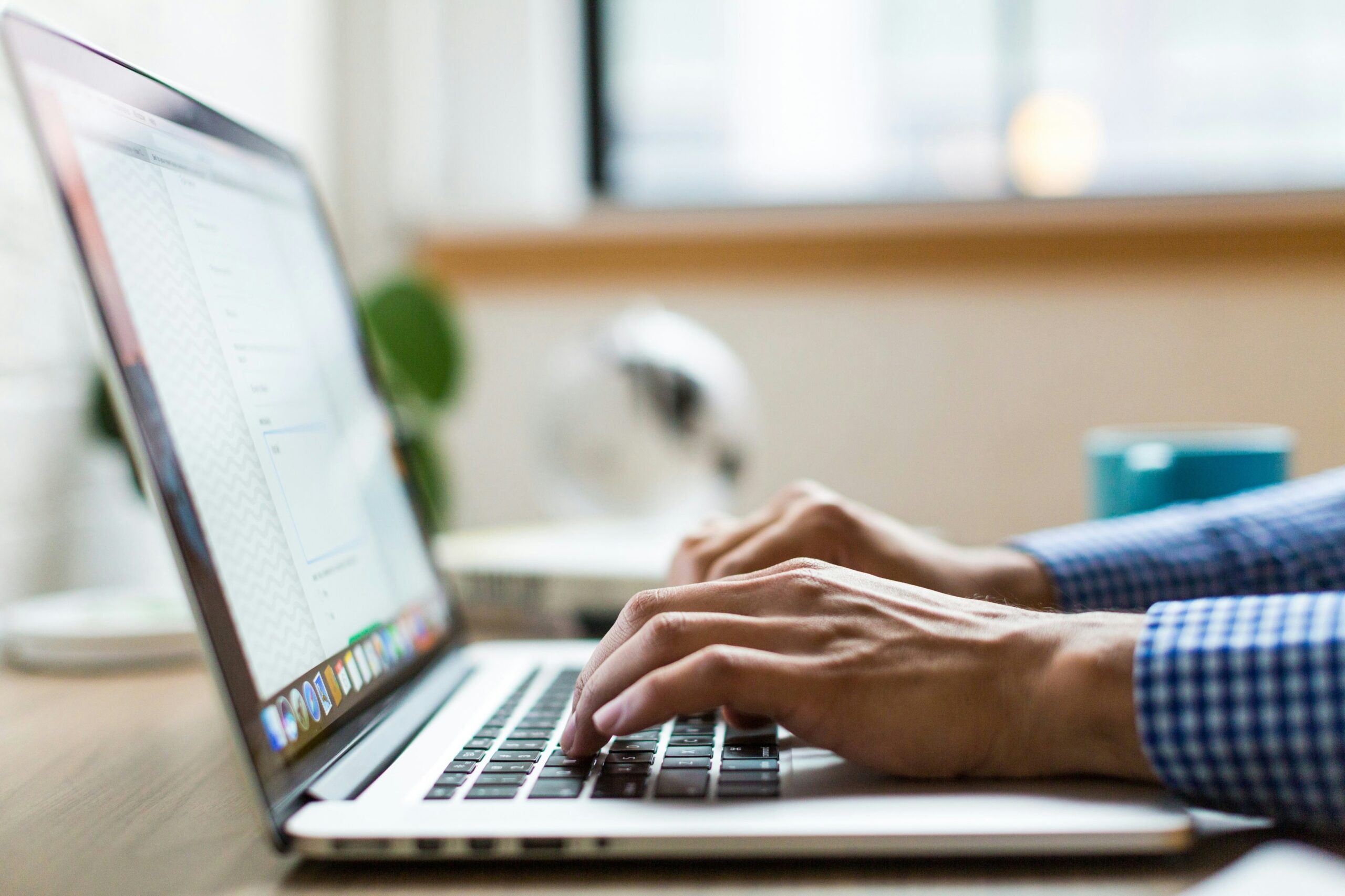
Predictive analytics isn’t a one-size-fits-all approach. Different advertising goals require different modeling techniques—some better suited for forecasting trends, others for segmenting audiences or recommending content. Understanding the strengths of each method helps marketers choose the right tool for their specific campaign objectives.
Linear Regression for Trend Forecasting
Linear regression is a fundamental technique used to identify relationships between variables. In advertising, it’s commonly applied to assess how changes in ad spend influence performance metrics like conversions, revenue, or click-through rates (CTR). Because of its simplicity and interpretability, regression is ideal for high-level budget planning and ROI forecasting.
Decision Trees and Random Forests for Audience Classification
Decision trees break down complex decisions into a series of binary choices—making them easy to understand and implement. Random forests build on this by combining multiple decision trees to improve accuracy and reduce bias. These models are particularly effective in classifying audiences based on behavior, engagement, or conversion likelihood, helping marketers tailor content and targeting strategies.
Neural Networks for Complex Data Patterns
Neural networks are powerful tools for recognizing patterns in large, unstructured datasets. They are especially useful when working with visual content, audio, or intricate user interactions—like product recommendations on e-commerce platforms. In advertising, neural networks are increasingly used for dynamic creative optimization and real-time personalization.
Time Series Forecasting (ARIMA) for Seasonal Campaigns
Autoregressive Integrated Moving Average (ARIMA) models are ideal for predicting future values based on past trends and seasonal cycles. Marketers use these models to forecast peaks in ad engagement or demand during specific times of year—such as holidays or sales events—allowing for proactive budget and inventory planning.
Collaborative Filtering for Content and Offer Personalization
Collaborative filtering analyzes similarities in user behavior across audiences to recommend products, services, or content. It’s commonly used in advertising platforms to serve personalized ads or offers based on what similar users have engaged with. This method is a cornerstone of programmatic ad targeting and content recommendation engines.
Future Trends in Predictive Analytics for Advertising
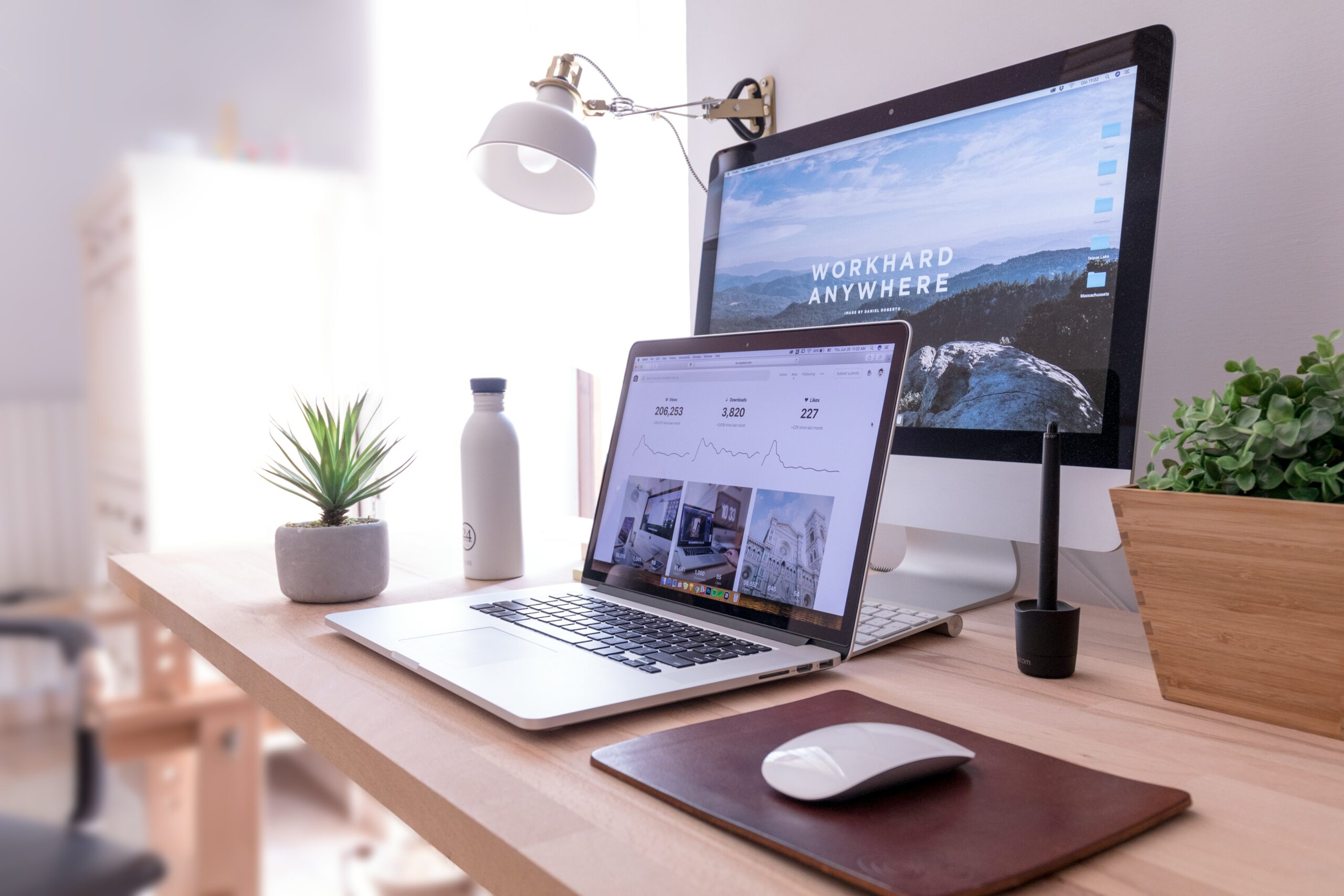
As marketing technology evolves, predictive analytics becomes more intelligent, responsive, and privacy-aware. Future developments will center on deepening automation, enhancing real-time data processing, and building consumer trust through ethical data use. Advertisers who embrace these innovations will be better positioned to scale results while adapting to a changing digital landscape.
AI-Powered Campaign Automation
Advancements in artificial intelligence are pushing predictive analytics toward greater autonomy. Deep learning models are being integrated into ad platforms to automatically adjust bids, reallocate budget, and personalize content without manual input. This level of automation enables advertisers to respond instantly to shifting consumer behaviors and market dynamics.
Real-Time, Multi-Channel Optimization
Analyzing and acting on data in real time rapidly becomes a competitive advantage. Future predictive systems will integrate seamlessly across platforms—search, social, mobile, and OTT—allowing marketers to optimize campaigns holistically. This cross-channel visibility helps ensure messaging consistency and maximizes engagement across the customer journey.
Improved Processing of Unstructured Data
With more content formats emerging—images, voice, video—future models will be better equipped to process unstructured data sources. This will open the door for more personalized creative delivery, using context cues and sentiment analysis to inform ad variations based on media type or user tone.
Data Ethics and Privacy-First Modeling
As regulations like GDPR and CCPA reshape data collection practices, predictive analytics must evolve to protect user privacy. Techniques such as differential privacy, federated learning, and anonymized modeling will allow advertisers to maintain predictive power without compromising compliance or consumer trust.
Prescriptive Analytics for Actionable Insights
The next step in the analytics evolution is prescriptive analytics—systems that not only predict outcomes but also recommend the best next move. These models will help advertisers make smarter decisions about which creatives to run, which audiences to pursue, and how to allocate budgets based on dynamic forecasts.
Frequently Asked Questions
What is the role of predictive analytics in advertising?
Predictive analytics uses historical and real-time data to forecast campaign outcomes like conversions, engagement, and customer behavior. It helps marketers make informed decisions about targeting, timing, and budget allocation.
Which models are most effective for personalized advertising?
Neural networks and collaborative filtering models are highly effective for personalizing ads. They analyze user preferences and behavior to recommend relevant content or offers.
How quickly can predictive models impact campaign performance?
If the data is high-quality and the model is well-trained, marketers can see improvements—such as increased conversion rates or lower CPA—within a few weeks to a few months of implementation.
What’s the difference between predictive and prescriptive analytics?
Predictive analytics forecasts what is likely to happen (e.g., user churn), while prescriptive analytics goes a step further by recommending actions to improve outcomes (e.g., send a retention offer).
Are predictive analytics tools compliant with privacy regulations?
Yes—many modern predictive tools now incorporate privacy-preserving methods like anonymization, differential privacy, or federated learning to comply with laws such as GDPR and CCPA while maintaining model performance.
Conclusion
Predictive analytics is more than just a data science buzzword—it’s a strategic capability that empowers advertisers to make smarter, faster, and more efficient decisions. By using historical and real-time data to anticipate user behavior, marketers can improve campaign precision, personalize messaging, and reduce wasted spend. Predictive models provide a foundation for performance-driven growth, whether you’re running display ads, planning a product launch, or scaling an e-commerce strategy.
As marketing technology evolves, so will the tools used to forecast outcomes and drive engagement. Embracing predictive analytics today means building a future-ready advertising strategy that can adapt to shifting market trends, emerging platforms, and growing consumer expectations. The key is to start with clean data, choose the right model for the goal, and continuously refine predictions with real-world feedback.
Comments