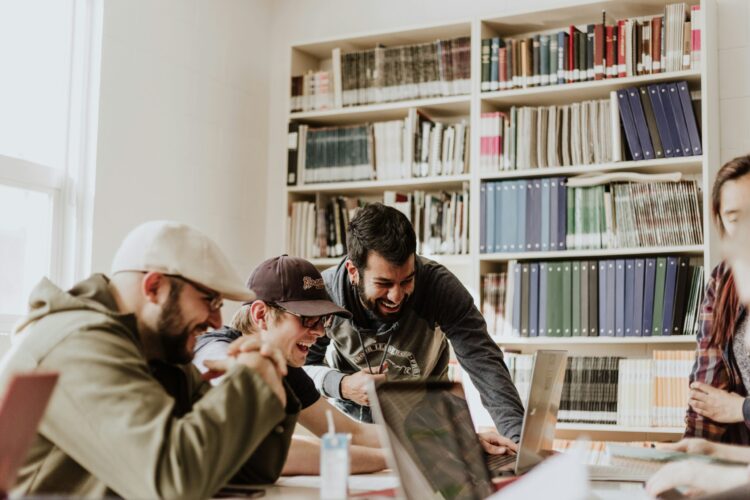
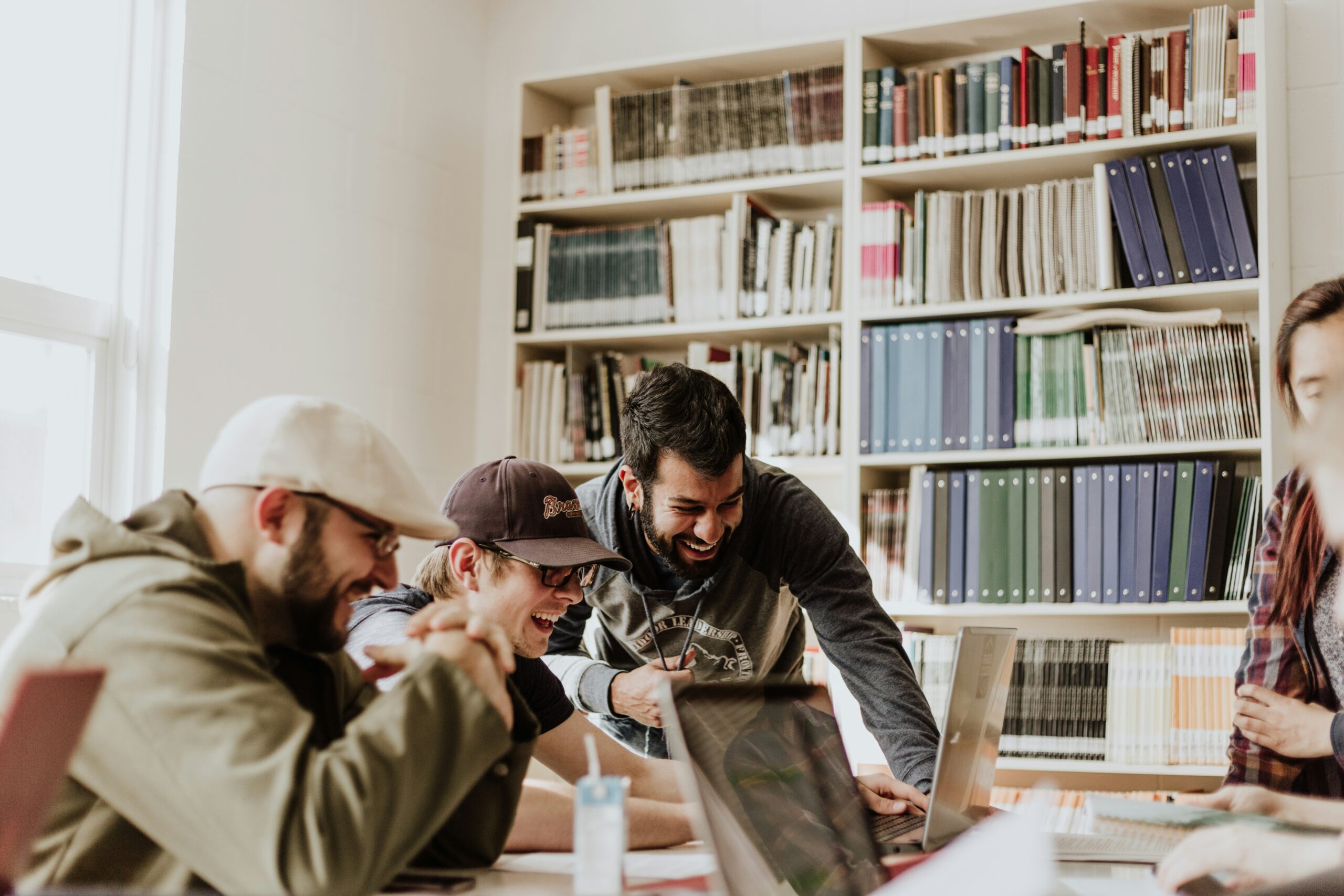
Mastering AI Marketing: Harnessing the Power of Predictive Analytics Tools
The marketing landscape is evolving consistently, as AI and predictive analytics tools take center stage in the art of understanding fiscal forecasts and consumer trends.
By tapping into the world of big data, savvy marketers can anticipate demand, refine targeting, and enhance campaign traction with remarkable precision.
These cutting-edge tools not only predict future behaviors but also empower businesses to make data-backed decisions that position them steps ahead of the competition.
From adjusting supply chains to tailoring user experiences, the mastery of predictive analytics is integral in constructing a powerful marketing system.
Keep reading to decode the methodology that will transform your organization’s marketing efficiency and effectiveness.
Key Takeaways
- Predictive Analytics in AI Marketing Enables Precise Planning in Targeting and Engaging Customers
- Strategic Use of Predictive Models and Data Analysis Boosts Marketing Efficiency and ROI
- Choosing the Right Predictive Analytics Tools Is Critical for Seamless Integration and Marketing Effectiveness
- The Blend of Automation and Human Insight Is Key to Effectively Utilizing Predictive Analytics for AI Marketing
- Ethical Considerations, Including Privacy and Bias, Are Vital in the Responsible Use of Predictive Analytics
Understanding Predictive Analytics in AI Marketing
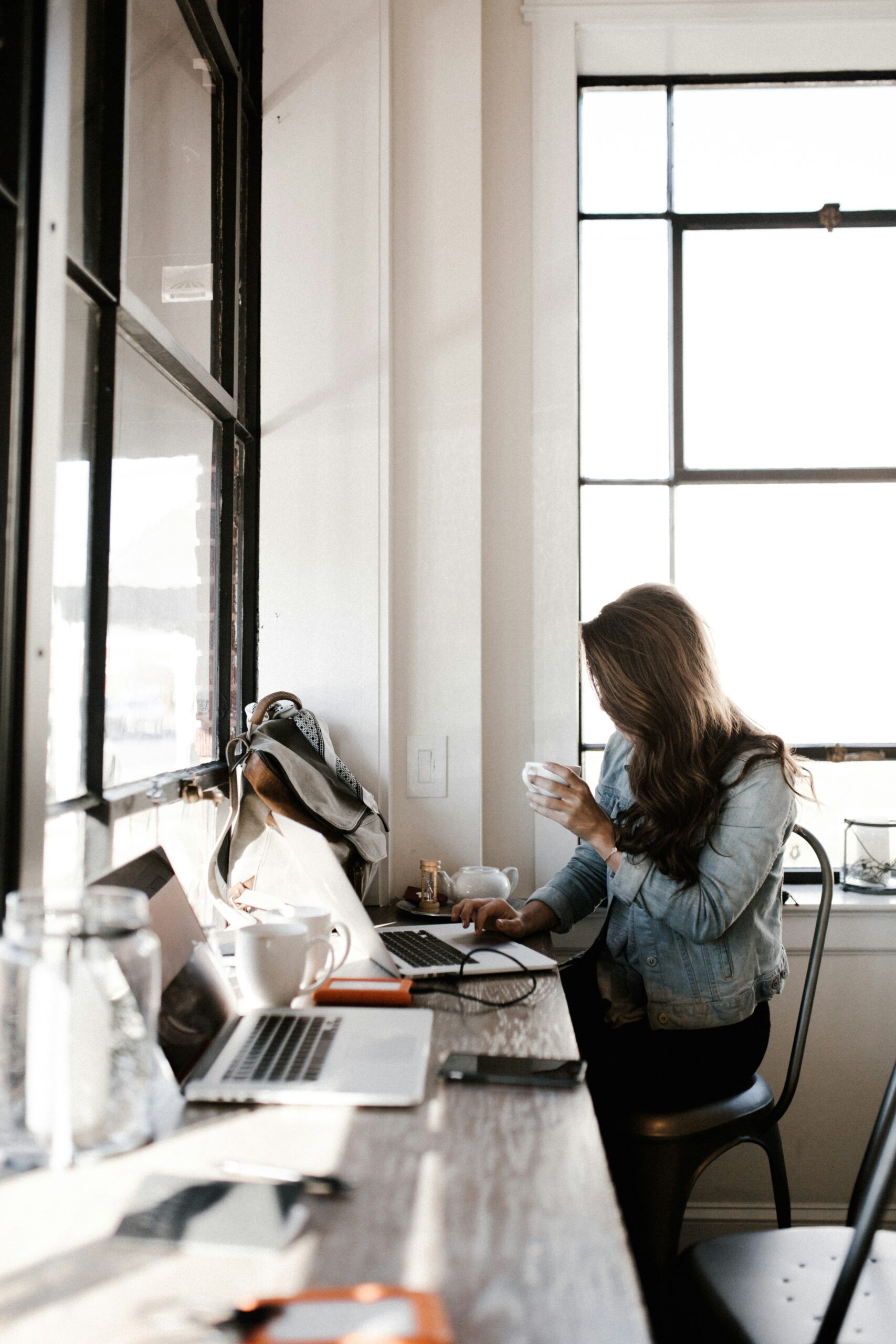
The convergence of artificial intelligence and predictive analytics is revolutionizing how businesses foresee consumer behavior and craft their strategies accordingly.
As organizations uncover the value of predictive models—spurred by the capabilities of algorithms for predictive analysis—they are empowered to calibrate their marketing efforts with unparalleled precision.
This combination guides marketers in deciphering the intricate patterns of demand, anticipates market shifts and tailors experiences to engage the target audience effectively.
Exploring the Basics of Predictive Analytics
Predictive analytics stands as a pillar of modern AI and marketing, harnessing past and present data to foretell future events with statistical models and machine learning. By analyzing patterns within large data sets—be it from social media, customer transactions, or market intelligence—these tools enable a proactive approach, crafting marketing strategies that address upcoming trends and consumer needs.
This methodology is not just about plotting data points on a chart; it’s about transforming raw data into a compass for decision-making that can steer the supply chain, personalization efforts, and inventory management with foresight and precision.
How Predictive Analytics Powers AI Marketing
Predictive analytics breathes life into AI marketing by distilling vast quantities of data into crystal-clear insights, allowing marketers to anticipate user needs and refine their campaigns.
By employing sophisticated tools like IBM Watson Studio or TIBCO Data Science, marketing professionals can parse through social media chatter, magazine mentions, and online behavior to deliver personalized marketing that resonates with the user experience.
Through AI, organizations can balance the scales of supply and demand, ensuring their inventory meets the upcoming wave of consumer interest with precision.
Unveiling the Benefits of Predictive Analytics Tools
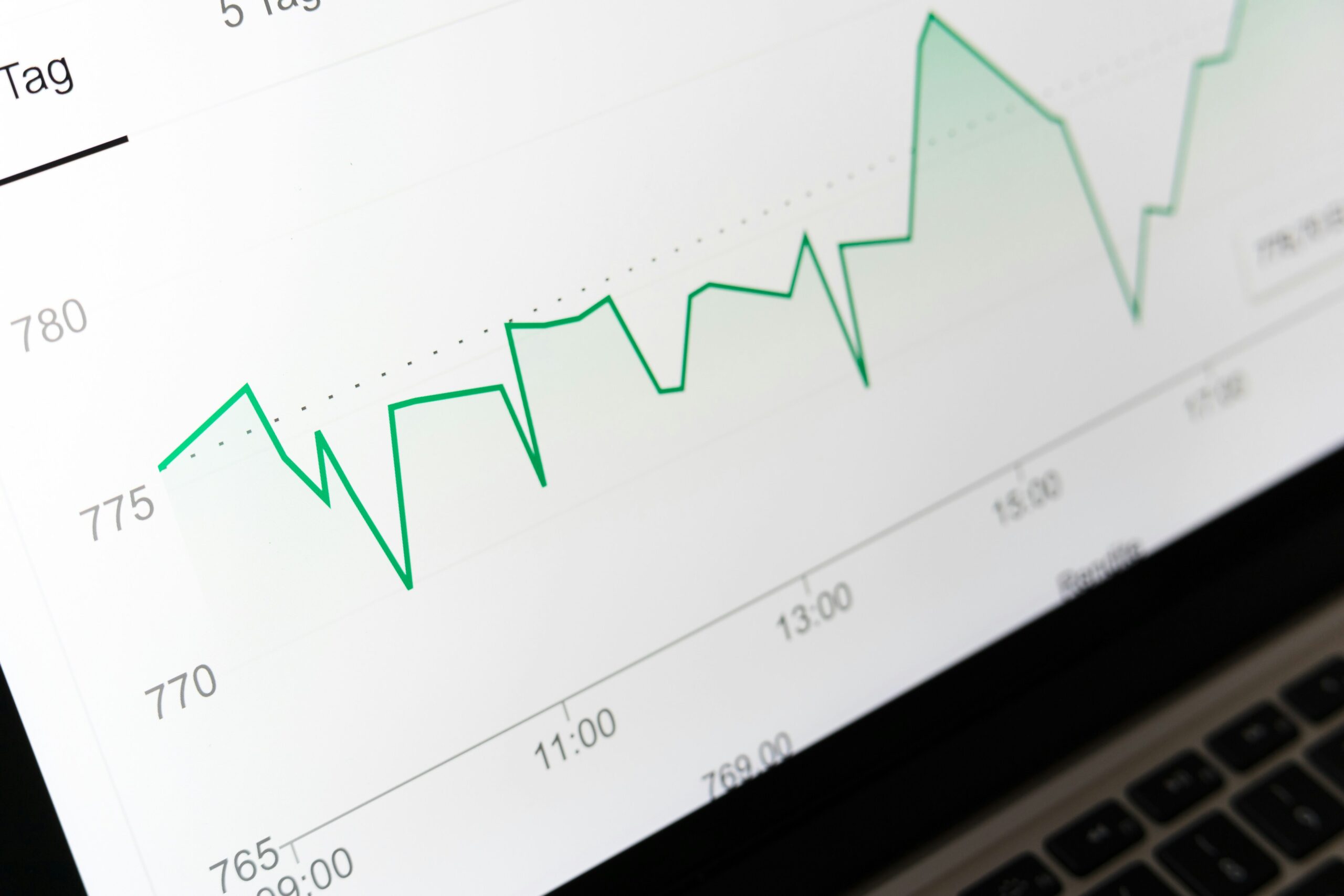
In the realm of AI marketing, predictive analytics tools stand as a cornerstone, equipping businesses with the power to make data-driven decisions that amplify efficiency.
These tools not only provide a competitive edge but also refine customer engagement with actionable insights and fine-tune marketing campaigns for an improved return on investment.
As companies deploy these advanced resources, they transition from a reactive to a proactive strategy, leveraging data analysis and predictive models to elevate their marketing landscape to new heights of effectiveness.
Boosting Efficiency With Data-Driven Decisions
Employing predictive analytics tools propels businesses toward a new echelon of efficiency, where careful analysis of consumer data translates into strategic actions that trim waste and bolster productivity.
Marketers gain the agility to respond nimbly to emerging trends, ensuring that resources are allocated to campaigns and initiatives that promise the most substantial impact on the market.
This precision in targeting, driven by insights gleaned from big data, optimizes both marketing endeavors and resource utilization within the complex supply and demand dynamics.
Enhancing Customer Engagement Through Insights
By tapping into predictive analytics, companies gain a profound understanding of customer behavior, allowing them to engage with their audience on a more personal level. This targeted approach not only captivates users but also fosters loyalty and significantly enhances the quality and relevance of every interaction, culminating in heightened customer satisfaction and stronger brand connections.
Streamlining Marketing Efforts for Better ROI
Streamlining marketing efforts with predictive analytics translates directly into amplified return on investment. Marketers can dissect and leverage data, devising campaigns that align closely with user preferences and trends, thus maximizing impact and reducing spend on poorly performing strategies.
- Identify high-yield marketing tactics through data-backed discernment.
- Allocate budget to impactful campaigns with precision, avoiding financial loss on ineffective methods.
- Measure campaign results against predictive benchmarks to continually refine and enhance marketing efficacy.
Selecting the Right Predictive Analytics Tools for Your Business
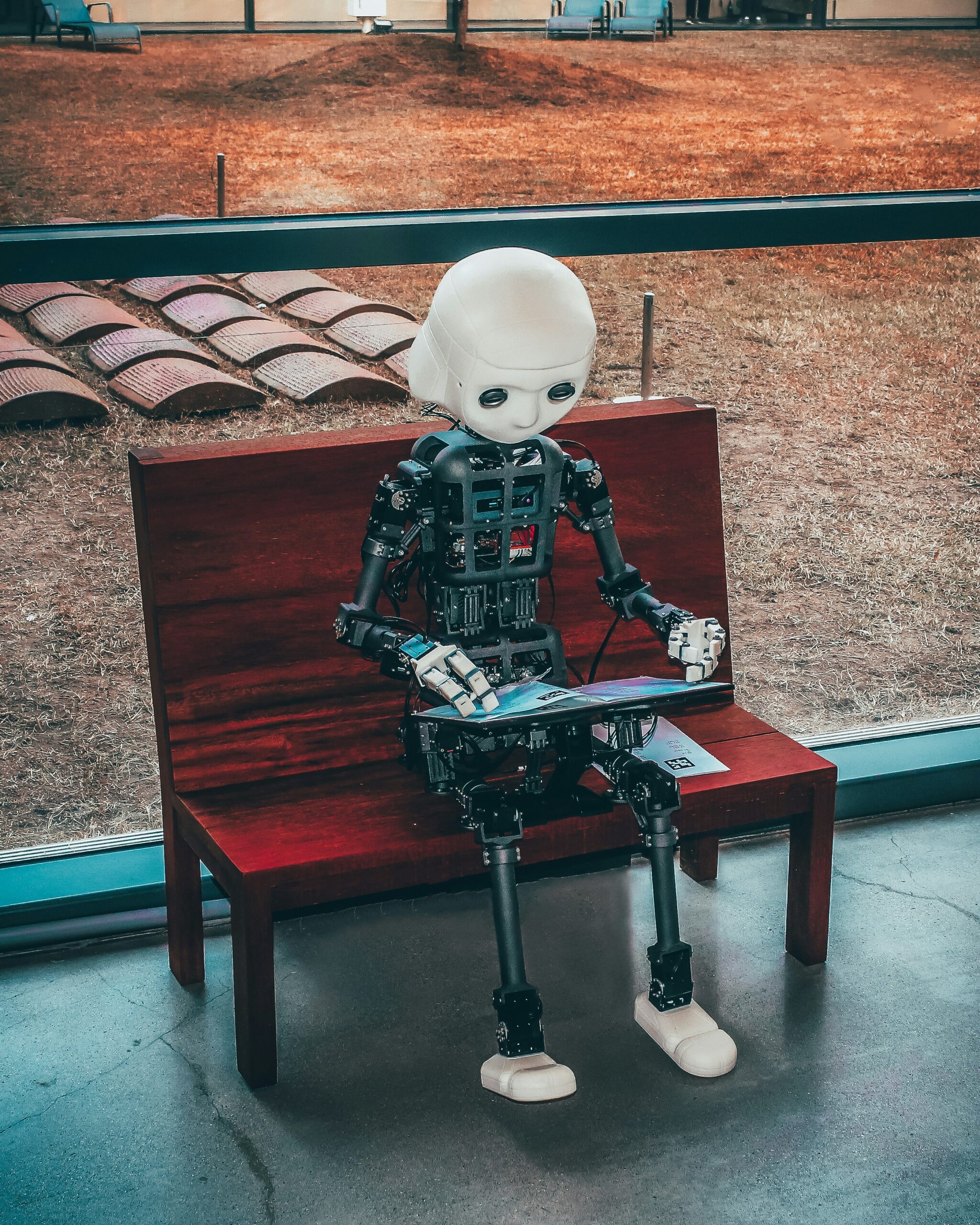
Embarking on the path of AI marketing requires a tool that not only aligns with an organization’s aspirations but also integrates seamlessly into its existing model.
Companies must look into the capabilities and functionalities of various leading predictive analytics tools.
This phase involves assessing which tools are best suited to fulfill specific marketing objectives, and how well they mesh with the current infrastructure to foster a symbiotic relationship between data science and marketing prowess.
Choosing the optimal tool is a pivotal step that can propel businesses toward realizing a more efficient, targeted, and results-driven marketing strategy.
Assessing the Features of Top Tools
When dissecting the array of predictive analytics tools on offer, an organization must weigh each system’s capacity for data preparation, integration, and vis––be it through user-friendly dashboards like Microsoft Power BI or comprehensive ecosystems like IBM Watson. Deliberate consideration should be given to how these tools tailor prediction algorithms to specific business needs, enhancing marketing precision and streamlining workflows for a transformative impact on the organization’s marketing intelligence.
Matching Tools to Marketing Goals
Identifying the optimal predictive analytics tools hinges on their alignment with specific marketing objectives, ensuring that features such as campaign simulation, consumer sentiment analysis, and behavioral forecasting are in concert with the desired outcomes. Marketers must ascertain that the chosen system not only integrates with their existing stack but also provides actionable insights to finely tune their marketing tactics towards the identified target audience.
Considering Ease of Integration With Existing Systems
Embarking on harnessing predictive analytics in AI marketing demands a tool that not only offers a treasure trove of features but one that can meld into an existing system without friction.
The ease with which these tools can be adopted, interacting smoothly with data warehouses, CRMs, and other operational technologies, determines the speed and success of their implementation, thereby affecting the marketing strategy’s agility and responsiveness.
Integrating Predictive Analytics Into AI Marketing Strategies
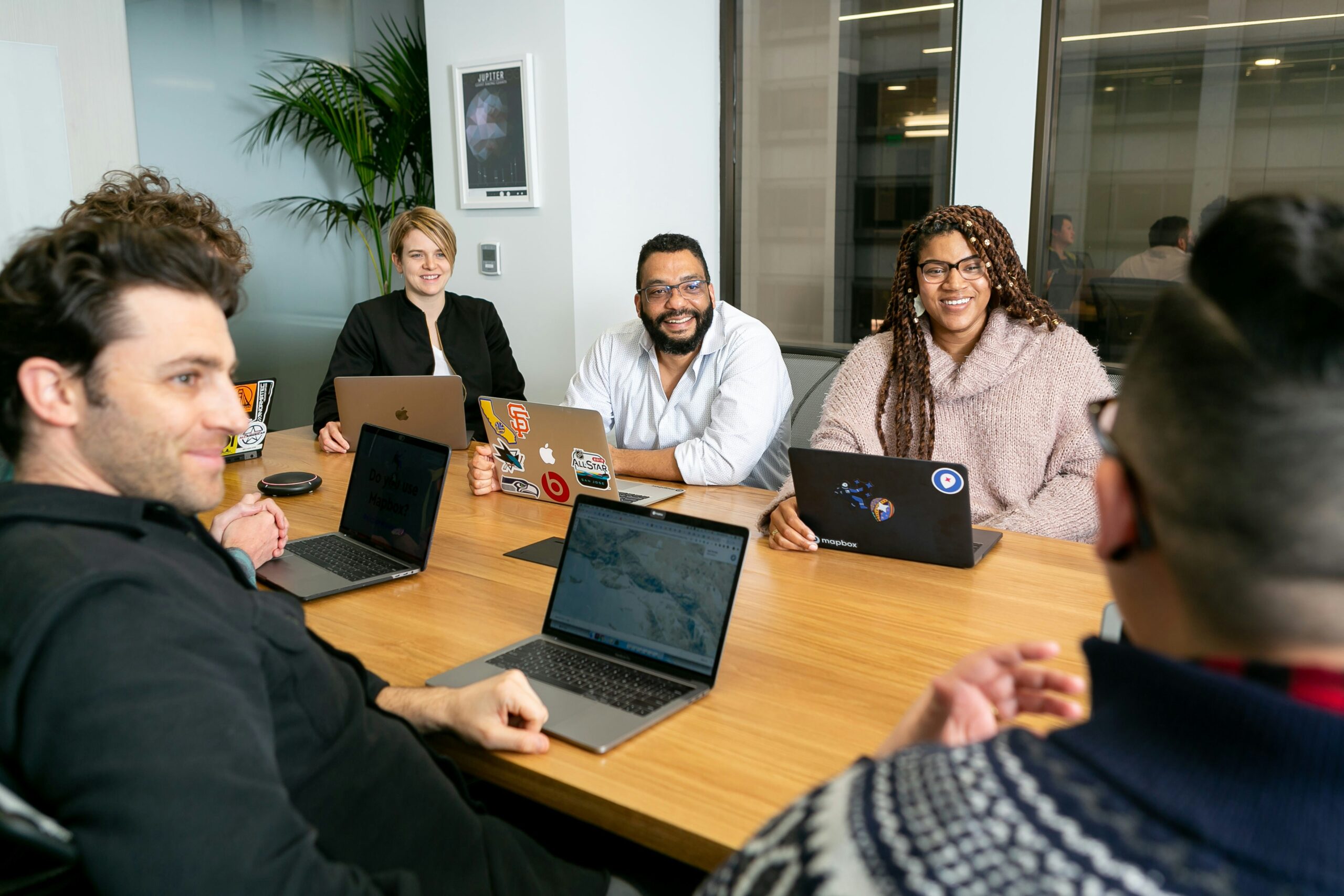
Within the dynamic landscape of AI marketing, the integration of predictive analytics is a critical step for organizations aiming to stay at the forefront of consumer engagement and market relevance.
It’s not merely about introducing new tools into the existing suite; it involves a strategic rethinking of operations from the ground up.
Setting well-defined targets for data utilization, crafting a streamlined process to transform insights into actions, and equipping teams with the skills to harness the full potential of predictive insights are all essential components of building a robust AI marketing strategy.
Each element contributes to a larger synergy that transforms raw numbers into a narrative that guides business growth and innovation.
Defining Clear Objectives for Data Use
Setting clear objectives for data use within AI marketing is paramount, ensuring predictive analytics tools are directed to not only gather relevant data but also to yield insights that are applicable and valuable. It’s about defining the data that will feed the algorithm, which, in turn, empowers the predictive system to deliver forecasts that align with marketing goals and drive actionable strategies that resonate with the target audience.
Establishing a Workflow for Analytics Insights
Establishing a workflow for analytics insights is a transformative step toward embedding predictive capabilities into marketing operations. It involves creating a systematic approach that begins with the collection and tracking of data, followed by data analysis and interpretation to conceive targeted marketing strategies.
This must be an iterative process, allowing for continuous refinement as new patterns emerge, ensuring that the organization remains adaptive and strategies stay aligned with evolving customer behaviors and market conditions.
Training Teams to Leverage Predictive Insights
Training teams to leverage predictive insights is crucial in today’s dynamic business environment. As organizations amass vast amounts of data, the ability to extract meaningful predictions from that data becomes a strategic advantage. This training equips teams with the necessary skills to analyze historical data, identify patterns, and use advanced analytics tools to generate predictive insights.
By understanding trends and anticipating future developments, teams can make informed decisions, optimize processes, and proactively address challenges. Moreover, the training empowers teams to embrace a forward-thinking approach, fostering a culture of innovation and adaptability.
Best Practices for Maximizing Predictive Analytics in Marketing
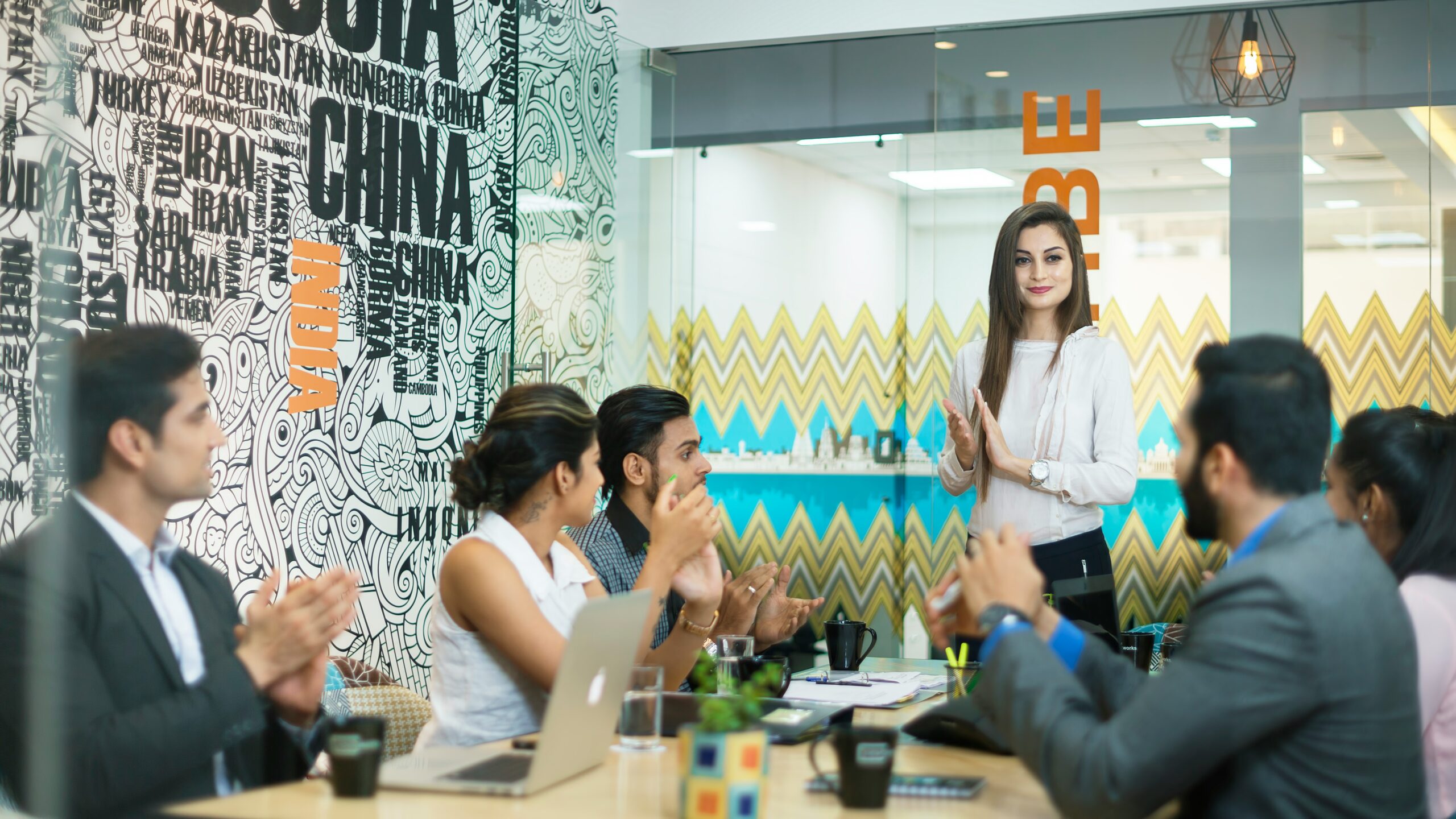
To effectively navigate the intersection of AI marketing and predictive analytics, organizations must aspire to perfect the art of synergy between advanced technological tools and strategic human insight.
This delicate balance calls for continuous attention to data quality, meticulous tuning of automation processes, and a vigilant eye on the fast-paced advancements in artificial intelligence.
By embracing these pillars—meticulous data quality management, astutely marrying automation with expert human judgment, and remaining agile in the face of technological change—businesses can ensure their predictive analytics efforts yield the utmost efficiency and campaign success.
Continuous Data Quality Management
Continuous Data Quality Management is a pivotal practice in ensuring the accuracy and reliability of data across an organization’s various datasets. It involves ongoing efforts to assess, cleanse, and enrich data, creating a foundation of trust for decision-making processes and customer interactions.
By establishing dynamic improvement cycles and incorporating feedback loops, businesses can swiftly identify and rectify discrepancies, preventing the proliferation of inaccuracies.
The business impact is substantial, enabling organizations to make informed decisions, engage in targeted marketing, and exhibit agility in adapting to evolving market conditions and regulatory requirements.
Objective | Tools & Techniques | Outcome | |
---|---|---|---|
Data Collection | Gather clean, comprehensive datasets | CRM, Web forms, IoT devices | High-quality input for analysis |
Data Cleaning | Remove errors and inconsistencies | Data scrubbing software, Manual review | Streamlined, usable data |
Data Verification | Confirm data accuracy and completeness | Cross-referencing tools, Validation algorithms | Trustworthy data for decision-making |
Continuous Monitoring | Ensure ongoing data integrity | Automated alerts, Regular audits | Sustained quality control over time |
Balancing Automation With Human Oversight
The synchrony between automation and human insight is essential in predictive analytics, allowing organizations to tap into the efficiency of machine learning while maintaining the creativity and critical thinking inherent in the human brain.
By installing checks and balances where automated systems are reviewed by skilled analysts, businesses strike a balance that maximizes both the speed of AI and the nuance of human oversight, thereby driving smarter marketing strategies that adapt as the landscape changes.
Action | Role of Automation | Role of Human Oversight | Combined Benefit |
---|---|---|---|
Data Analysis | Process large volumes quickly with AI algorithms | Apply context and strategic thinking to findings | Rich, actionable insights with strategic foresight |
Campaign Adjustment | Implement changes across platforms efficiently | Review performance and tweak for human relevance | Optimized campaigns that resonate with audiences |
Performance Tracking | Monitor key metrics continuously in real-time | Interpret data beyond numbers to guide decisions | Dynamic strategy pivots based on nuanced analysis |
Customer Interaction | Manage basic queries and interactions 24/7 | Handle complex issues requiring empathy and understanding | Seamless customer service with a personal touch |
Keeping Up With the Evolving AI Landscape
Adapting to the rapidly advancing field of AI marketing is not merely about keeping pace with technology; it’s about continually nurturing a culture of learning and innovation.
Marketers and data scientists must stay informed about the latest developments in AI, such as deep learning and natural language processing, to sustain a competitive advantage and improve predictive marketing analytics continually.
- Deep learning enhances pattern recognition, allowing for more nuanced customer behavior forecasting.
- Natural language processing betters the understanding of user sentiment and conversation nuances across digital platforms.
- Frequent training sessions for marketing and analytics teams to stay adept with emerging AI tools and applications.
Navigating the Ethical Considerations of AI and Predictive Analytics
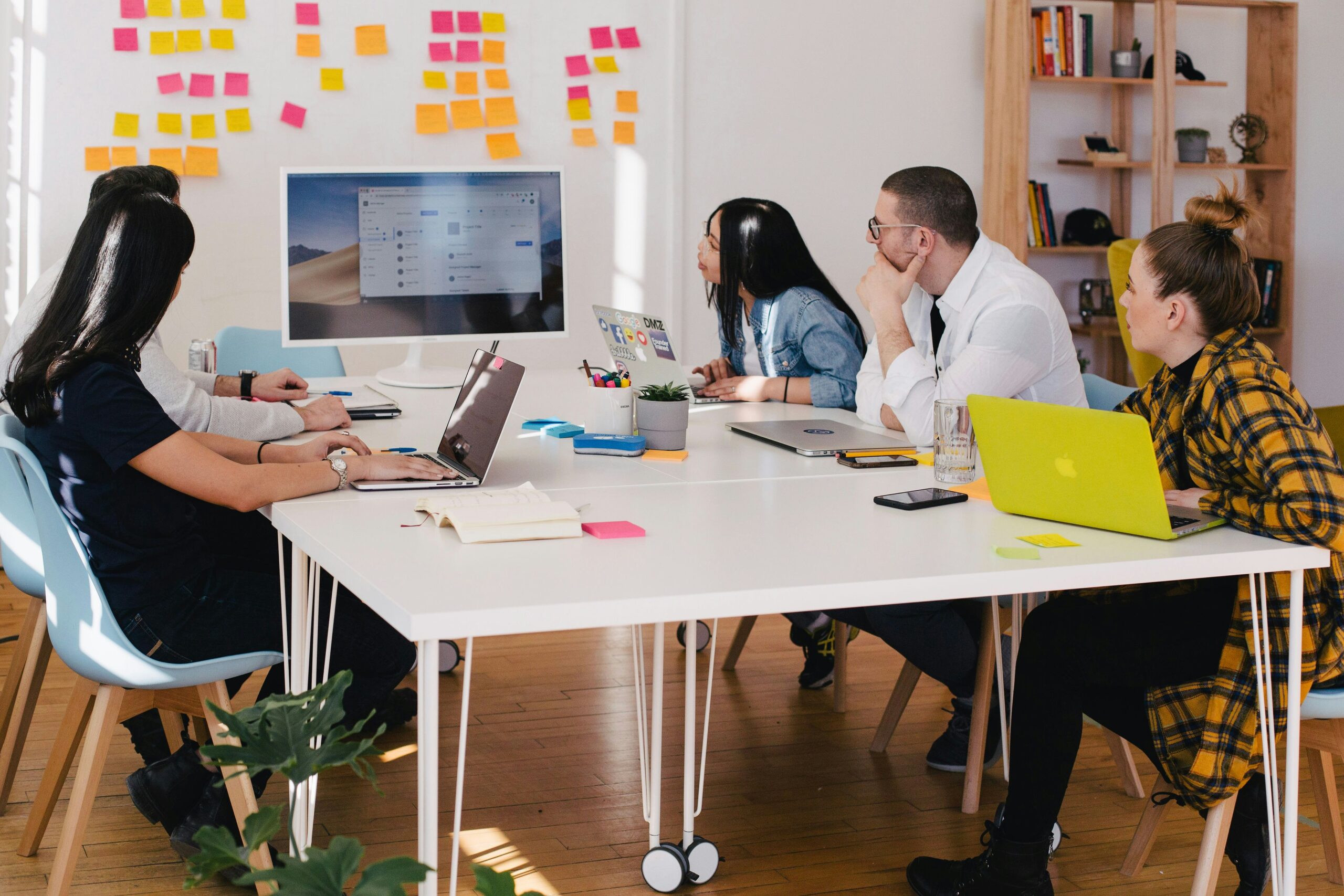
Navigating the delicate balance between leveraging consumer data for insights and upholding individual privacy has become a concern.
The pursuit of unbiased, equitable data and algorithms stands as another pillar of ethical AI marketing, ensuring that the systems powering predictive models do not perpetuate existing disparities or introduce new ones.
Additionally, forging a bond of trust with customers hinges on maintaining transparent marketing practices that clarify how data is used to shape their user experience.
This ongoing dialogue shapes the ethical framework within which AI marketing operates, guarding against the risks while maximizing the transformative potential of data-driven insights.
Privacy and Data Protection Challenges
As organizations leverage consumer data to fuel predictive analytics, they face the challenge of safeguarding user privacy and securing sensitive information against potential breaches.
Balancing the benefits of personalization with the imperative of privacy protection requires diligent adherence to data protection regulations and transparent communication with users about how their data is utilized and protected.
Concern | Impact | Preventive Measures |
---|---|---|
User Privacy | Potential erosion of consumer trust | Strict compliance with privacy laws, clear user consent protocols |
Data Security | Risk of unauthorized access and data breaches | Robust encryption, regular security audits, and breach response planning |
Ethical Data Usage | Damage to brand reputation from misuse of data | Transparent policies, ethical guidelines for data handling |
Addressing Potential Biases in Data and Algorithms
Confronting biases in data and algorithms is a critical step toward ethical AI marketing, as unchecked preconceptions can skew predictive analytics and yield misleading outcomes. Marketers and data scientists must continuously scrutinize their data sets and refine their algorithms for predictive analysis, ensuring that their marketing strategies are rooted in objective, equitable insights.
By committing to this level of vigilance, organizations fortify their AI initiatives against bias, enhancing the integrity and inclusiveness of their marketing efforts.
Maintaining Transparency in AI Marketing Practices
In an era where AI-driven marketing is surging ahead, the call for transparency becomes critical. Companies strive to demystify their use of predictive analytics, aiming to openly communicate how customer data informs targeted campaigns and personalized experiences.
This practice not only builds consumer trust but also fortifies a brand’s credibility and fosters a culture of accountability in a data-centric marketplace.
Conclusion
In conclusion, mastering AI marketing through the utilization of predictive analytics tools grants businesses a formidable edge in crafting sophisticated strategies that resonate deeply with consumer trends and behaviors.
These advanced tools empower companies to pivot from reactive tactics to proactive, data-driven approaches that substantially enhance efficiency, customer engagement, and ROI.
By managing data quality, striking a balance between automation and human intuition, and continuously evolving with AI advancements, organizations can unlock powerful insights for more targeted and effective marketing campaigns.
However, navigating this high-tech terrain requires a steadfast commitment to ethical considerations, including privacy protection, bias mitigation, and transparency to maintain consumer trust.
In summary, those who adeptly harness the predictive capabilities of AI emerge as leaders in the marketing landscape, ready to meet future challenges with confidence and strategic insight.
Comments